Large-scale bot detection for search engines.
WWW(2010)
摘要
ABSTRACTIn this paper, we propose a semi-supervised learning approach for classifying program (bot) generated web search traffic from that of genuine human users. The work is motivated by the challenge that the enormous amount of search data pose to traditional approaches that rely on fully annotated training samples. We propose a semi-supervised framework that addresses the problem in multiple fronts. First, we use the CAPTCHA technique and simple heuristics to extract from the data logs a large set of training samples with initial labels, though directly using these training data is problematic because the data thus sampled are biased. To tackle this problem, we further develop a semi-supervised learning algorithm to take advantage of the unlabeled data to improve the classification performance. These two proposed algorithms can be seamlessly combined and very cost efficient to scale the training process. In our experiment, the proposed approach showed significant (i.e. 2:1) improvement compared to the traditional supervised approach.
更多查看译文
AI 理解论文
溯源树
样例
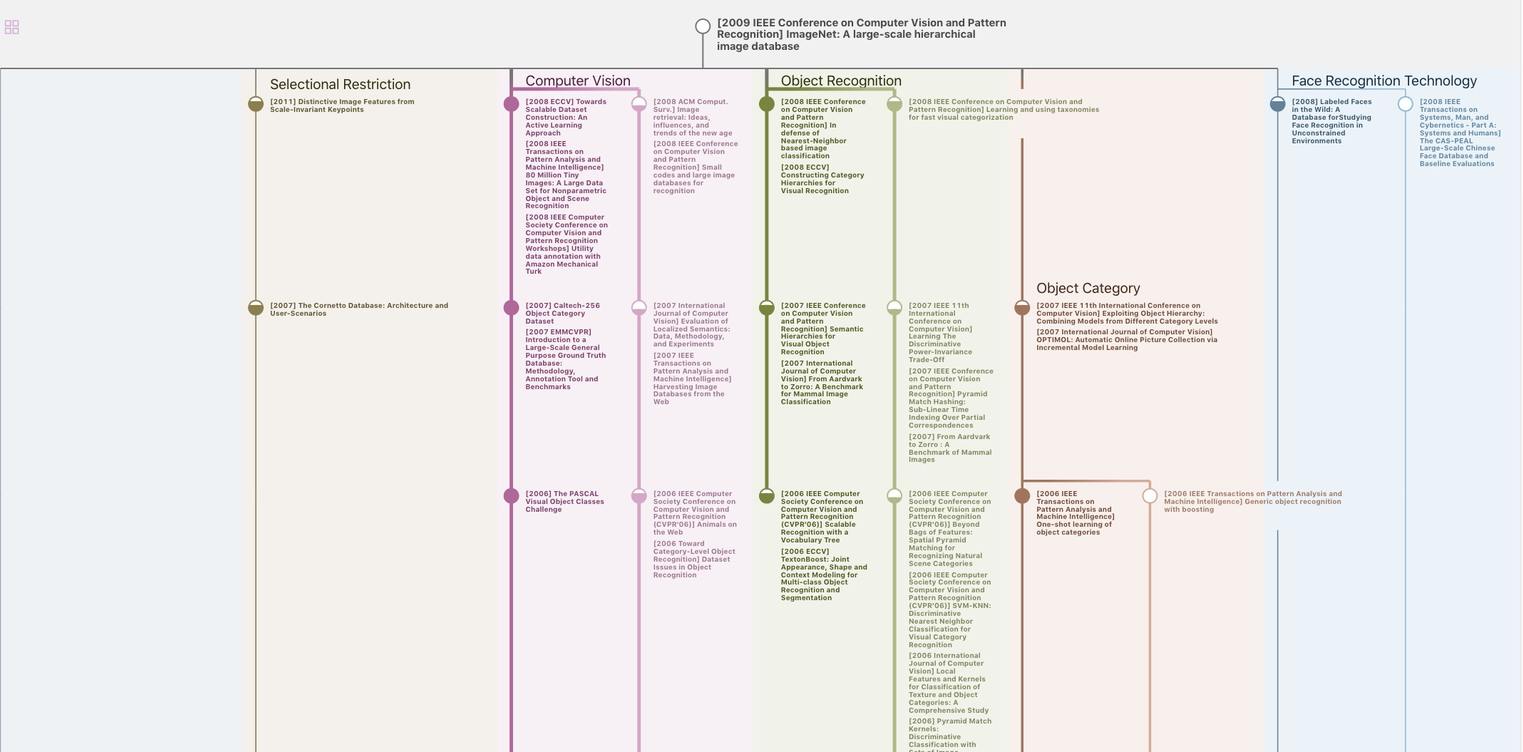
生成溯源树,研究论文发展脉络
Chat Paper
正在生成论文摘要