Emotional speaker recognition based on i-vector through Atom Aligned Sparse Representation
ICASSP(2013)
摘要
I-vector algorithm was previously adopted to improve the performance of ASR (Automatic Speaker Recognition) system which is degraded by emotion variability. The variability compensation technique is LDA (Linear Discriminant Analysis) which assumes the variability is speaker-independent. However, this assumption is not suitable for emotion variability because we discover that the pattern of emotion variability is speaker-dependent. Therefore, a novel emotion synthesis algorithm AASR (Atom Aligned Sparse Representation) is proposed to characterize this speaker-dependent pattern and compensate the emotion variability within i-vectors. The experiments conducted on MASC show that our algorithm, compared with the GMM-UBM algorithm and the conventional variability compensation algorithm LDA, both can enhance the speaker identification and verification performances.
更多查看译文
关键词
image representation,automatic speaker recognition,gmm-ubm algorithm,masc,emotional speaker recognition,speaker-dependent variability,emotion synthesis algorithm,i-vector algorithm,atom aligned sparse representation,linear discriminant analysis,speaker recognition,emotion recognition,lda,speaker identification,asr system,compensation,aasr,emotion variability compensation technique,speaker-dependent pattern,speaker verification,dictionaries,vectors,sparse matrices,speech
AI 理解论文
溯源树
样例
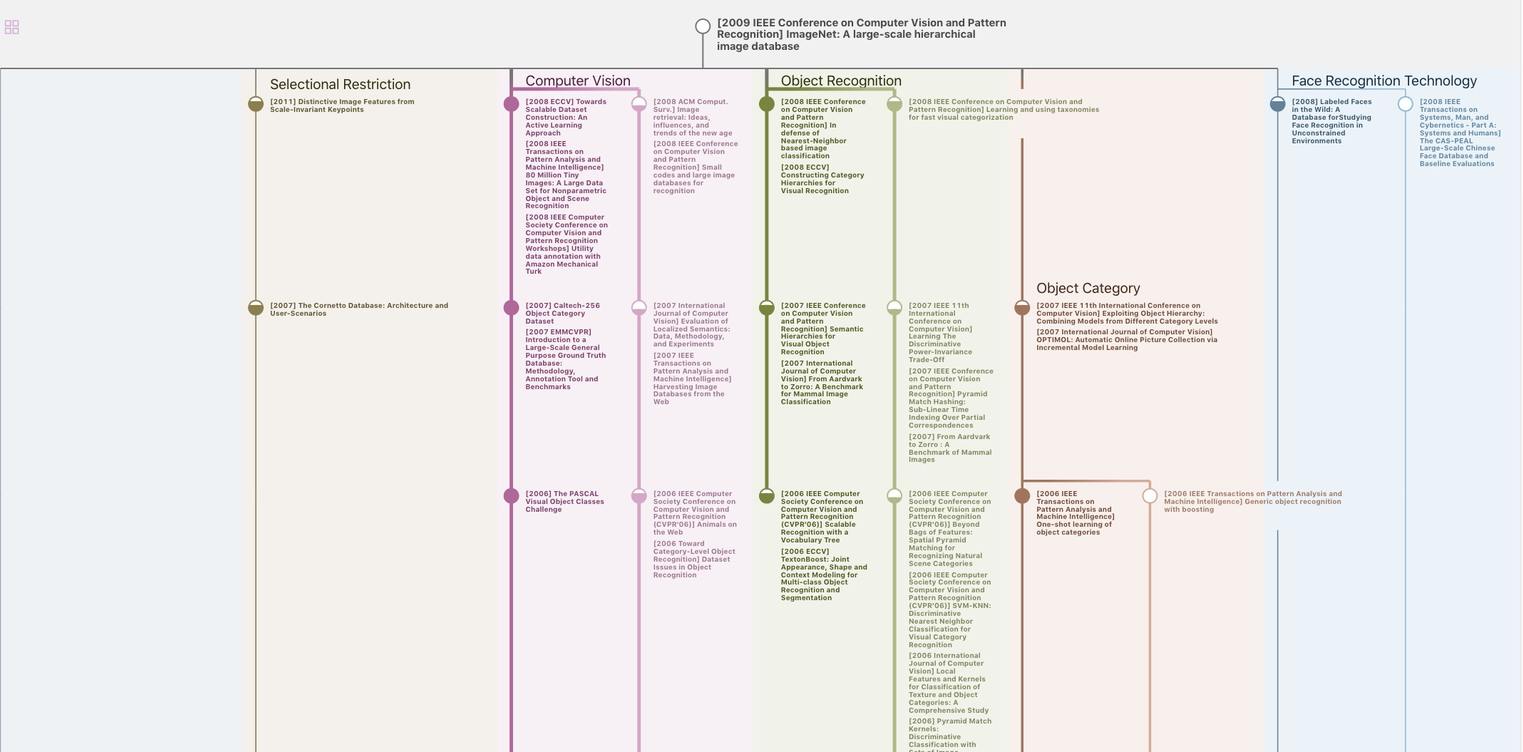
生成溯源树,研究论文发展脉络
Chat Paper
正在生成论文摘要