Testing the Gauss linear assumption for on-line predictions
Progress in Artificial Intelligence(2012)
摘要
The article studies prediction under some background assumptions and tests the assumptions. We use a conformal predictors framework and obtain predictions as regions. This is done by an on-line compression model that satisfies our assumptions. If the assumptions are appropriate for data, the prediction regions are automatically valid. The validity means that for successive predictions an error rate does not exceed a prefixed significance level (up to statistical fluctuation). It is important to test our assumptions to maintain the validity property; or otherwise the predictions can be misleading. We perform testing by calculating martingales. The martingales here are functions of p -values that are the output of the on-line compression model. Large values of the martingales reflect departures from the assumptions. This article extends our previous work on martingale testing and focusses on the Gauss linear assumption. The article presents experiments for the predictions and testing. We use four synthetic datasets as well as a benchmark Abalone dataset that is assumed to agree with the Gauss linear assumption.
更多查看译文
关键词
Testing assumptions,Martingales,Gauss linear assumption,Conformal predictors
AI 理解论文
溯源树
样例
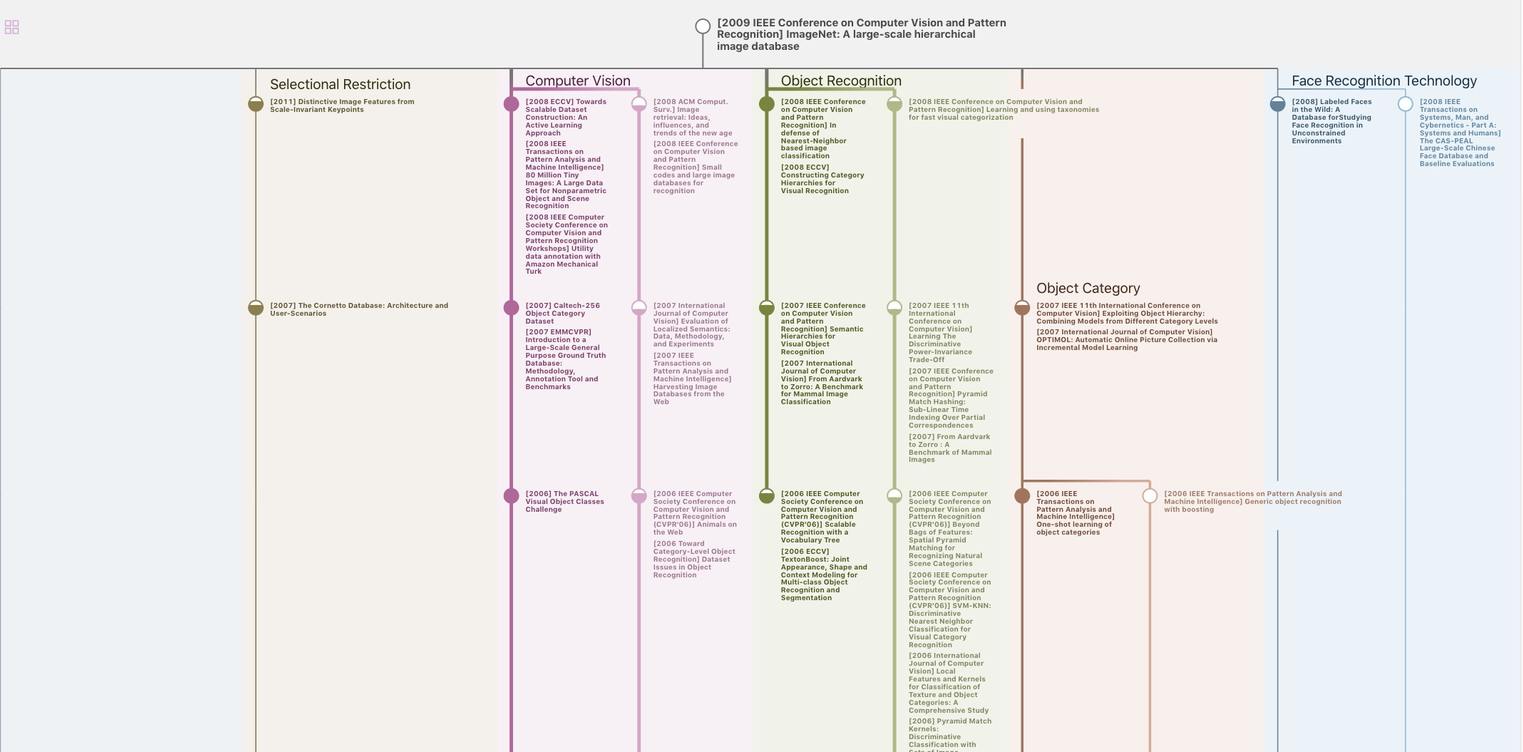
生成溯源树,研究论文发展脉络
Chat Paper
正在生成论文摘要