Hierarchical Bottom-Up Safe Semi-Supervised Support Vector Machines for Multi-Class Transductive Learning.
JIDM(2013)
摘要
Semi-supervised approaches have been successfully applied to many machine learning problems. A particular case of semi-supervised settings is transductive learning, in which the goal is solely to label the available unlabeled data, instead of generating a predictive model that should generalize well to unseen data. Nevertheless, there are cases in which a transductive learner may perform even worse than an inductive learner that is trained with only the labeled data. For alleviating this problem, an effort towards safe semi-supervised support vector machines (S4VM) was made, so that a transductive SVM would never degenerate the performance when compared to its inductive counterpart. Even though robust, S4VM still lacks the ability of naturally dealing with multi-class problems, having to rely on multi- class encoding schemes, such as one-vs-one and one-vs-all strategies. These schemes may not take advantage of the full potential of S4VM, specially in complex multi-class problems with overlapping classes. In this article, we address this problem by providing a binary-tree scheme for aggregating distinct S4VMs in a bottom-up fashion. The proposed approach is named HiBUST, which stands for Hierarchical Bottom-Up S4VM Tree. Experimental results show that HiBUST can provide increased predictive performance for many multi-class problems when compared to a one-vs-one S4VM. In addition, we show that HiBUST is also beneficial for complex binary-class problems.
更多查看译文
关键词
transductive learning,semi supervised learning
AI 理解论文
溯源树
样例
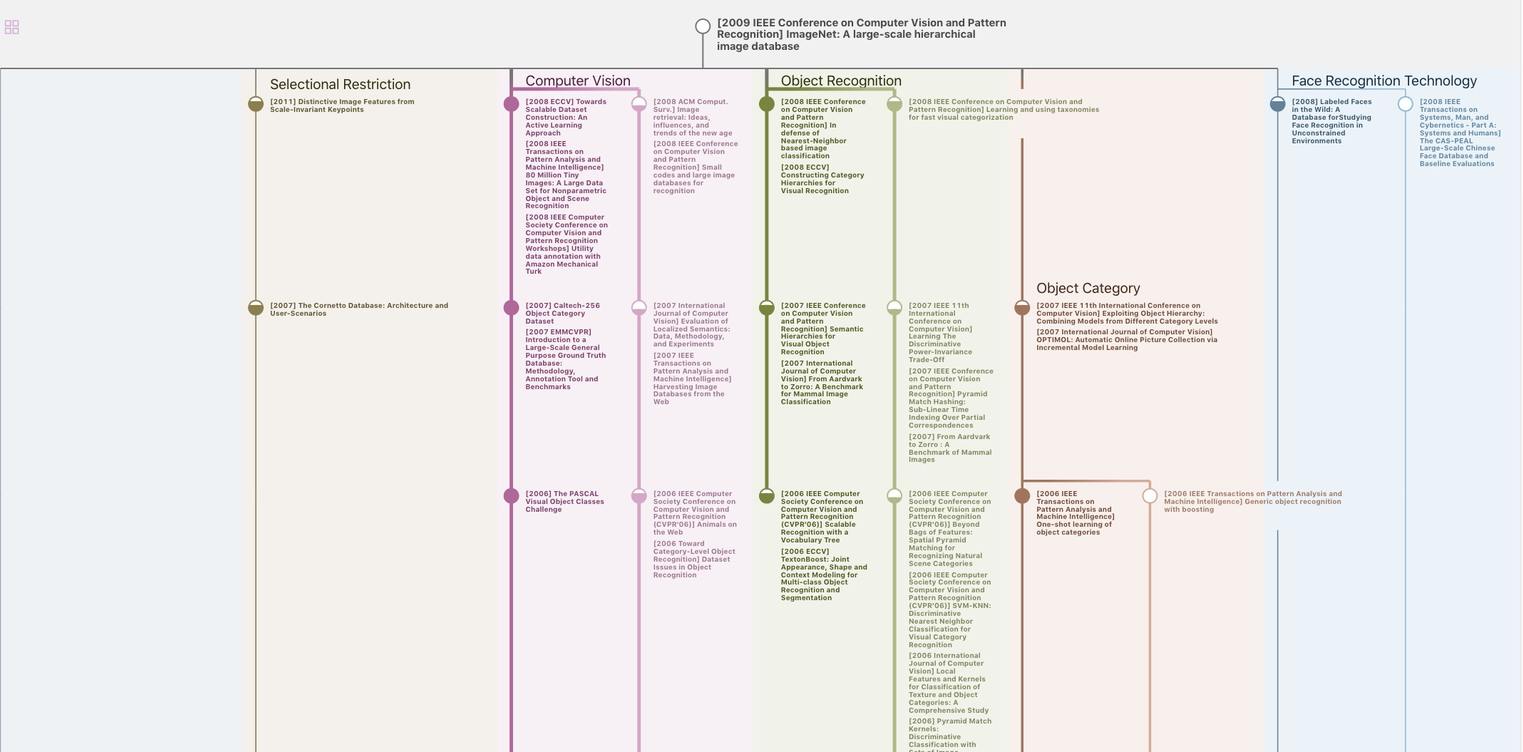
生成溯源树,研究论文发展脉络
Chat Paper
正在生成论文摘要