A Non-negative Matrix Factorization Framework for Combining Multiple Image Segmentations
msra(2008)
摘要
Segmentation, or partitioning images into internally ho- mogeneous regions, is an important first step in many Com- puter Vision tasks. In this paper, we attack the segmen- tation problem using an ensemble of low cost image seg- mentations. These segmentations are reconciled by apply- ing recent techniques from the consensus clustering liter- ature which exploit a Non-negative Matrix Factorization (NMF) framework. We describe extensions to these methods that scale them for large images and incorporate smooth- ness constraints. This framework allows us to uniformly combine segmentations from different algorithms or featur e modalities, while avoiding significant parameter tuning fo r the specific image being segmented. We demonstrate that combining multiple "naive" image segmentations derived from k-means clustering compares favorably with more ad- vanced Mean Shift and Efficient Graph Based Segmentation algorithms. The approach is evaluated on the Berkeley im- age segmentation dataset.
更多查看译文
AI 理解论文
溯源树
样例
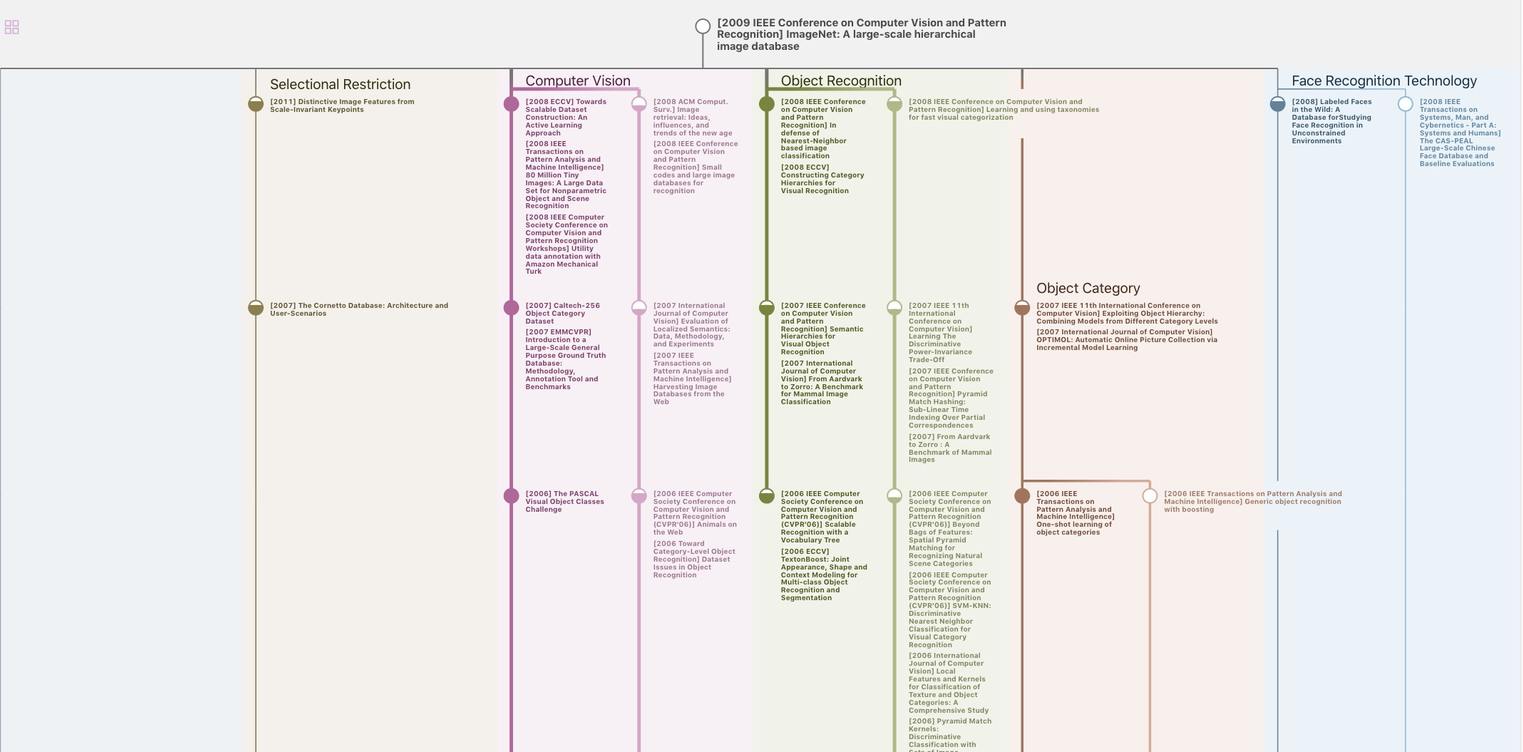
生成溯源树,研究论文发展脉络
Chat Paper
正在生成论文摘要