Variational Bayesian Methods For Multimedia Problems
IEEE Transactions on Multimedia(2014)
摘要
In this paper we present an introduction to Variational Bayesian (VB) methods in the context of probabilistic graphical models, and discuss their application in multimedia related problems. VB is a family of deterministic probability distribution approximation procedures that offer distinct advantages over alternative approaches based on stochastic sampling and those providing only point estimates. VB inference is flexible to be applied in different practical problems, yet is broad enough to subsume as its special cases several alternative inference approaches including Maximum A Posteriori (MAP) and the Expectation-Maximization (EM) algorithm. In this paper we also show the connections between VB and other posterior approximation methods such as the marginalization-based Loopy Belief Propagation (LBP) and the Expectation Propagation (EP) algorithms. Specifically, both VB and EP are variational methods that minimize functionals based on the Kullback-Leibler (KL) divergence. LBP, traditionally developed using graphical models, can also be viewed as a VB inference procedure. We present several multimedia related applications illustrating the use and effectiveness of the VB algorithms discussed herein. We hope that by reading this tutorial the readers will obtain a general understanding of Bayesian methods and establish connections among popular algorithms used in practice.
更多查看译文
关键词
Bayes methods,Graphical models,Multimedia communication,Inverse problems,Probabilistic logic,Streaming media,Approximation methods
AI 理解论文
溯源树
样例
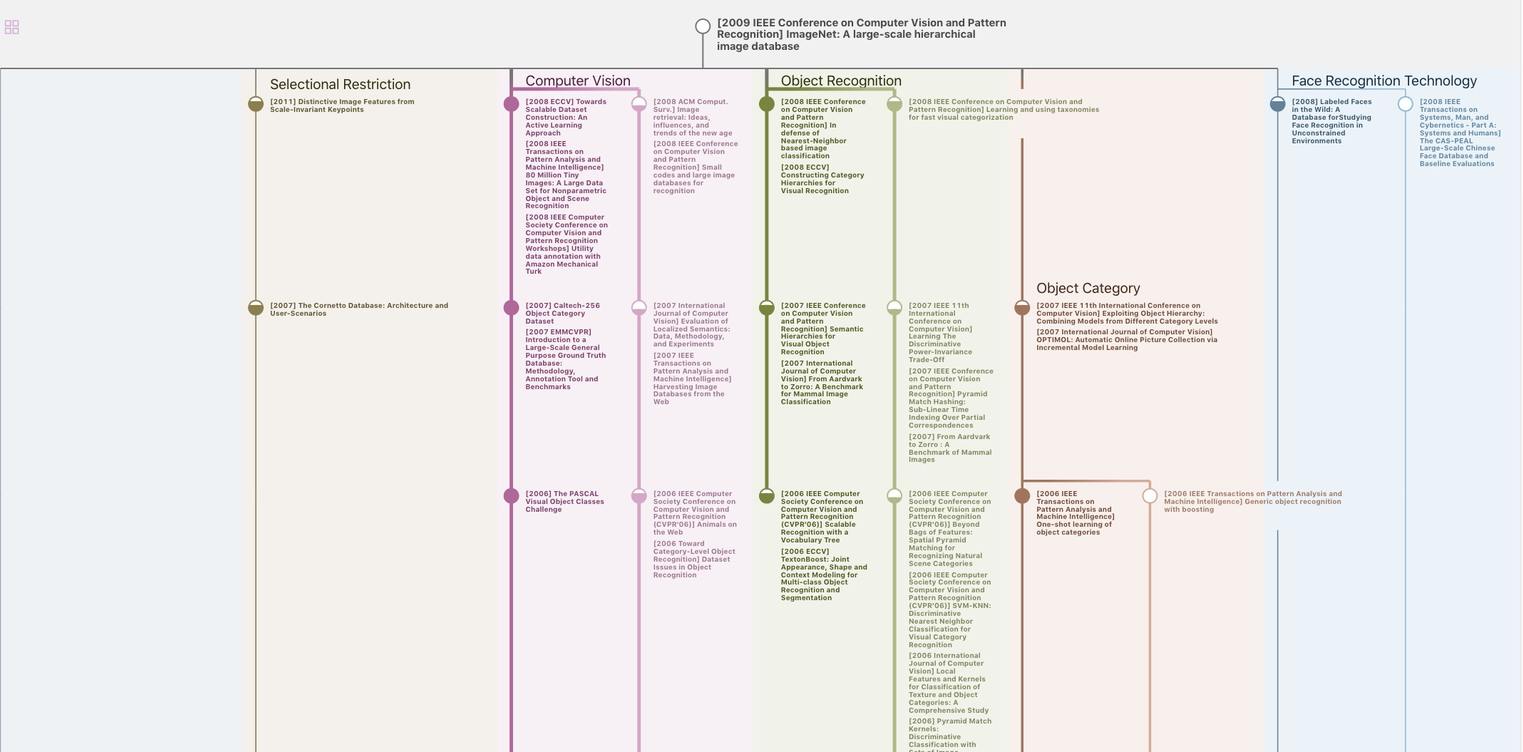
生成溯源树,研究论文发展脉络
Chat Paper
正在生成论文摘要