Multivariate process and quality monitoring applied to an electrolysis process Part II. Multivariate time-series analysis of lagged latent variables
CHEMOMETRICS AND INTELLIGENT LABORATORY SYSTEMS(1998)
摘要
Multivariate time series analysis is applied to understand and model the dynamics of an electrolytic process manufacturing copper. Here, eight metal impurities were measured, twice daily, over a period of one year, to characterize the quality of the copper. In the data analysis, these eight variables were summarized by means of principal component analysis (PCA). Two principal component (PC) scores were sufficient to well summarize the eight measured variables (R-2 = 0.67). Subsequently, the dynamics of these PC-scores (latent variables) were investigated using multivariate time series analysis, i.e., partial least squares (PLS) modelling of the lagged latent variables. Stochastic models of the auto-regressive moving average (ARMA) family were appropriate for both PC-scores. Hence, the dynamics of both scores make the exponentially weighted moving average (EWMA) control chart suitable for process monitoring. (C) 1998 Elsevier Science B.V. All rights reserved.
更多查看译文
关键词
multivariate time series analysis,PLS,PCA,EWMA,control charts
AI 理解论文
溯源树
样例
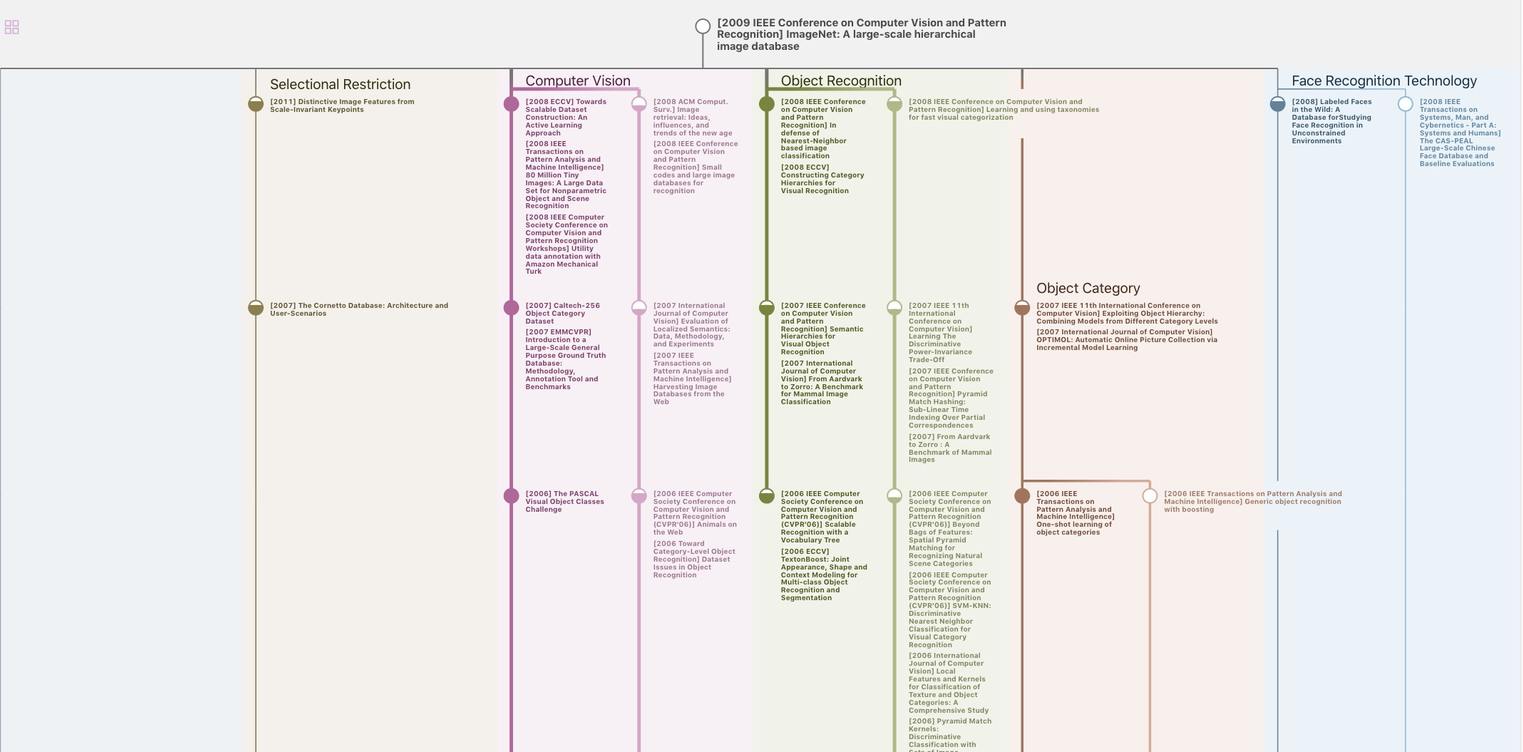
生成溯源树,研究论文发展脉络
Chat Paper
正在生成论文摘要