Manifold learning techniques for non-rigid structure from motion
Manifold learning techniques for non-rigid structure from motion(2009)
摘要
This dissertation establishes the foundations for treating non-rigid structure from motion as a manifold learning problem. Non-rigid structure from motion (or NRSFM) is the computer vision technique that recovers the 3D structure of a deformable object from a monocular video sequence. State of the art techniques assume that the object is generated from a linear combination of basis shapes and several techniques (like EM or matrix decomposition) are then used to exploit this assumption. So far, nobody has ever considered this setup as a linear manifold learning problem. In this dissertation, we show how NRSFM can be formulated as a manifold embedding recovery problem. First, the different frames of the video sequence are embedded in a low dimensional manifold, from which the shape coordinates are discovered and then used to recover the camera parameters and the shape basis. We also push the limits of this model by relaxing the linearity assumption of the manifold and only constraining its dimensionality. This looser assumption is proven to be much more valid than the simple linearity one for certain objects where only the number of degrees of freedom is known. To solve this problem, a new manifold learning technique, named LSML, is introduced. LSML focuses on learning tangents rather than points themselves as done in conventional manifold learning. Both methods are validated and compared with state of the art techniques on real and synthetic data.
更多查看译文
关键词
low dimensional manifold,linear manifold,conventional manifold learning,looser assumption,art technique,non-rigid structure,manifold embedding recovery problem,basis shape,linearity assumption,new manifold
AI 理解论文
溯源树
样例
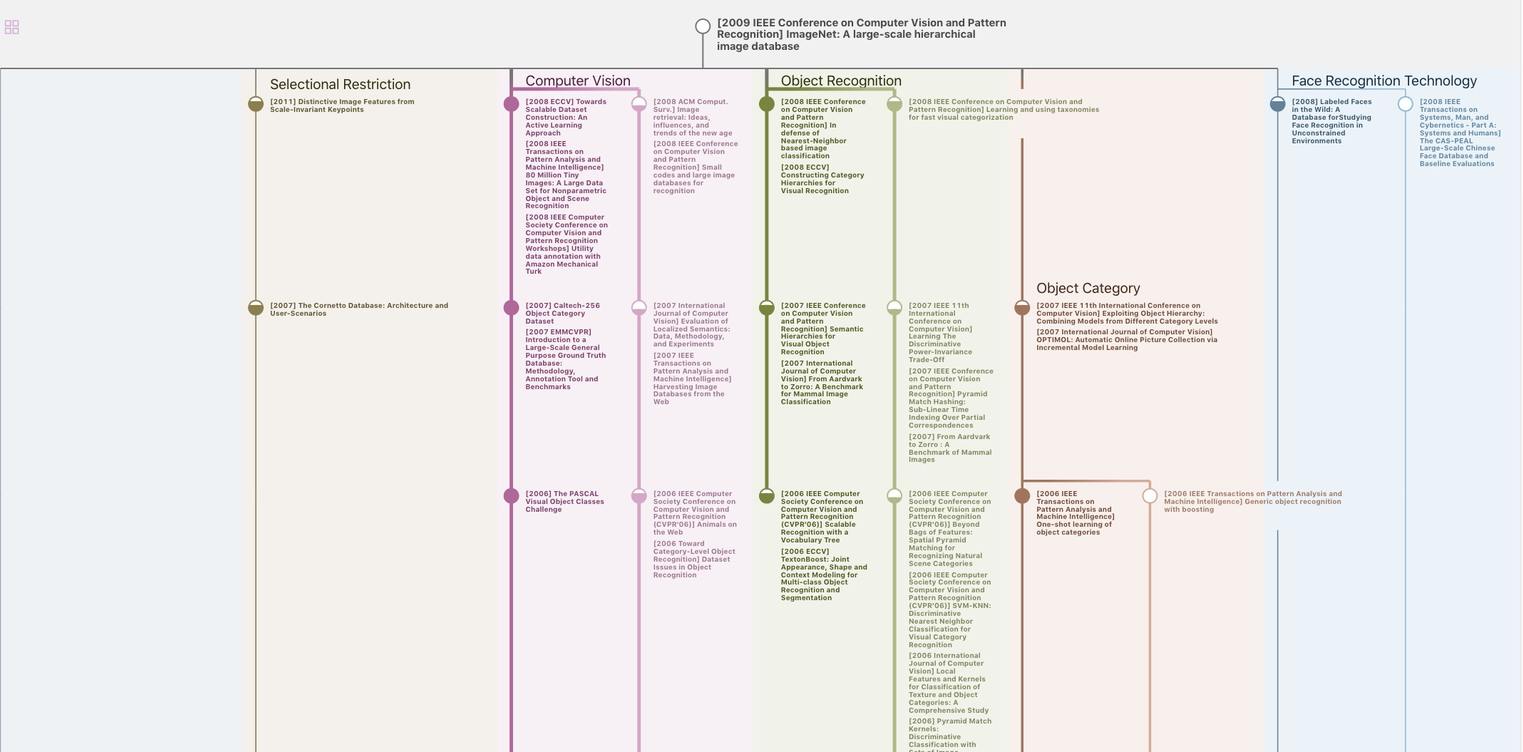
生成溯源树,研究论文发展脉络
Chat Paper
正在生成论文摘要