Multi-attribute spaces: Calibration for attribute fusion and similarity search
CVPR(2012)
摘要
Recent work has shown that visual attributes are a powerful approach for applications such as recognition, image description and retrieval. However, fusing multiple attribute scores — as required during multi-attribute queries or similarity searches — presents a significant challenge. Scores from different attribute classifiers cannot be combined in a simple way; the same score for different attributes can mean different things. In this work, we show how to construct normalized “multi-attribute spaces” from raw classifier outputs, using techniques based on the statistical Extreme Value Theory. Our method calibrates each raw score to a probability that the given attribute is present in the image. We describe how these probabilities can be fused in a simple way to perform more accurate multiattribute searches, as well as enable attribute-based similarity searches. A significant advantage of our approach is that the normalization is done after-the-fact, requiring neither modification to the attribute classification system nor ground truth attribute annotations. We demonstrate results on a large data set of nearly 2 million face images and show significant improvements over prior work. We also show that perceptual similarity of search results increases by using contextual attributes.
更多查看译文
关键词
fusing multiple attribute score,attribute classification system,attribute-based similarity search,perceptual similarity,contextual attribute,attribute fusion,different thing,Multi-attribute space,prior work,visual attribute,ground truth attribute annotation,different attribute
AI 理解论文
溯源树
样例
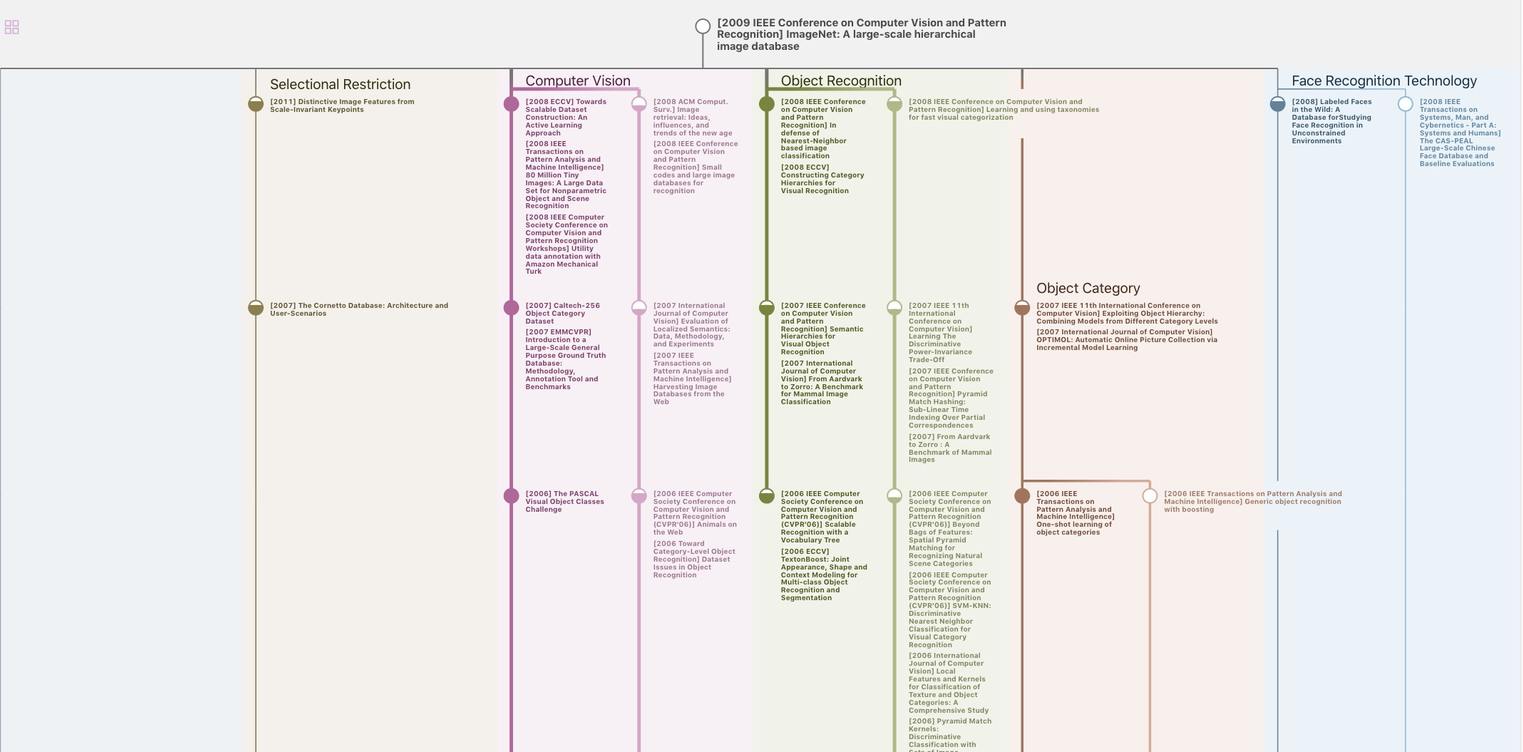
生成溯源树,研究论文发展脉络
Chat Paper
正在生成论文摘要