SVD Postmapping for Nonlinear Dimensionality Reduction
msra
摘要
Dimensionality reduction is a key technique in machine learning and pattern recognition when dealing with complicated real world applications. While many techniques have been developed to address this problem and widely applied in practice, there is relatively less effort in studying extension of the dimensionality reduction to new data points. In this paper, we propose a novel approach to extend the dimensionality reduction to new input data and illustrate its use in real applications with several dimensionality reduction methods. Our method does not require any prior knowledge of the dimensionality reduction algorithm and can be used with all nonlinear dimensionality reduction algorithms that preserve local isometry. The experimental results show the properties of the proposed algorithm and its potential for applications in classification and pattern analysis.
更多查看译文
AI 理解论文
溯源树
样例
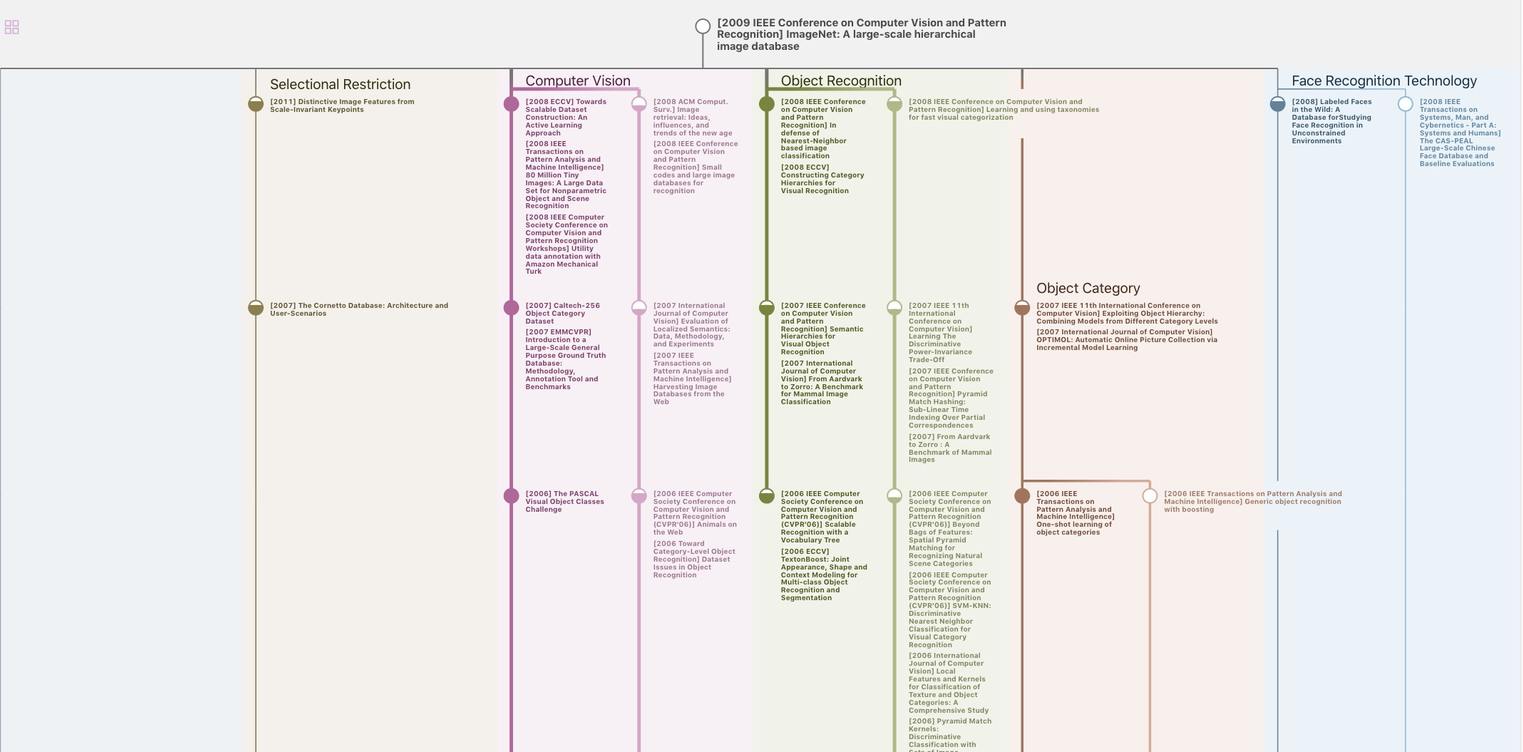
生成溯源树,研究论文发展脉络
Chat Paper
正在生成论文摘要