Learning-based Multi-Sieve Co-reference Resolution with Knowledge.
EMNLP-CoNLL '12: Proceedings of the 2012 Joint Conference on Empirical Methods in Natural Language Processing and Computational Natural Language Learning(2012)
摘要
We explore the interplay of knowledge and structure in co-reference resolution. To inject knowledge, we use a state-of-the-art system which cross-links (or "grounds") expressions in free text to Wikipedia. We explore ways of using the resulting grounding to boost the performance of a state-of-the-art co-reference resolution system. To maximize the utility of the injected knowledge, we deploy a learning-based multi-sieve approach and develop novel entity-based features. Our end system outperforms the state-of-the-art baseline by 2 B 3 F1 points on non-transcript portion of the ACE 2004 dataset.
更多查看译文
关键词
end system,state-of-the-art baseline,state-of-the-art co-reference resolution system,state-of-the-art system,co-reference resolution,B3 F1 point,free text,learning-based multi-sieve approach,non-transcript portion,novel entity-based feature,Learning-based multi-sieve co-reference resolution
AI 理解论文
溯源树
样例
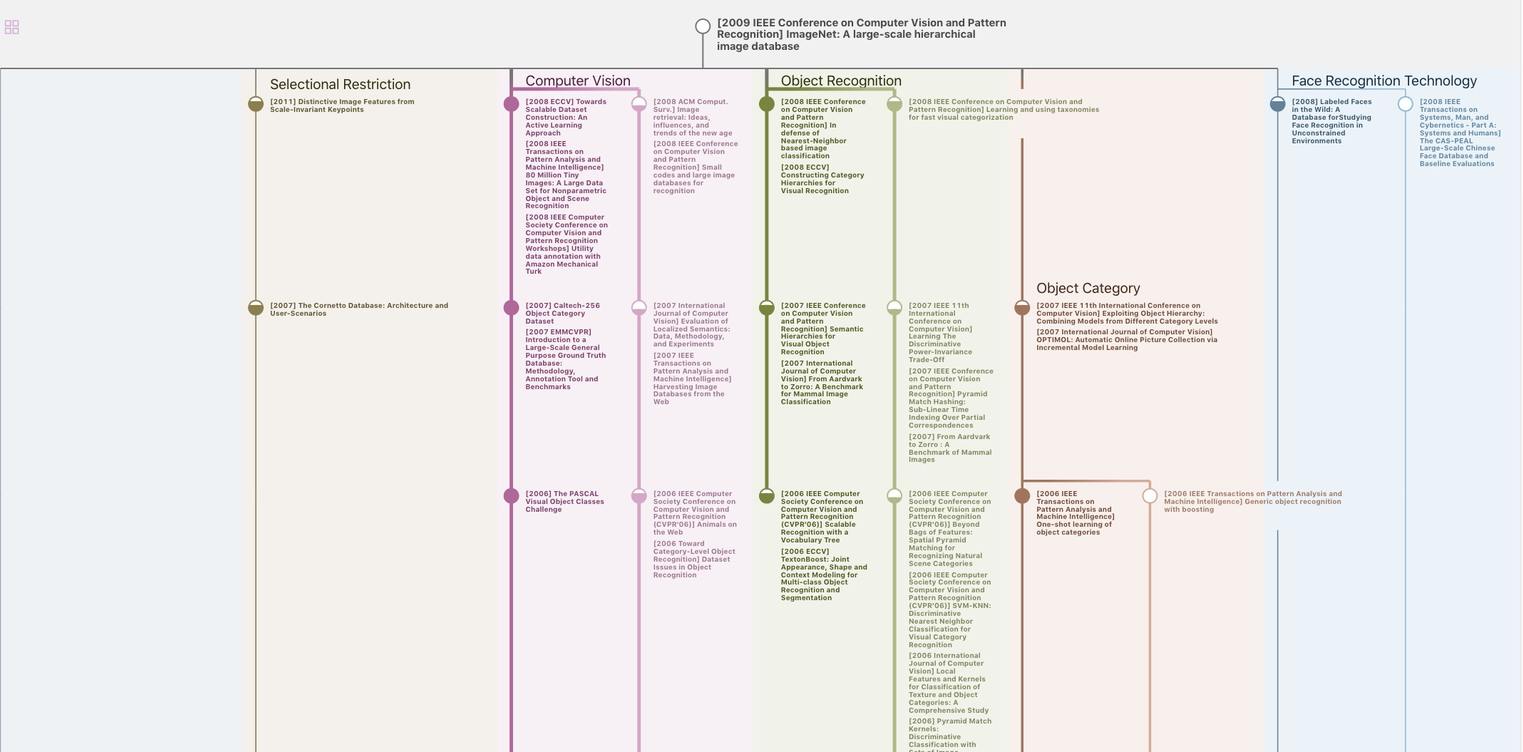
生成溯源树,研究论文发展脉络
Chat Paper
正在生成论文摘要