Large scale link based latent Dirichlet allocation for web document classification
Clinical Orthopaedics and Related Research(2010)
摘要
In this paper we demonstrate the applicability of latent Dirichlet allocation
(LDA) for classifying large Web document collections. One of our main results
is a novel influence model that gives a fully generative model of the document
content taking linkage into account. In our setup, topics propagate along links
in such a way that linked documents directly influence the words in the linking
document. As another main contribution we develop LDA specific boosting of
Gibbs samplers resulting in a significant speedup in our experiments. The
inferred LDA model can be applied for classification as dimensionality
reduction similarly to latent semantic indexing. In addition, the model yields
link weights that can be applied in algorithms to process the Web graph; as an
example we deploy LDA link weights in stacked graphical learning. By using
Weka's BayesNet classifier, in terms of the AUC of classification, we achieve
4% improvement over plain LDA with BayesNet and 18% over tf.idf with SVM. Our
Gibbs sampling strategies yield about 5-10 times speedup with less than 1%
decrease in accuracy in terms of likelihood and AUC of classification.
更多查看译文
关键词
web document classification,latent dirichlet allocation,topic dis- tribution,latent semantic indexing,gibbs sampling,gibbs sampler
AI 理解论文
溯源树
样例
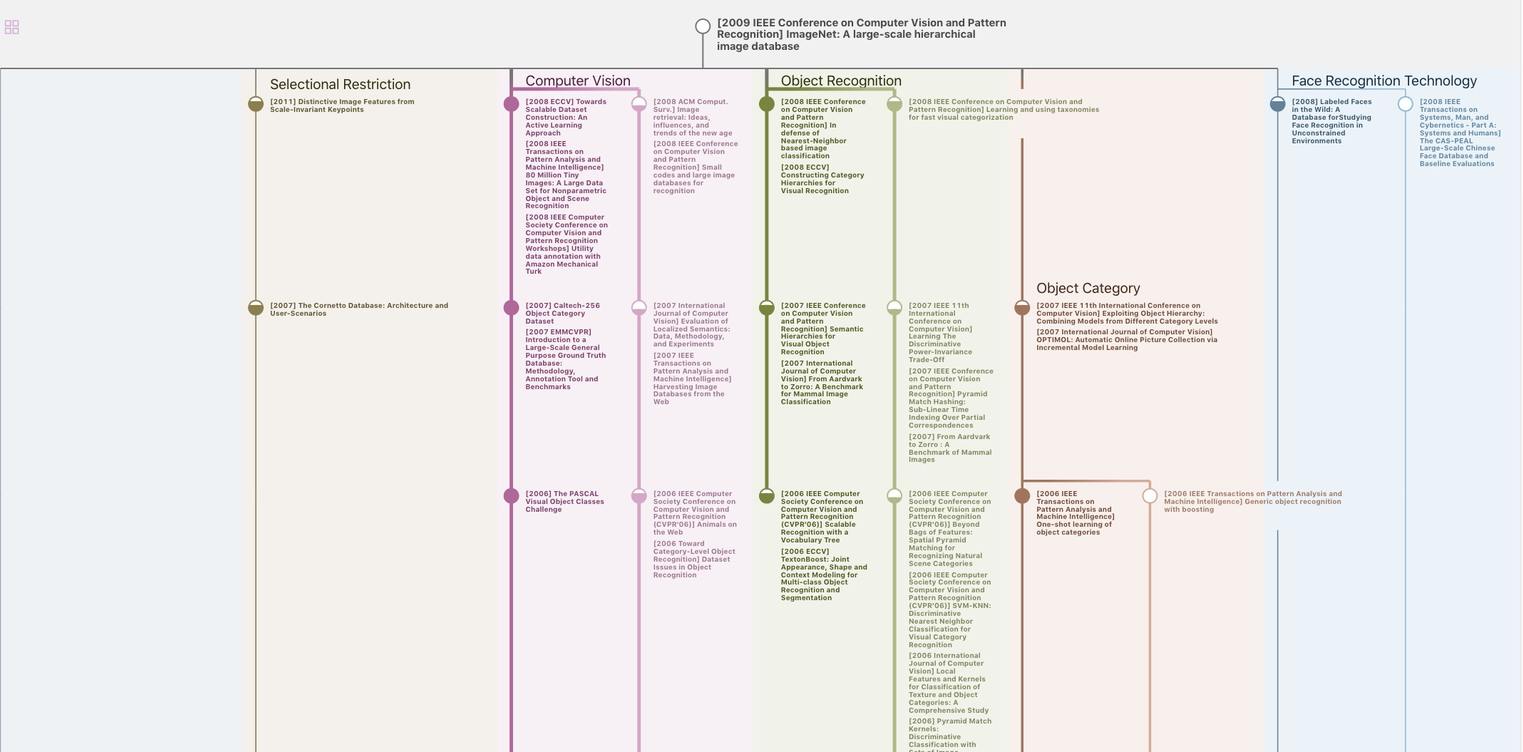
生成溯源树,研究论文发展脉络
Chat Paper
正在生成论文摘要