Clustering Ios Executable Using Self-Organizing Maps
2013 INTERNATIONAL JOINT CONFERENCE ON NEURAL NETWORKS (IJCNN)(2013)
摘要
We pioneer the study on applying both SOMs and GHSOMs to cluster mobile apps based on their behaviors, showing that the SOM family works well for clustering samples with more than ten thousands of attributes. The behaviors of apps are characterized by system method calls that are embedded in their executable, but may not be perceived by users. In the data preprocessing stage, we propose a novel static binary analysis to resolve and count implicit system method calls of iOS executable. Since an app can make thousands of system method calls, it is needed a large dimension of attributes to model their behaviors faithfully. On collecting 115 apps directly downloaded from Apple app store, the analysis result shows that each app sample is represented with 18000+ kinds of methods as their attributes. Theoretically, such a sample representation with more than ten thousand attributes raises a challenge to traditional clustering mechanisms. However, our experimental result shows that apps that have similar behaviors (due to having been developed from the same company or providing similar services) can be clustered together via both SOMs and GHSOMs.
更多查看译文
关键词
conformal mapping,mobile computing,neural networks,data preprocessing
AI 理解论文
溯源树
样例
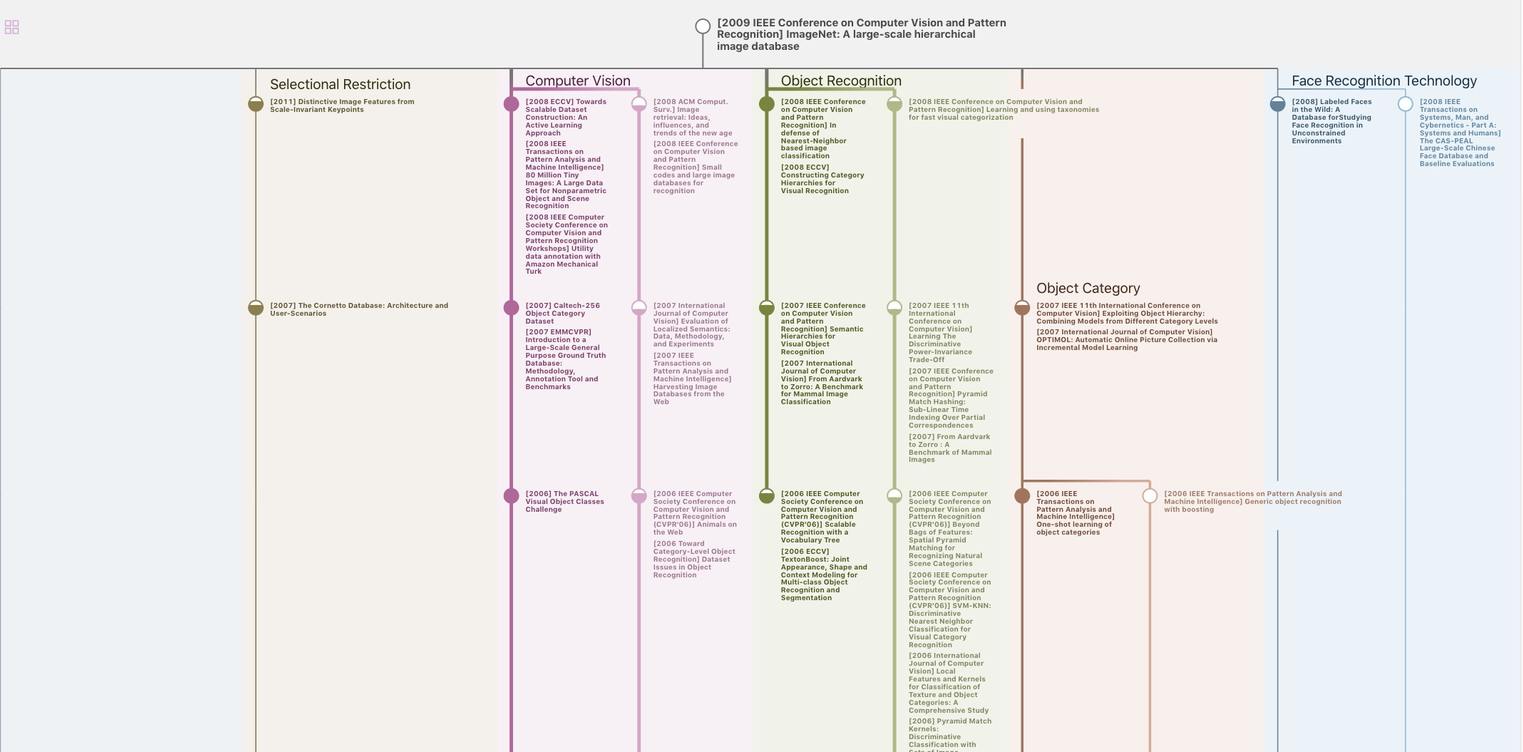
生成溯源树,研究论文发展脉络
Chat Paper
正在生成论文摘要