Discovering Prognostic Features Using Genetic Programming in Remaining Useful Life Prediction
IEEE Transactions on Industrial Electronics(2014)
摘要
In prognostics approaches, features (e.g., vibration level, root mean square or outputs from signal processing techniques) extracted from the measurement (e.g., vibration, current, and pressure, etc.) are often used or modeled as an indicator to the equipment's health condition. When faults are detected or when increasing/decreasing trends are shown in the health indicator, prediction algorithms are applied to extrapolate the future behavior and predict remaining useful life (RUL). However, it is difficult to make an accurate prediction if the trend of the health indicator is not obvious through the entire life cycle or if the trend is only shown right before a failure occurs. The challenge lies in whether an advanced feature (e.g., a mathematical combination of a group of the extracted features) can be found to clearly present/correlate with the fault progression. A genetic programming method is proposed to address the challenge of automatically discovering advanced feature(s), which can well capture the fault progression, from the measurement or extracted features in the purpose of RUL prediction.
更多查看译文
关键词
prediction algorithms,predict remaining useful life,prognostics,equipments health condition,genetic programming method,genetic programming (gp),health indicator,failure analysis,measurement,remaining useful life (rul),indicators,genetic algorithms,prediction theory,condition monitoring,rul,remaining useful life prediction,feature selection,remaining life assessment,faults detection,prognostic approaches
AI 理解论文
溯源树
样例
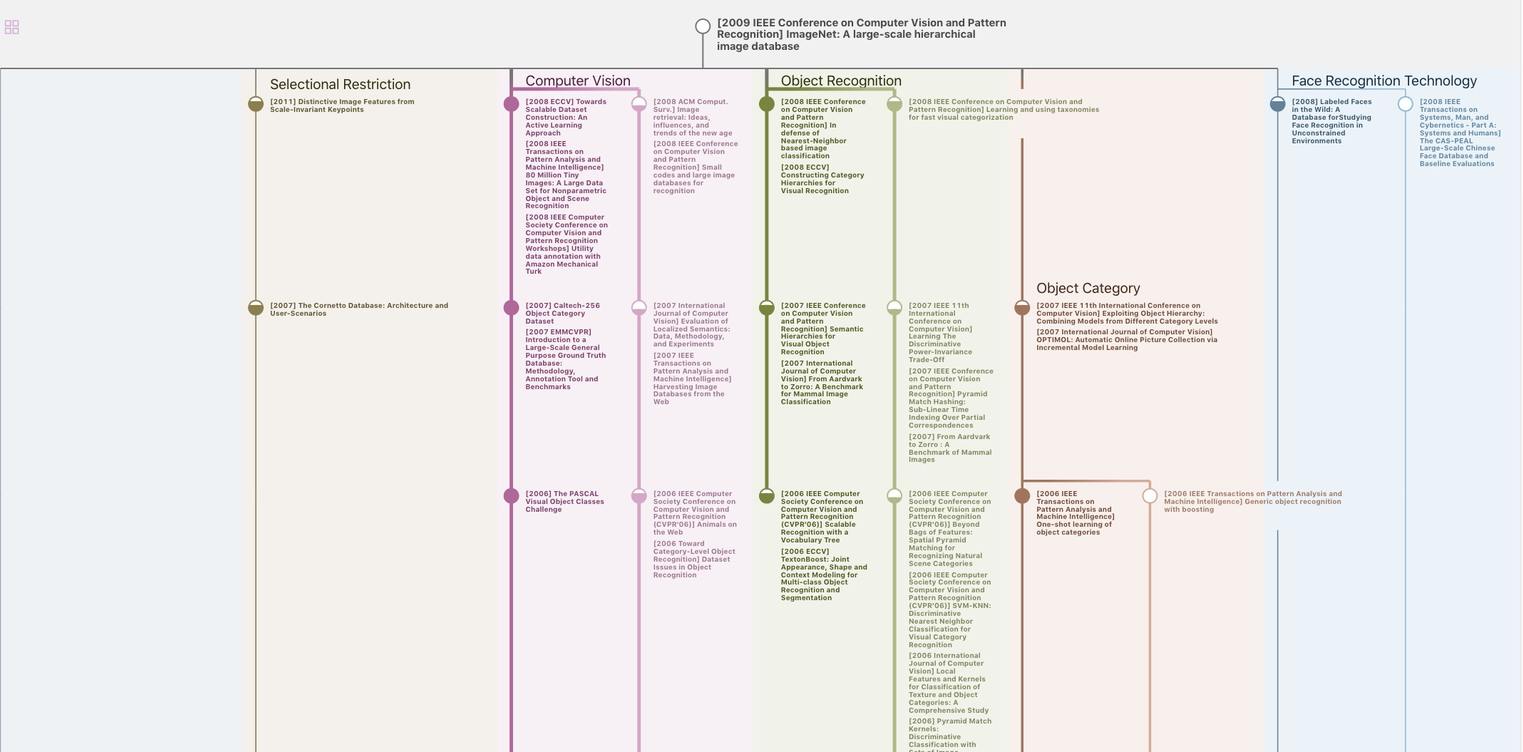
生成溯源树,研究论文发展脉络
Chat Paper
正在生成论文摘要