Stereo Where-What Networks: Unsupervised Binocular Feature Learning
2013 INTERNATIONAL JOINT CONFERENCE ON NEURAL NETWORKS (IJCNN)(2013)
摘要
Unsupervised feature learning has been shown promising in the field of machine learning. However, the learning algorithms used in these methods, e.g., Deep Belief Networks based on Restricted Boltzman Machines, are typically restricted in different ways, e. g., hard to train and calculate the partition function [1]. In this article, we present a cortexinspired learning network, Where-What Networks (WWN), for the problem of unsupervised learning of binocular local features. The results show that the learned features autonomously developed selectivity for disparity, profile and location of the input patterns. We present a novel algorithm, Dynamic Synapse Lobe Component Analysis (DSLCA), which not only resembles the pattern of neural connections in the visual cortex, but also results in the autonomous development of "domain disparity". To our knowledge, this work is the first to introduce unsupervised learning of both domain and weight disparities between left and right local receptive fields. Moreover, given the theoretical optimality of WWNs [2] and their empirically proven strength in supervised learning, the presented work is the first step towards creating a semi-supervised learning network for simultaneous type, location (including distance) and 3D shape perception.
更多查看译文
关键词
unsupervised learning
AI 理解论文
溯源树
样例
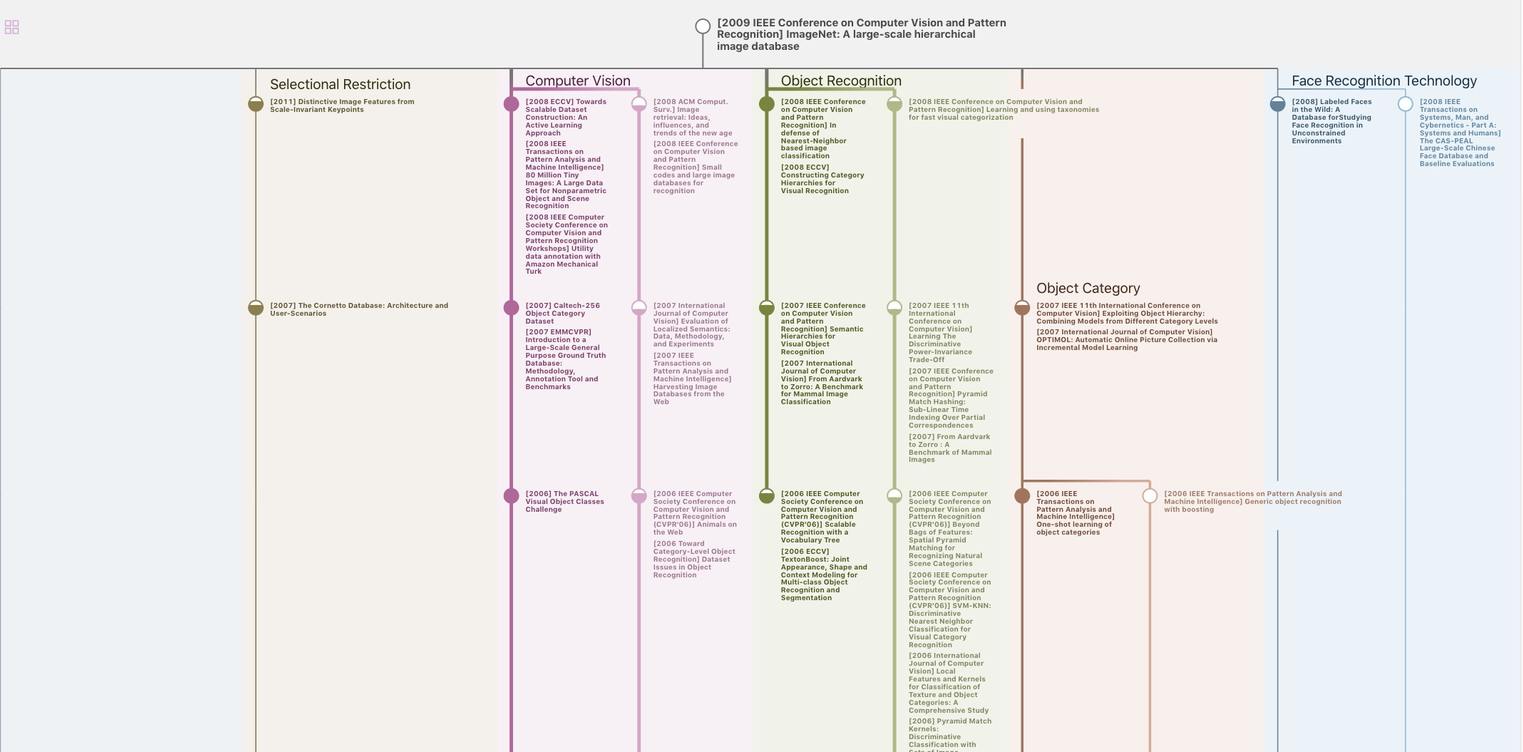
生成溯源树,研究论文发展脉络
Chat Paper
正在生成论文摘要