Multiple Instance Learning Of Pulmonary Embolism Detection With Geodesic Distance Along Vascular Structure
2007 IEEE CONFERENCE ON COMPUTER VISION AND PATTERN RECOGNITION, VOLS 1-8(2007)
摘要
We propose a novel classification approach for automatically detecting pulmonary embolism (PE) from computed-tomography-angiography images. Unlike most existing approaches that require vessel segmentation to restrict the search space for PEs, our toboggan-based candidate generator is capable of searching the entire lung for any suspicious regions quickly and efficiently. We then exploit the spatial information supplied in the vascular structure as a post-candidate-generation step by designing classifiers with geodesic distances between candidates along the vascular tree. Moreover, a PE represents a cluster of voxels in an image, and thus multiple candidates can be associated with a single PE and the PE is identified if any of its candidates is correctly classified. The proposed algorithm also provides an efficient solution to the problem of learning with multiple positive instances. Our clinical studies with 177 clinical cases demonstrate that the proposed approach outperforms existing detection methods, achieving 81% sensitivity on an independent test set at 4 false positives per study.
更多查看译文
关键词
image classification,bismuth,clustering algorithms,search space,biomedical imaging,learning artificial intelligence,false positive,image segmentation,spatial information,geodesic distance
AI 理解论文
溯源树
样例
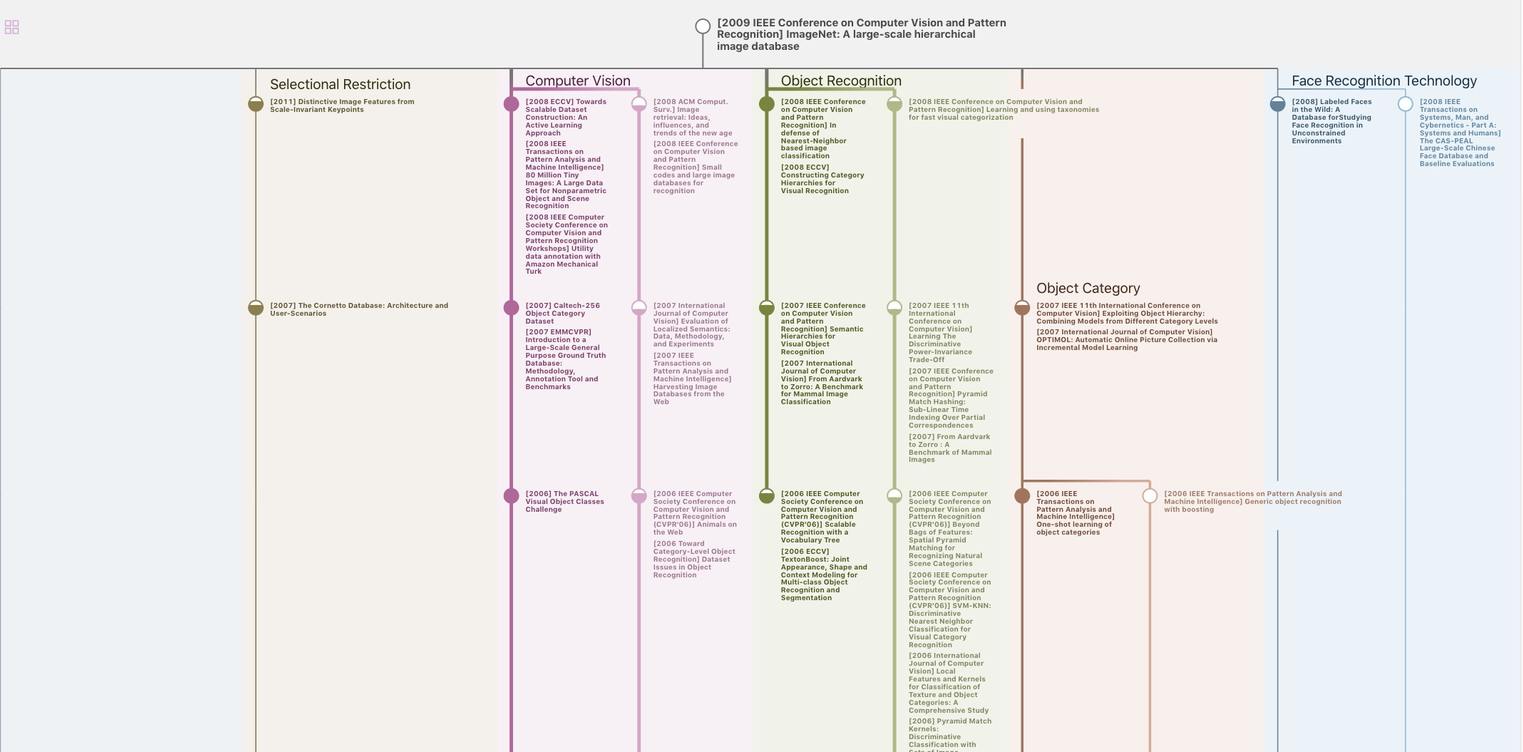
生成溯源树,研究论文发展脉络
Chat Paper
正在生成论文摘要