A Mixed Generative-Discriminative Framework For Pedestrian Classification
CVPR(2008)
摘要
This paper presents a novel approach to pedestrian classification which involves utilizing the synthesized virtual samples of a learned generative model to enhance the classification performance of a discriminative model. Our generative model captures prior knowledge about the pedestrian class in terms of a number of probabilistic shape and texture models, each attuned to a particular pedestrian pose. Active learning provides the link between the generative and discriminative model, in the sense that the former is selectively sampled such that the training process is guided towards the most informative samples of the latterIn large-scale experiments on real-world datasets of tens of thousands of samples, we demonstrate a significant improvement in classification performance of the combined generative-discriminative approach over the discriminative-only approach (the latter exemplified by a neural network with local receptive fields and a support vector machine using Haar wavelet features).
更多查看译文
关键词
Haar transforms,image classification,image enhancement,image sampling,image texture,probability,support vector machines,wavelet transforms,Haar wavelet features,active learning,classification performance,combined generative-discriminative approach,discriminative-only approach,mixed generative-discriminative framework,pedestrian classification,probabilistic shape-texture models,support vector machine,training process,
AI 理解论文
溯源树
样例
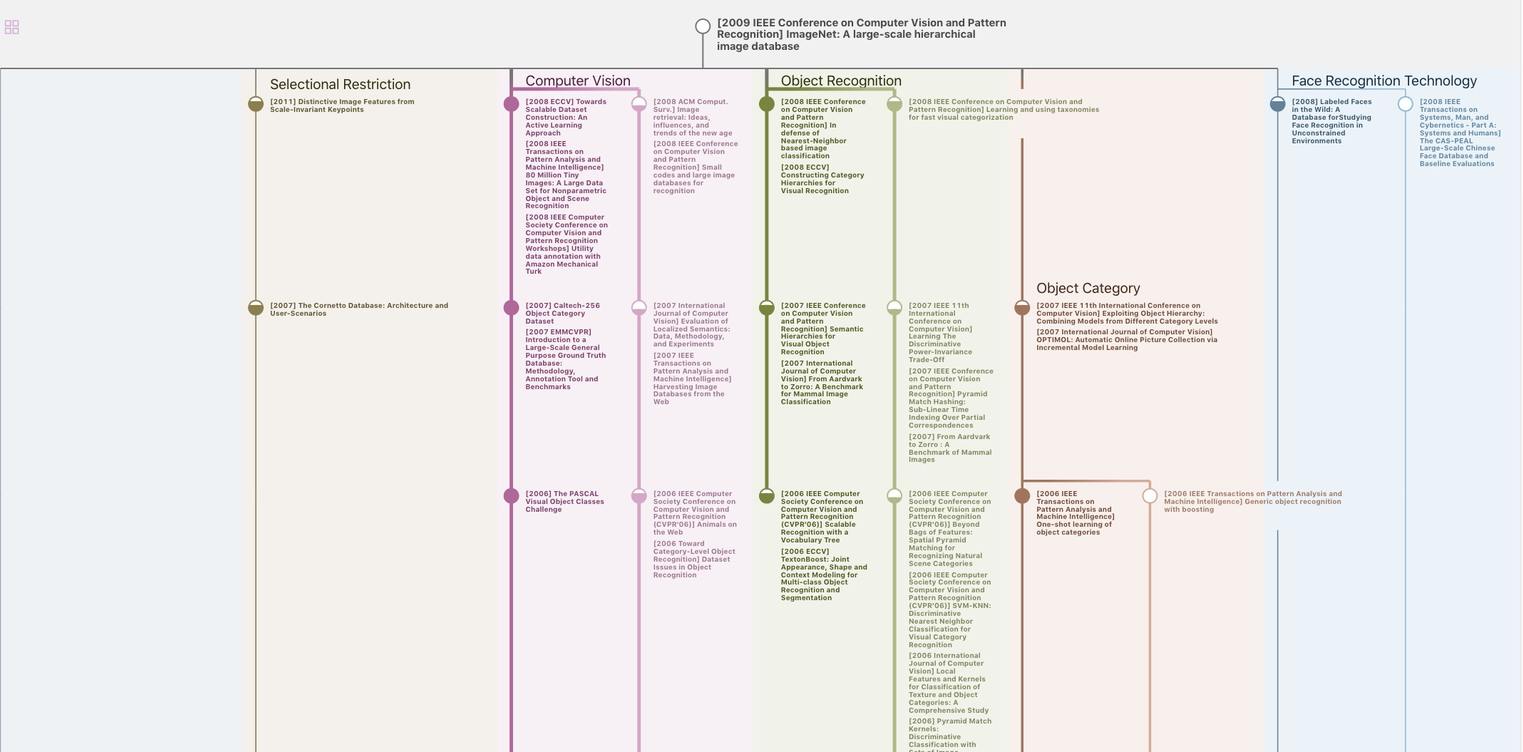
生成溯源树,研究论文发展脉络
Chat Paper
正在生成论文摘要