Multi-Task Drug Bioactivity Classification With Graph Labeling Ensembles
PRIB'11: Proceedings of the 6th IAPR international conference on Pattern recognition in bioinformatics(2011)
摘要
We present a new method for drug bioactivity classification based on learning an ensemble of multi-task classifiers. As the base classifiers of the ensemble we use Max-Margin Conditional Random Field (MMCRF) models, which have previously obtained the state-of-the-art accuracy in this problem. MMCRF relies on a graph structure coupling the set of tasks together, and thus turns the multi-task learning problem into a graph labeling problem. In our ensemble method the graphs of the base classifiers are random, constructed by random pairing or random spanning tree extraction over the set of tasks.We compare the ensemble approaches on datasets containing the cancer inhibition potential of drug-like molecules against 60 cancer cell lines. In our experiments we find that ensembles based on random graphs surpass the accuracy of single SVM as well as a single MMCRF model relying on a graph built from auxiliary data.
更多查看译文
关键词
drug bioactivity prediction,multi-task learning,ensemble methods,kernel methods
AI 理解论文
溯源树
样例
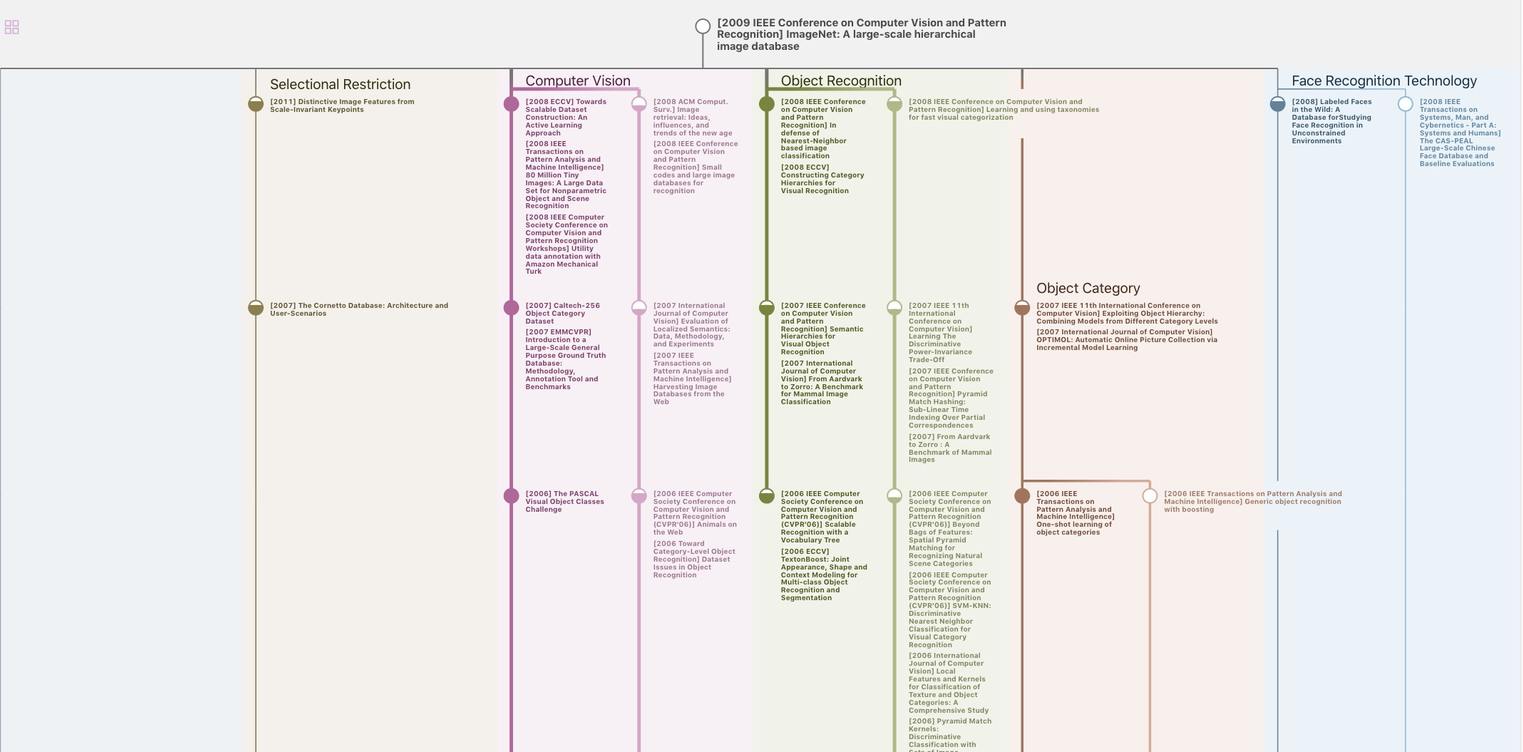
生成溯源树,研究论文发展脉络
Chat Paper
正在生成论文摘要