Inference Of Co-Occurring Classes: Multi-Class And Multi-Label Classification
COMPUTATIONAL INTELLIGENCE PARADIGMS IN ADVANCED PATTERN CLASSIFICATION(2012)
摘要
The inference of co-occurring classes, i.e. multi-class and multi-label classification, is relevant to various aspects of human cognition, human-machine interactions and to the analysis of knowledge domains and processes that have traditionally been investigated in the social sciences, life sciences and humanities. Human knowledge representations usually comprise multiple classes which are rarely mutually exclusive. Each instance (sample) can belong to one or more of these classes. However, full labeling is not always possible, and the size of the consistently labeled is often limited. The level of existence of a class often varies between instances or sub-classes. The features that distinguish the classes are not always known, and can be different between classes. Hence, methods should be devised to perform multi-class and multi-label classification, and to approach the challenges entailed in the complex knowledge domains. This chapter surveys current approaches to multi-class and multi-label classification in various knowledge domains, and approaches to data annotation (labeling). In particular, it presents a classification algorithm designed for inferring the levels of co-occurring affective states (emotions, mental states, attitudes etc.) from their non-verbal expressions in speech.
更多查看译文
AI 理解论文
溯源树
样例
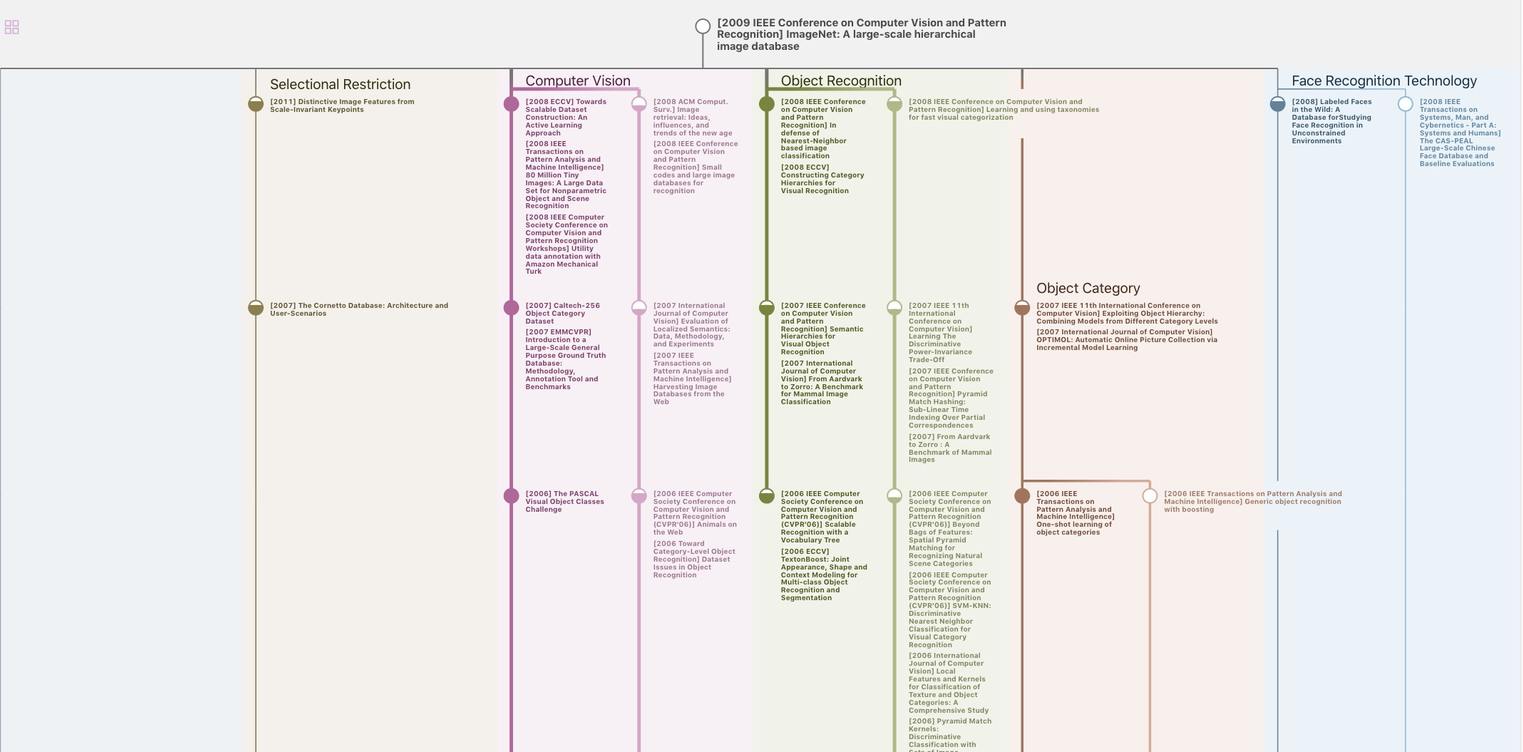
生成溯源树,研究论文发展脉络
Chat Paper
正在生成论文摘要