Temporal patterns of frequency-localized features in asr
Temporal patterns of frequency-localized features in asr(2003)
摘要
This work investigates the use of frequency-localized temporal patterns of the speech signal for developing robust front-end for Automatic Speech Recognition (ASR). Various linear transforms are investigated for parameterization of the frequency-localized temporal patterns. We show that temporal patterns closely follow the properties of a first-order Markov process, which results in the PCA transforms being very close to the DCT transform. Better recognition performance is achieved on using the DCT components of temporal patterns as opposed to directly using temporal patterns for feature estimation. Other linear transforms such as Linear Discriminant Analysis (LDA) are also studied for the parameterization. The parameterized TempoRA1 Patterns (TRAPS) are used to estimate broad-phonetic clans-posteriors independently in each critical-band. These class-posteriors are combined and used as the features for word recognition. Our work shows that broad-phonetic features generalize better than other conventional features and yield considerable complementary information with respect to short-term cepstral features in ASR. Two practical applications are proposed for the broad-phonetic TRAPS features: (1) Distributed Speech Recognition (DSR) in cellular telephony, (2) Voice Activity Detection (VAD) tanks. These features yield a significant improvement in the performance for these applications. New band-independent categories are proposed which represent distinct speech-events in the frequency-localized temporal patterns of the speech signal. These categories are obtained by clustering the mean temporal patterns of context-independent phones using an agglomerative hierarchical clustering technique. A Universal TempoRAl PatternS (UTRAPS) system is proposed for the speech-event class-posteriors estimation. Combining UTRAPS features with cepstral features achieves a significant improvement in the recognition performance under noisy conditions. Finally, this work studies the effect of broadening the frequency-context on TRAPS features and ASR. This study shows that combining temporal patterns from more than one critical-band is important to achieve higher recognition rates.
更多查看译文
关键词
higher recognition rate,frequency-localized temporal pattern,speech signal,frequency-localized feature,mean temporal pattern,word recognition,traps feature,recognition performance,significant improvement,temporal pattern,better recognition performance
AI 理解论文
溯源树
样例
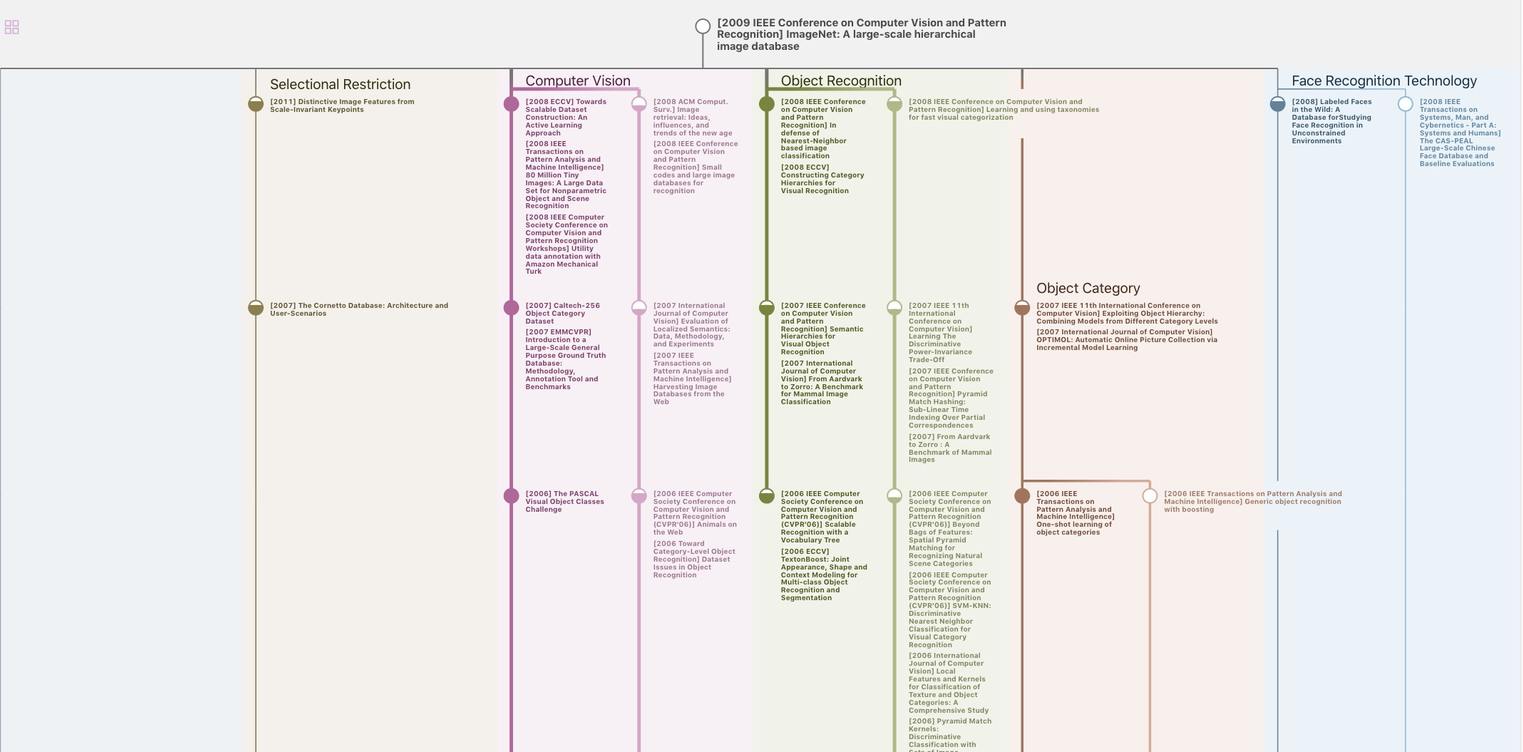
生成溯源树,研究论文发展脉络
Chat Paper
正在生成论文摘要