Japanese Dependency Analysis Based On Improved Svm And Knn
SMO'07: Proceedings of the 7th WSEAS International Conference on Simulation, Modelling and Optimization(2007)
摘要
This paper presents a method of Japanese dependency structure analysis based on improved Support Vector Machine (SVM). Japanese dependency analyzer based on SVM has been proposed and has achieved high accuracy. The efficient way to improve dependency accuracy farther is to increase the training data. However, the increase of training data will bring a great amount of training cost and decrease the parsing efficiency. We delete those samples that are unused or not good to improve the classifier's performance, and then train the reduced training set with SVM to obtain the final classifier. Furthermore, we combine improved SVM with K nearest neighbors(KNN) to improve the performance of dependency analyzer. Experiments using the Kyoto University Corpus show that the method outperforms previous systems as well as the dependency accuracy and the parsing efficiency.
更多查看译文
关键词
Japanese dependency analysis,Support Vector Machine(SVM),improved SVM,large training set SVM (LSVM),nearest neighbor-SVM (NN-SVM),K nearest neighbors(KNN)
AI 理解论文
溯源树
样例
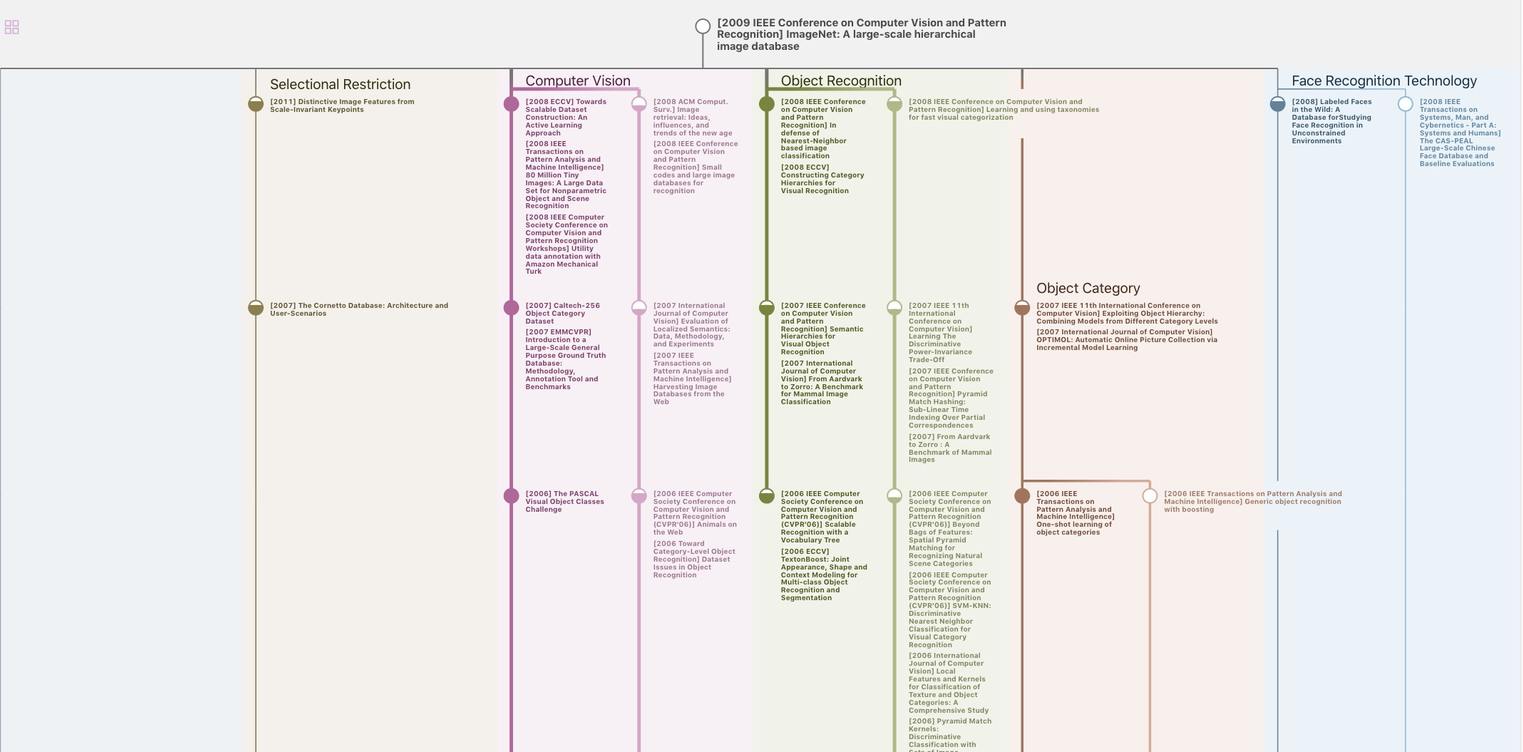
生成溯源树,研究论文发展脉络
Chat Paper
正在生成论文摘要