Time-Series Classification in Many Intrinsic Dimensions
SDM(2010)
摘要
In the context of many data mining tasks, high dimensional- ity was shown to be able to pose significant problems, com- monly referred to as different aspects of the curse of dimen- sionality. In this paper, we investigate in the time-series do- main one aspect of the dimensionality curse called hubness, which refers to the tendency of some instances in a data set to become hubs by being included in unexpectedly many k-nearest neighbor lists of other instances. Through empir- ical measurements on a large collection of time-series data sets we demonstrate that the hubness phenomenon is caused by high intrinsic dimensionality of time-series data, and shed light on the mechanism through which hubs emerge, focusing on the popular and successful dynamic time warping (DTW) distance. Also, the interaction between hubness and the in- formation provided by class labels is investigated, by con- sidering label matches and mismatches between neighboring time series. Following our findings we formulate a framework for cat- egorizing time-series data sets based on measurements that reflect hubness and the diversity of class labels among near- est neighbors. The framework allows one to assess whether hubness can be successfully used to improve the performance of k-NN classification. Finally, the merits of the framework are demonstrated through experimental evaluation of 1-NN and k-NN classifiers, including a proposed weighting scheme that is designed to make use of hubness information. Our experimental results show that the examined framework, in the majority of cases, is able to correctly reflect the cir- cumstances in which hubness information can effectively be employed in k-NN time-series classification.
更多查看译文
关键词
time series data,time series,data mining,k nearest neighbor,intrinsic dimension,dynamic time warping
AI 理解论文
溯源树
样例
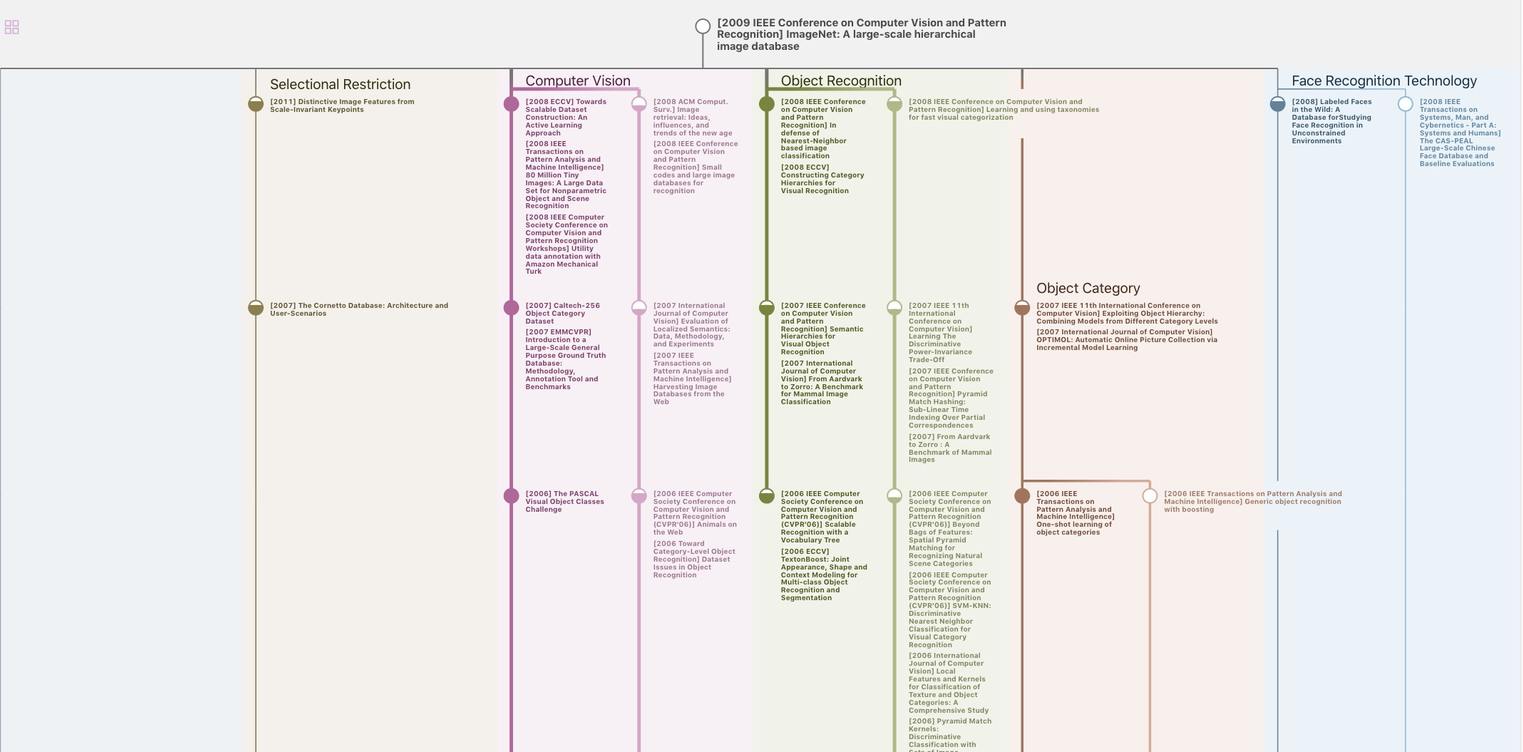
生成溯源树,研究论文发展脉络
Chat Paper
正在生成论文摘要