Fingerprinting Codes and the Price of Approximate Differential Privacy
STOC(2015)
摘要
We show new lower bounds on the sample complexity of $(\varepsilon, \delta)$-differentially private algorithms that accurately answer large sets of counting queries. A counting query on a database $D \in (\{0,1\}^d)^n$ has the form "What fraction of the individual records in the database satisfy the property $q$?" We show that in order to answer an arbitrary set $\mathcal{Q}$ of $\gg nd$ counting queries on $D$ to within error $\pm \alpha$ it is necessary that $$ n \geq \tilde{\Omega}\Bigg(\frac{\sqrt{d} \log |\mathcal{Q}|}{\alpha^2 \varepsilon} \Bigg). $$ This bound is optimal up to poly-logarithmic factors, as demonstrated by the Private Multiplicative Weights algorithm (Hardt and Rothblum, FOCS'10). In particular, our lower bound is the first to show that the sample complexity required for accuracy and $(\varepsilon, \delta)$-differential privacy is asymptotically larger than what is required merely for accuracy, which is $O(\log |\mathcal{Q}| / \alpha^2)$. In addition, we show that our lower bound holds for the specific case of $k$-way marginal queries (where $|\mathcal{Q}| = 2^k \binom{d}{k}$) when $\alpha$ is not too small compared to $d$ (e.g. when $\alpha$ is any fixed constant). Our results rely on the existence of short \emph{fingerprinting codes} (Boneh and Shaw, CRYPTO'95, Tardos, STOC'03), which we show are closely connected to the sample complexity of differentially private data release. We also give a new method for combining certain types of sample complexity lower bounds into stronger lower bounds.
更多查看译文
关键词
differential privacy,fingerprinting codes
AI 理解论文
溯源树
样例
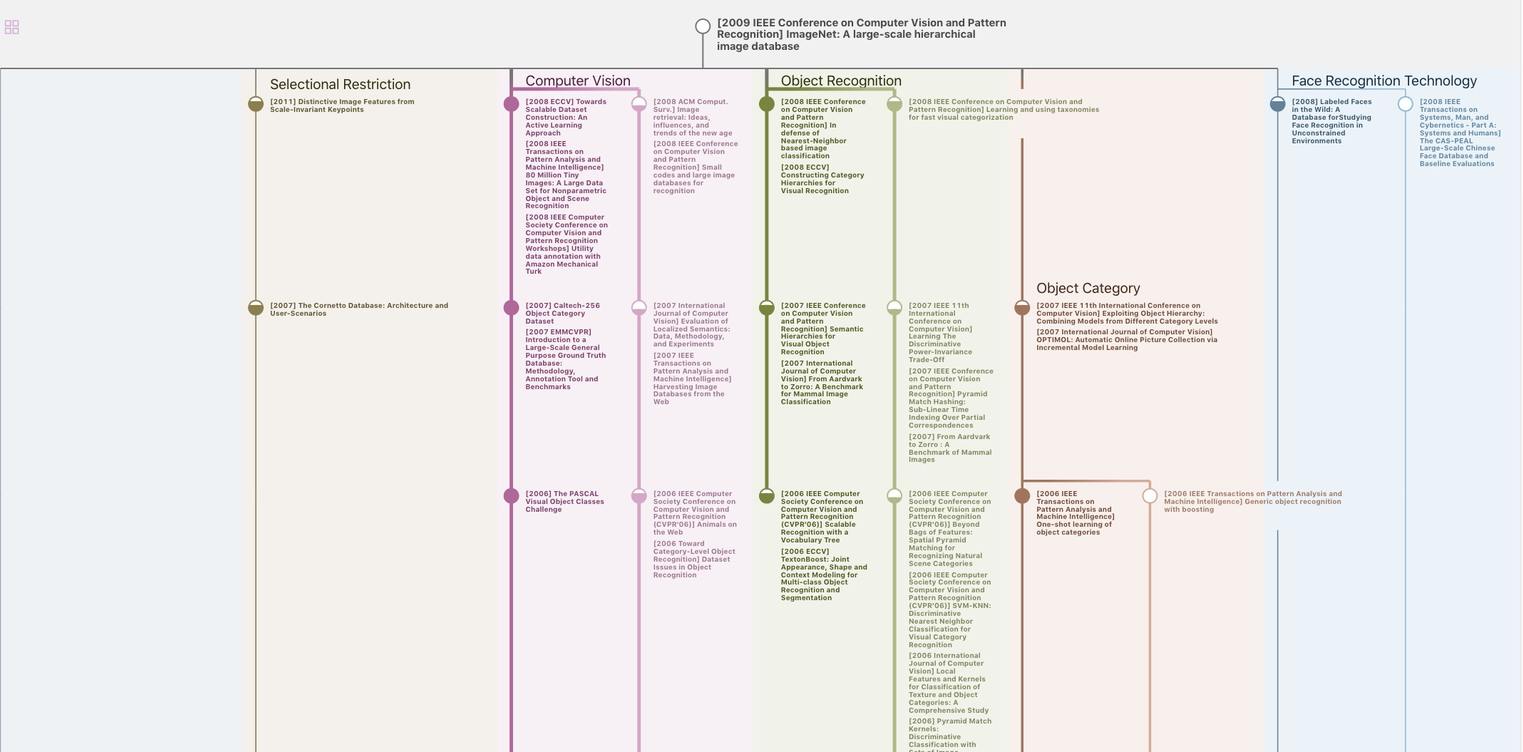
生成溯源树,研究论文发展脉络
Chat Paper
正在生成论文摘要