A Unified Formulation Of Gaussian Versus Sparse Stochastic Processes-Part Ii: Discrete-Domain Theory
IEEE TRANSACTIONS ON INFORMATION THEORY(2014)
摘要
This paper is devoted to the characterization of an extended family of continuous-time autoregressive moving average (CARMA) processes that are solutions of stochastic differential equations driven by white Levy innovations. These are completely specified by: 1) a set of poles and zeros that fixes their correlation structure and 2) a canonical infinitely divisible probability distribution that controls their degree of sparsity (with the Gaussian model corresponding to the least sparse scenario). The generalized CARMA processes are either stationary or nonstationary, depending on the location of the poles in the complex plane. The most basic nonstationary representatives (with a single pole at the origin) are the Levy processes, which are the non-Gaussian counterparts of Brownian motion. We focus on the general analog-to-discrete conversion problem and introduce a novel spline-based formalism that greatly simplifies the derivation of the correlation properties and joint probability distributions of the discrete versions of these processes. We also rely on the concept of generalized increment process, which suppresses all long range dependencies, to specify an equivalent discrete-domain innovation model. A crucial ingredient is the existence of a minimally supported function associated with the whitening operator L; this B-spline, which is fundamental to our formulation, appears in most of our formulas, both at the level of the correlation and the characteristic function. We make use of these discrete-domain results to numerically generate illustrative examples of sparse signals that are consistent with the continuous-domain model.
更多查看译文
关键词
Sparsity, non-Gaussian stochastic processes, innovation modeling, continuous-time signals, stochastic differential equations, sampling, exponential B-splines, Levy process, CARMA processes, infinite divisibility
AI 理解论文
溯源树
样例
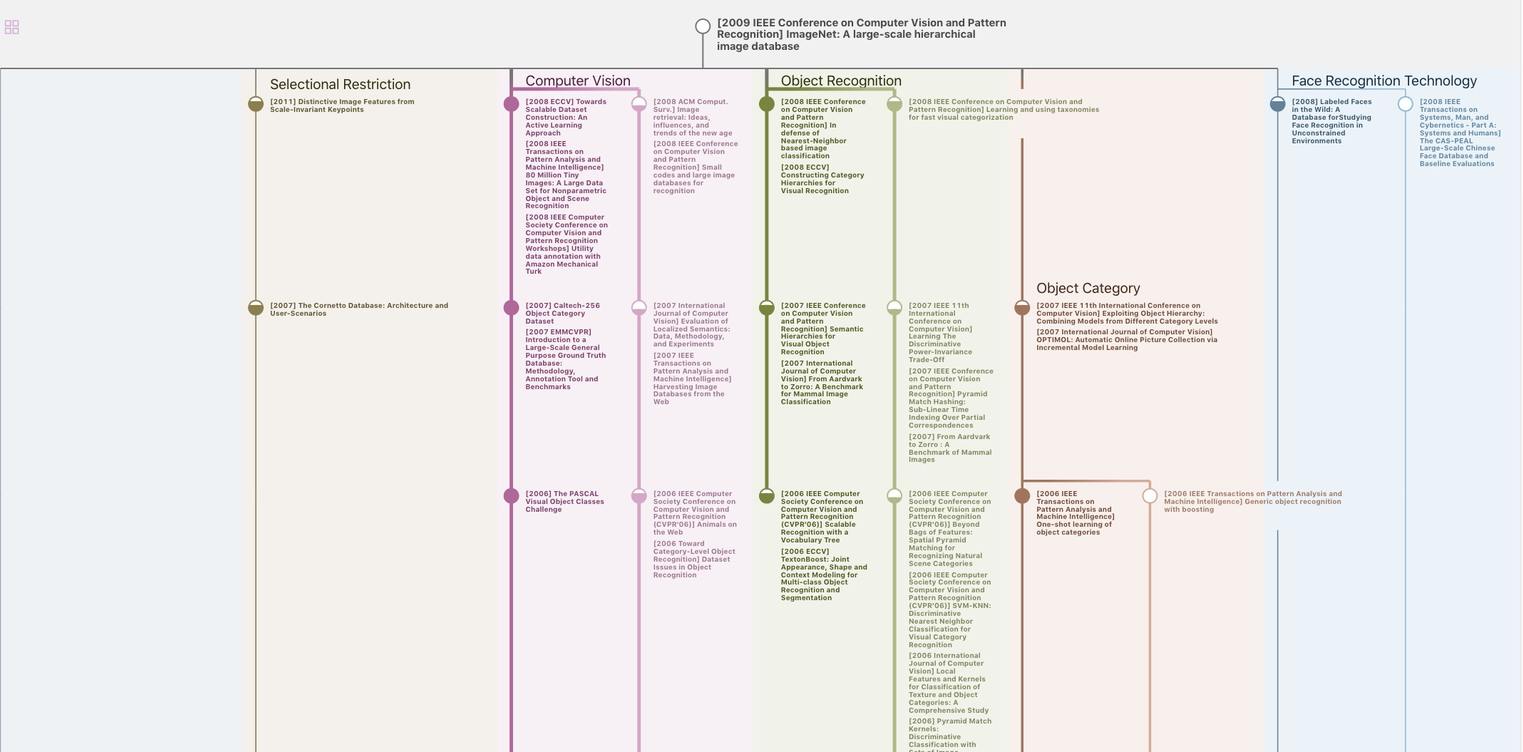
生成溯源树,研究论文发展脉络
Chat Paper
正在生成论文摘要