A Semi-Supervised Batch-Mode Active Learning Strategy for Improved Statistical Machine Translation.
Conference on Computational Natural Language Learning(2010)
摘要
The availability of substantial, in-domain parallel corpora is critical for the development of high-performance statistical machine translation (SMT) systems. Such corpora, however, are expensive to produce due to the labor intensive nature of manual translation. We propose to alleviate this problem with a novel, semi-supervised, batch-mode active learning strategy that attempts to maximize indomain coverage by selecting sentences, which represent a balance between domain match, translation difficulty, and batch diversity. Simulation experiments on an English-to-Pashto translation task show that the proposed strategy not only outperforms the random selection baseline, but also traditional active learning techniques based on dissimilarity to existing training data. Our approach achieves a relative improvement of 45.9% in BLEU over the seed baseline, while the closest competitor gained only 24.8% with the same number of selected sentences.
更多查看译文
关键词
English-to-Pashto translation task show,high-performance statistical machine translation,manual translation,translation difficulty,batch-mode active learning strategy,proposed strategy,random selection baseline,seed baseline,traditional active learning technique,batch diversity,improved statistical machine translation,semi-supervised batch-mode active learning
AI 理解论文
溯源树
样例
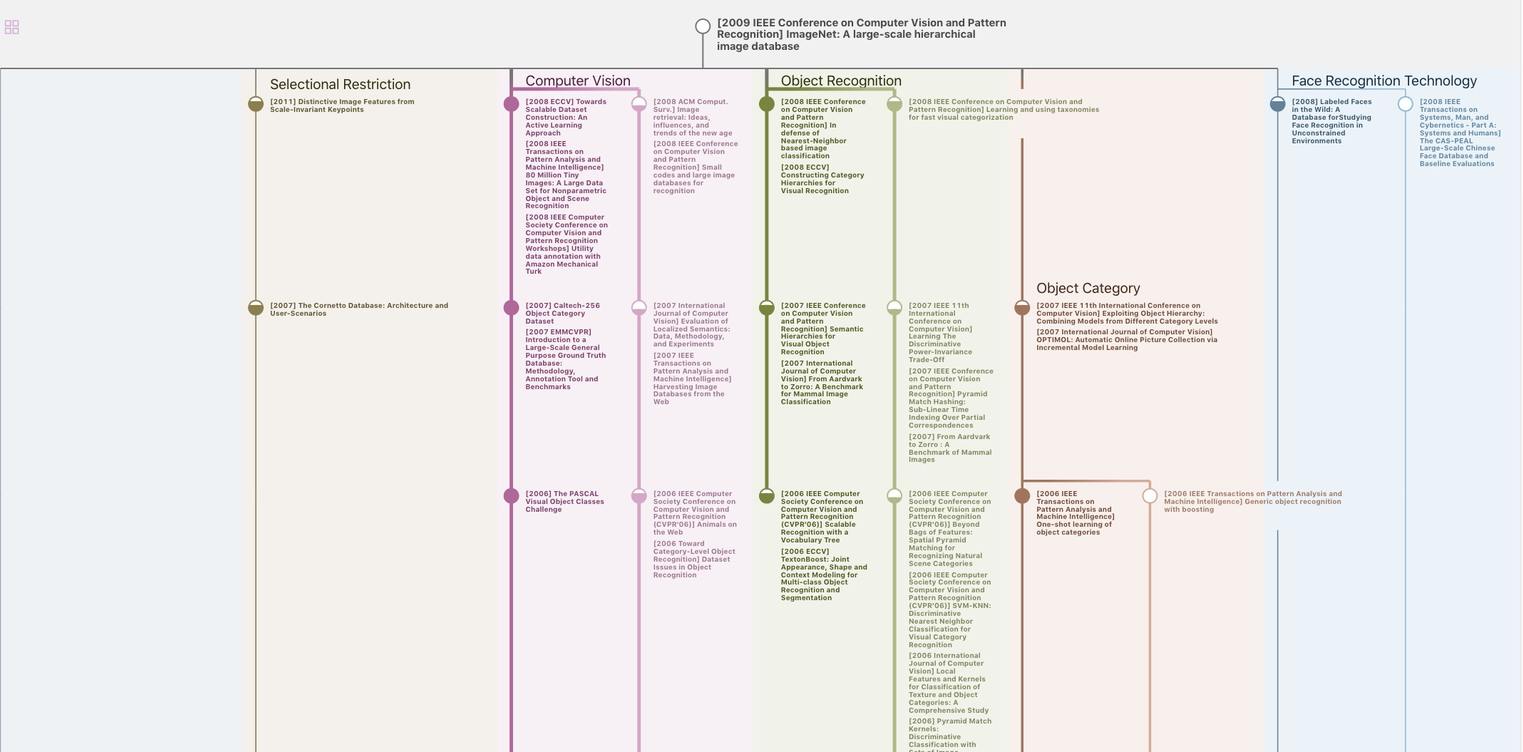
生成溯源树,研究论文发展脉络
Chat Paper
正在生成论文摘要