From Plan Traces to Hierarchical Task Networks Using Reinforcements: A Preliminary Report
msra(2011)
摘要
A key challenge of automated planning is the requirement of a domain expert to provide some sort of background planning knowledge about the dynamics of the planning domain. At a minimum, classical planners require semantic descriptions (i.e., preconditions and effects) of possible actions. More recent planning paradigms allow or require the expert to provide additional knowledge about the structural properties of the domain and problem-solving strategies. In many realistic planning domains, however, additional planning knowledge may not be completely available; this is partly because it is very difficult for the experts to compile such knowledge due to the complexities in the domains and it is partly because there is limited access to an expert to provide it. Thus, it is crucial to develop systems in order to learn planning knowledge when human contributions are limited or unavailable. One of the best-known approaches for modeling planning knowledge about a problem domain is Hierarchical Task Networks (HTNs). An HTN planner formulates a plan via task-decomposition methods (also known as HTN methods), which describe how to decompose complex tasks (i.e., symbolic representations of activities to be performed) into simpler subtasks until tasks are reached that correspond to actions that can be performed directly. The basic idea was developed in the mid-70s [Sacerdoti, 1975], and the formal underpinnings were developed in the mid-90s [Erol et al., 1996]. More recently, the HTN planner SHOP [Nau et al., 1999] has demonstrated impressive speed gains over earlier classical planners by using HTN-based domain-specific strategies for problem-solving while performing domain-independent search. HTNs provide a natural modeling framework in many real-world applications including evacuation planning, manufacturing, and UAV management planning [Nau et al., 2005]. Over the years, researchers have developed several systems that are capable of learning HTNs. Some concentrate on learning applicability conditions of given HTNs such as CaMEL [Ilghami et al., 2005] and DiNCAD [Xu and MunozAvila, 2005], while others learn both preconditions and the task decompositions – i.e., the hierarchical structure relat-
更多查看译文
AI 理解论文
溯源树
样例
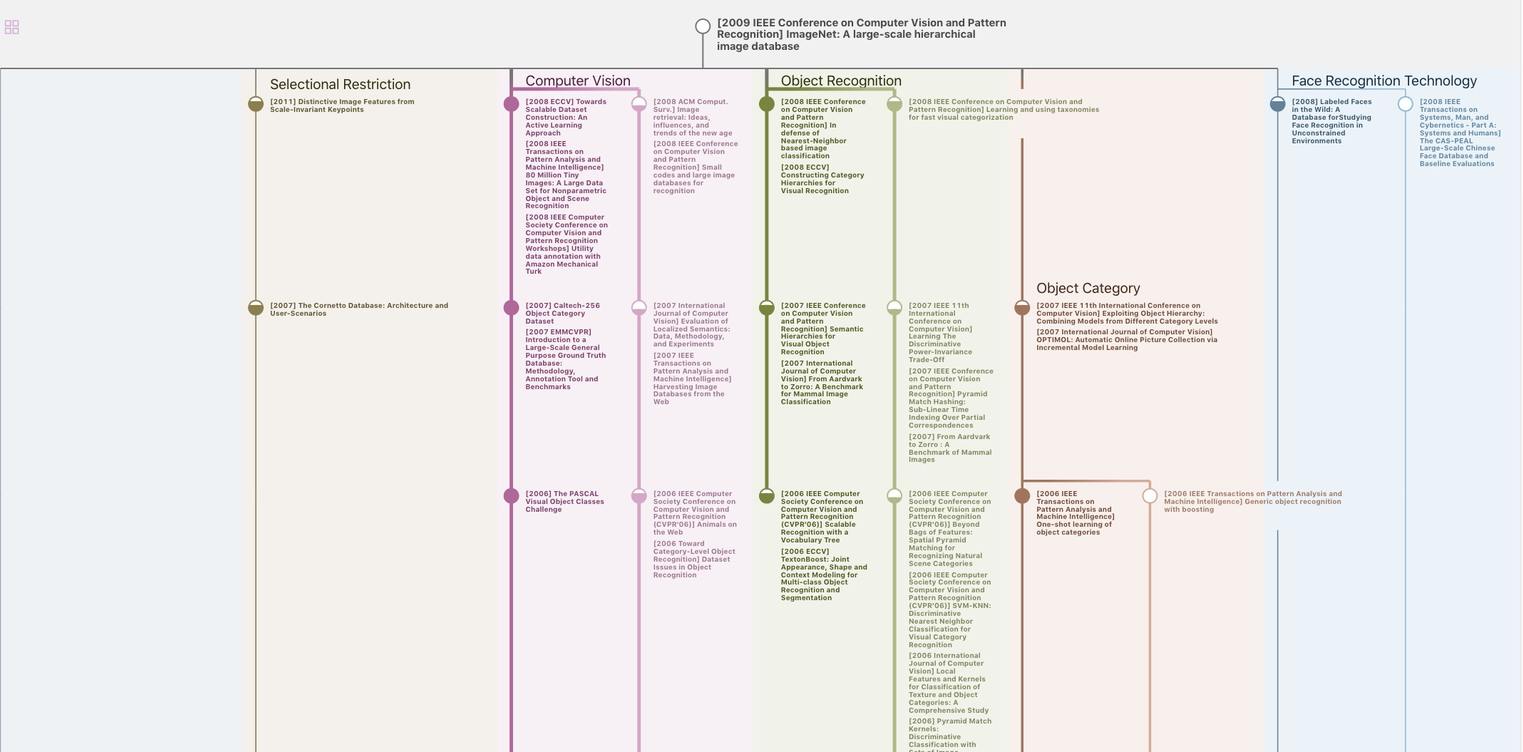
生成溯源树,研究论文发展脉络
Chat Paper
正在生成论文摘要