Blind Matrix Decomposition Via Genetic Optimization Of Sparseness And Nonnegativity Constraints
ICANN'07: Proceedings of the 17th international conference on Artificial neural networks(2007)
摘要
Nonnegative Matrix Factorization (NMF) has proven to be a useful tool for the analysis of nonnegative multivariate data. However, it is known not to lead, to unique results when applied to nonnegative Blind Source Separation (BSS) problems. In this paper we present first results of an extension to the NMF algorithm which solves the BSS problem when the underlying sources are sufficiently sparse. As the proposed target function is discontinuous and possesses many local minima, we use a genetic algorithm for its minimization. Application to a microarray data set will be considered also.
更多查看译文
关键词
BSS problem,NMF algorithm,genetic algorithm,microarray data,nonnegative multivariate data,Blind Source,Nonnegative Matrix Factorization,local minimum,proposed target function,underlying source,blind matrix decomposition,genetic optimization,nonnegativity constraint
AI 理解论文
溯源树
样例
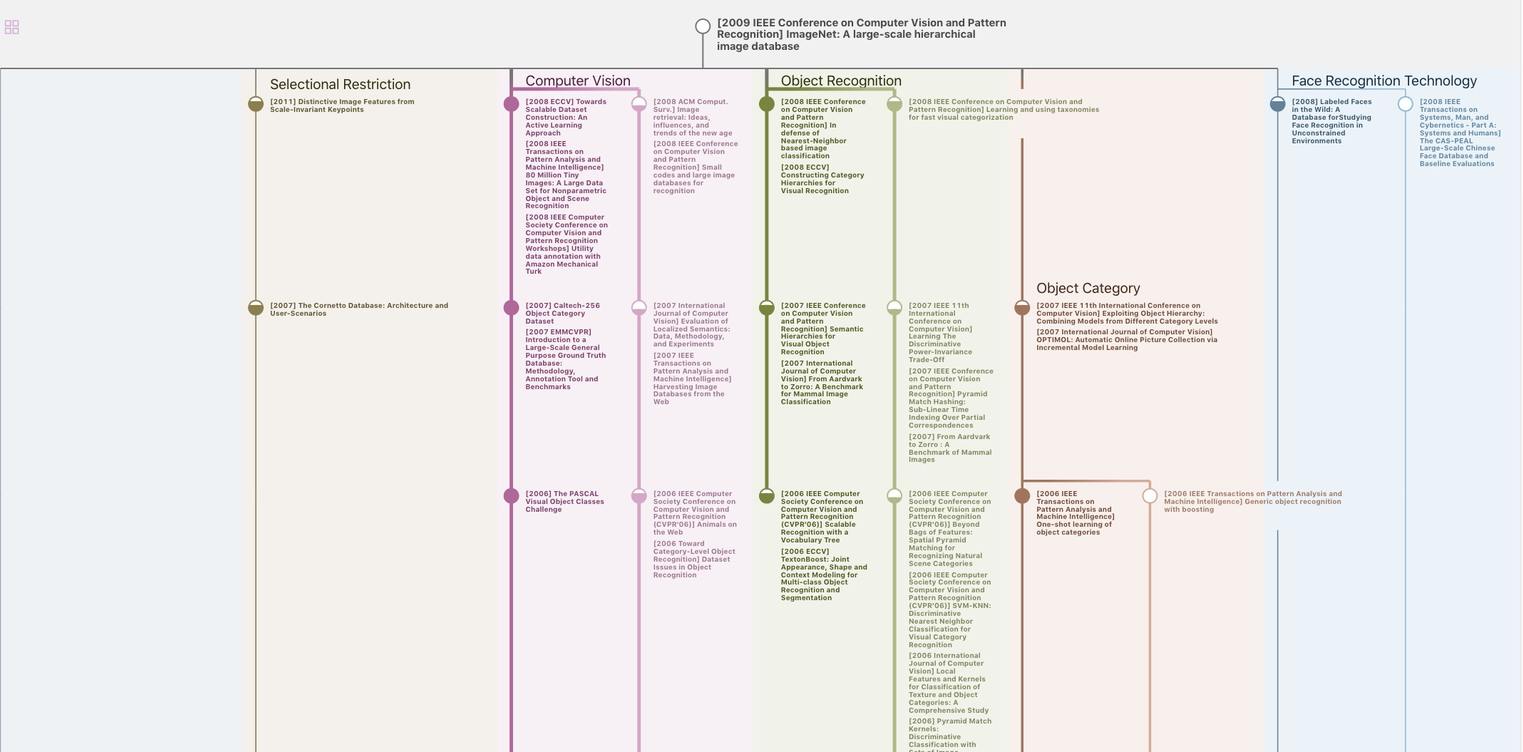
生成溯源树,研究论文发展脉络
Chat Paper
正在生成论文摘要