Coherence between surface electromyograms is influenced by electrode placement in hand muscles
Journal of Neuroscience Methods(2011)
摘要
We used multi-channel surface electromyograms (EMGs) to examine if electrode location influences coherence measures derived from pairs of EMGs recorded from two hand muscles during a pinch task. A linear probe of 16 electrodes was used to estimate the location of the innervation zone in first dorsal interosseous (FDI) and abductor pollicis brevis (APB). Four electrodes were then placed on the skin overlying each muscle and three bipolar electrode configurations were constructed with their center points directly over the innervation zone, and 15 mm distal and proximal to the innervation zone. Ten subjects performed two force-matching tasks for 120 s at 2 N and 3.5 N by pressing a force sensor held between the thumb and index finger. Coherence spectra were calculated from pairs of EMGs recorded from the two muscles. Maximal coherence from 1 to 15 Hz and 16 to 32 Hz was calculated at both force levels from the EMGs with electrodes centered over the innervation zones of FDI and APB. These values were compared to the maximal coherence from all other EMG comparisons across muscles recorded with electrodes that avoided the innervation zones. ANOVA revealed significant main effects only for electrode location, with a 58.1% increase ( p = 0.001) in maximal coherence for EMGs detected from pairs of electrodes that avoided the innervation zone (from 0.11 ± 0.02 to 0.18 ± 0.03; mean ± 95% confidence interval). These results indicate that electrode location relative to the innervation zone influences EMG–EMG coherence and should be carefully considered when placing EMG electrodes on hand muscles. Keywords EMG–EMG coherence Motor unit Common modulation Cross-correlation Hand Pinch grip Synchronization 1 Introduction Coherent oscillations in the synaptic inputs to motor neurons are thought to result in the periodic modulation of motor unit discharge rates (i.e., motor unit coherence), though the functional significance of the rhythmic activity to motor control is not clear ( Baker et al., 1999; Kilner et al., 2003; Semmler, 2002 ). One potential confound to determining the functional significance of the rhythmic activity is related to the technique commonly used to identify the rhythmic activity. Specifically, coherence analysis between two signals is routinely used in neurophysiology to estimate the magnitude of the linear correlation between specific frequency components in the two signals ( Amjad et al., 1997; Christakos, 1997; Halliday et al., 1995 ). Although coherence measures in humans are most commonly derived from the discharge times of pairs of simultaneously active motor units ( Farmer et al., 1993; Kakuda et al., 1999; Semmler et al., 2003 ), this may be problematic as pair-wise correlations between the discharge times of two neurons may not accurately reflect correlated activity across entire populations of neurons ( Schneidman et al., 2006 ). More recently, coherence measures derived from pairs of surface electromyograms (EMGs) recorded over synergist muscles (i.e., EMG–EMG coherence) have been used and are thought to provide a more representative measure of rhythmic activity across muscles ( Baker et al., 1999; Farmer et al., 2007; Kilner et al., 1999 ). Two primary lines of evidence support the use of EMG–EMG coherence as a population measure of rhythmic activity. The first line of support is mathematically derived ( Amjad et al., 1997; Halliday et al., 1995; Myers et al., 2003; Stegeman et al., 2010; Williams and Baker, 2009 ), including modeling approaches that suggest that the surface EMG can be used to reflect the common modulation in the discharge rates of motor units within and across muscles. The second line of support is derived from the positive associations demonstrated experimentally between measures of coherence calculated from different neurophysiologic signals, including EMGs, electroencephalograms (EEGs), magnetoencephalograms (MEGs), and cortical neuron and motor unit discharge times ( Baker et al., 1999; Halliday et al., 1998; Hansen et al., 2002; Kilner et al., 1999 ). For example, Baker et al. (1999) found that coherence between cortical neurons and EMGs was modulated in a task-dependent manner from 20 to 30 Hz in primates performing precise hand tasks and that EMG–EMG coherence from intrinsic hand and forearm muscles was modulated similarly in humans performing the same task. Nonetheless, the surface EMG can be influenced by many different factors independent of neuromuscular activity ( Farina et al., 2004b; Keenan et al., 2007 ) which will influence its sensitivity as a measure of motor unit activity. Although previous work has focused on how signal processing (e.g., rectification) may influence EMG-derived measures of coherence ( Myers et al., 2003; Neto and Christou, 2010; Stegeman et al., 2010; Yao et al., 2007 ), the influence of electrode location on coherence measures has not been systematically addressed. In contrast to a number of studies that highlight the importance of avoiding the innervation zone when placing EMG electrodes ( Beck et al., 2008; Merletti et al., 2003; Rainoldi et al., 2004; Roy et al., 1986 ), many studies report placement of electrodes on the muscle belly where the innervation zone is likely to be located (see review: Mesin et al., 2009 ). This problem is likely compounded in hand muscles, where short fiber lengths and an innervation zone usually located in the middle of the muscle ( Keenan et al., 2005; Saitou et al., 2000 ) make it difficult to avoid the innervation zone when placing surface electrodes. The development of multi-channel EMG systems has allowed non-invasive methods to estimate the location of the innervation zone ( Lapatki et al., 2006; Mesin et al., 2009; Saitou et al., 2000 ). The purpose of this study was to use multi-channel surface EMGs to examine if electrode location relative to the estimated location of the innervation zone influences EMG–EMG coherence measures during a pinch-grip task. 2 Materials and methods 2.1 Subjects Ten healthy, young adults (5 women, 5 men; 24.4 ± 1.3 years (mean ± SD); range: 23–27 years old) with no known neuromuscular disorders volunteered to participate in this study. All subjects were right-hand dominant as assessed by the Edinburgh Handedness Inventory ( Oldfield, 1971 ). The Institutional Review Board at the University of Wisconsin-Milwaukee approved all procedures and subjects gave their written formal consent before participating in the study. 2.2 Experimental procedure We first identified the innervation zone and muscle fiber direction using multi-channel surface EMG (EMG-USB; OT Bioelettronica, Torino, Italy). Surface EMG signals were recorded from first dorsal interosseous (FDI) and abductor pollicis brevis (APB) muscles of the non-dominant left hand. A reference electrode was placed on the left wrist and the subject's skin was prepared using abrasive paste (Spes Medica, Italy). A probe consisting of 16 silver electrodes (2.5-mm inter-electrode distance) (SA16/5, OT Bioelettronica, Torino, Italy) was used to identify the innervation zone by visual analysis of the 15 bipolar EMG signals generated from consecutive electrodes during a series of test contractions of the FDI and APB muscles ( Fig. 1 A ). As commonly done ( Merletti et al., 2003 ), the probe was positioned over each muscle and the orientation of the probe was adjusted to clearly identify propagation of the motor unit action potentials in both directions from the innervation zone ( Fig. 1 B). The innervation zone was then estimated as the EMG signal that was closest to the location where motor unit action potentials started to propagate in both directions and a clear change in the polarity of the phases of the potential was present ( Mesin et al., 2009; Saitou et al., 2000 ); this location was marked on the skin over both muscles ( Fig. 1 A and C). The direction of the probe was also marked on the skin to estimate muscle fiber direction ( Fig. 1 A and C). After estimating the location of the innervation zone, four surface EMG electrodes (4-mm diameter, silver–silver chloride; 15 mm inter-electrode distance) were placed on the skin overlying both the FDI and the APB muscles (shown only for APB in Fig. 1 C). Three bipolar EMG recording configurations were constructed from consecutive pairs of electrodes placed in line with muscle fiber direction. Two electrodes ( Fig. 1 C, electrodes 2 and 3) were placed with their center point over the innervation zone. The other two electrode configurations had their center points positioned 15 mm distal ( Fig. 1 C, electrodes 1 and 2) and proximal ( Fig. 1 C, electrodes 3 and 4) to the innervation zone. A common ground electrode (4-mm diameter, silver–silver chloride) was placed on the head of the ulna on the dorsal surface of the hand. The surface EMG signals were amplified (1 K) and band-pass filtered (13–1 kHz) using an isolated bio-amplifier (Coulbourn Instruments). EMG signal quality was checked before and after each experiment by checking the baseline level of noise at rest, as well as having subjects perform a brief 3–5 s maximal contraction of each muscle (index finger abduction for FDI and thumb abduction for APB) while the experimenter provided manual resistance ( Keenan et al., 2009 ). The subjects were seated on a chair with the left arm resting on a vacuum foam pad (VersaForm pillow, Tumble Forms). We asked participants to perform force matching tasks for 120 s at 2.0 and 3.5 N while holding a uniaxial force sensor (model ELFS-B3-10; Entran) between the thumb-tip and radial side of the distal phalanx of the index finger (i.e., key pinch). These low force levels were chosen to replicate previous work using pinch grip tasks ( Kilner et al., 1999 ). Subjects were asked to slightly extend the other three fingers not involved in the task so that they could not assist the index finger during the task. Two round metal caps (18 mm diameter) were covered with fine sandpaper (180 grit) and securely fastened onto both sides of the force sensor to provide a rigid, high-friction interface between the fingers and the sensor. To provide visual feedback of force, subjects were seated and facing a 24-in LCD monitor located 1.25 m away. The target force was displayed as a horizontal dashed line located in the middle of the screen, and the force produced by the subject during the 120 s trial was displayed as a horizontal solid line. Subjects were instructed to match their actual force with the target force as closely as possible. Due to hardware limitations related to significant cable noise in the Coulbourn bio-amplifiers when recording six EMG signals simultaneously, two force matching trials were performed at each force level. One trial involved recording two bipolar EMGs from the proximal and distal pairs of electrodes from each muscle (i.e., from electrode pairs that did not overlap the innervation zone of each muscle). The other trial involved an identical force matching task that was performed with the electrode connections reconfigured to record from only the pair of electrodes centered over the innervation zone of each muscle. The order of the two force-matching trials and force levels was randomized across subjects. 2.3 Analysis The procedures for calculation of coherence between two signals have been described in detail in previous publications ( Amjad et al., 1997; Halliday et al., 1995 ). Briefly, spectral analysis of the EMG signals was performed using custom scripts written in Matlab and based predominantly on software by Neurospec ( www.neurospec.org ) ( Halliday et al., 1995 ). As EMG–EMG coherence during hand pressing tasks is reported to be in specific frequency bins ( Baker et al., 1999; Farmer et al., 2007; Kilner et al., 1999 ), maximal EMG–EMG coherence for each force trial was calculated within the frequencies from 1 to 15 Hz and 16 to 32 Hz. For the main comparison of interest in the current study, coherence was calculated between rectified EMGs detected with electrodes centered over the innervation zones of FDI and APB, and this value was compared to the maximal EMG–EMG coherence from all other EMG comparisons across muscles (i.e., total of four other comparisons from EMG pairs with electrodes positioned away from the innervation zone). We also examined if the frequency where coherence reached a maximum was significantly different across conditions. Repeated measures ANOVA was used to test differences in maximal coherence and peak frequency between electrode location (i.e., electrodes centered over or away from the innervation zone), force level (2 or 3.5 N), and frequency band (1–15 or 16–32 Hz). As commonly done ( Amjad et al., 1997; Kilner et al., 1999 ), a Fisher transformation was applied to coherence values before performing ANOVA, and then this quantity was transferred back to the domain of coherence and is reported in the text and figures. Statistical significance was set at p < 0.05. The significance level for coherence functions was computed with α = 0.05. Results are presented as mean ± 95% confidence intervals (CI) in the text and in Fig. 2 . 3 Results Fig. 1 shows representative data demonstrating the change in coherence when recording EMG signals that are detected with electrodes centered over the innervation zone or away from the innervation zone. Specifically, in Fig. 1 D, pairs of EMGs were recorded from FDI and APB with the electrodes centered either over the innervation zone (electrodes 2 and 3) or away from the innervation zone (electrodes 1 and 2). Coherence spectra calculated from each pair of signals are shown in Fig. 1 E. Coherence increased across the frequencies of interest (1–15 and 16–32 Hz) when EMGs were detected with electrodes placed away from the innervation zone (in Fig. 1 E, compare thick black line with dotted black line; 95% confidence interval is represented by dashed horizontal line). In all subjects the coherence level was significant (i.e., above the 95% confidence interval) when EMGs were detected with electrodes placed away from the innervation zone. When computed from EMGs with electrodes centered over the innervation zone, EMG–EMG coherence was significant in all subjects from 16 to 32 Hz, and in 9 of 10 subjects from 1 to 15 Hz. Fig. 2 shows the magnitude of coherence (mean ± CI, n = 10) for each experimental condition. As there were no significant differences across force level ( p = 0.488) or frequency range ( p = 0.129), data are reported below collapsed across electrode locations. Coherence increased ( p = 0.001) from 0.11 ± 0.02 to 0.18 ± 0.03 (mean ± CI) when calculated from EMGs that were detected with electrodes centered over the innervation zone relative to EMGs from electrodes placed away from the innervation zone. This represents a 58.1% increase in coherence values with a 1.5 cm shift in electrode placement. In addition, the frequency at which the peak in coherence was found was not significantly different for any of the three experimental conditions ( p > 4.97). For example, at the 2 N force level, the frequency of peak coherence for the low and high frequency range was 8.2 ± 1.1 Hz and 24.6 ± 1.0 Hz, respectively. 4 Discussion The results indicate that coherence values derived from surface EMGs are sensitive to electrode location with respect to the innervation zone. Specifically, a change in electrode location of 1.5 cm away from the center of the innervation zone resulted in a 58.1% increase in the level of EMG–EMG coherence between APB and FDI during a pinch task ( Fig. 2 ). In contrast, the change in electrode location did not influence the frequency where the peak in coherence was observed. The implications of these findings on EMG–EMG coherence, especially in hand motor control studies where this measure is frequently used, are discussed below. The placement of bipolar electrodes on the belly of a muscle, where the innervation zone is likely to be located, is still the most common recording configuration (see review: Mesin et al., 2009 ), although a number of studies highlight the importance of not placing electrodes in this location ( Beck et al., 2008; Merletti et al., 2003; Rainoldi et al., 2004; Roy et al., 1986 ). There are at least two potential problems with placing EMG electrodes over the innervation zone that could have resulted in the decreased EMG–EMG coherence observed in the current study. First, the amplitude of the EMG signal detected with a pair of electrodes placed over a muscle often has a minimum when detected with electrodes placed over the innervation zone ( Beck et al., 2008; Rainoldi et al., 2004 ). Second, spectral estimates of the frequency content of a signal can be altered by electrode location relative to the innervation zone ( Mesin et al., 2009; Roy et al., 1986 ), which may be problematic for coherence analysis which involves cross-correlation between EMG signals in the frequency domain. The current study builds on the existing literature and suggests another potential confound related to electrode location when detecting EMG–EMG coherence. EMG–EMG coherence changed substantially with small changes (i.e., 1.5 cm) in electrode placement, and the high sensitivity of coherence to electrode location in the current study has a number of practical implications for EMG–EMG coherence studies. One issue is that experimental recordings with bipolar electrodes over hand muscles are necessarily placed on the muscle belly due to the small size of the muscles, and therefore they most likely overlap the innervation zone. Thus, trying to avoid the innervation zone is not a trivial task when placing electrodes on hand muscles. Although there are detailed maps of anatomical locations (i.e., innervation zones and tendon endings) that are routinely used to guide EMG electrode placement (e.g., see Rainoldi et al., 2004 ), not all muscles are uniform in the arrangement of their innervation zones. For example, although FDI has an innervation zone that is located with little variability at nearly 40% of the length of the muscle distal to the proximal attachment of the muscle to the first metacarpal bone ( Keenan et al., 2005; Saitou et al., 2000 ), the innervation zone can be quite variable in other muscles (e.g., APB ( Saitou et al., 2000 ) and rectus femoris ( Rainoldi et al., 2004 )). This variability within and across subjects presents practical limitations when trying to avoid the innervation zone when using anatomical maps to guide electrode placements. Alternatively, the development and availability of multi-channel EMG systems to estimate the location of the innervation zone has increased, and this current study supports its use in the context of obtaining EMG–EMG coherence values, especially from hand muscles. However, the use of multi-channel EMG systems is still far from standard practice and may be cost-prohibitive for some users. Another potential issue is that there exist experimental conditions where proper electrode placement is likely not sufficient to avoid the influence of electrode location on EMG–EMG coherence. Specifically, the location of the innervation zone can change during both isometric and anisometric contractions ( Mesin et al., 2009; Piitulainen et al., 2009 ), which could result in unpredictable changes in EMG–EMG coherence that are unrelated to a change in rhythmic drive to the muscle. For example, during piloting for the current study, it was observed that the estimated location of the innervation zone could change by over a centimeter dependent on force level during the isometric contractions, similar to other published reports ( Piitulainen et al., 2009 ). This is likely the result of having different motor units active at different force levels, as well as the possibility of changes in muscle fiber length during the isometric contractions at different force levels. To address this confound in the current study, the test contractions that were performed to identify the innervation zone were done at force levels similar to those used during the force-matching tasks. In addition, previous work has demonstrated that the innervation zone will shift during anisometric contractions ( Mesin et al., 2009 ), which may correspondingly influence EMG–EMG coherence values. Due to the high sensitivity of EMG–EMG coherence to electrode placement relative to the innervation zone, it is possible that changes in innervation zone location and variability within and across muscles in its location could influence the values derived from coherence analysis. This is problematic because EMG–EMG coherence may change across conditions due to peripheral factors that influence the EMG, which are unrelated to the rhythmic drive to the muscles involved in the task. An underlying assumption of the technique of EMG–EMG coherence is that the EMG signal accurately reflects the neural drive to a muscle and is sensitive to detect the common modulation of motor unit activity in the muscle. While in general an EMG signal detects motor unit population activity, there are many different factors that can influence the EMG signal and correspondingly any variable derived from the EMG ( Farina et al., 2004b ). For example, EMG signals are influenced by motor unit action potential conduction velocity, muscle size, excitation level, and subcutaneous tissue thickness, which can influence measures derived from surface EMGs, including motor-unit synchronization ( Keenan et al., 2007; Kleine et al., 2001; Zhou and Rymer, 2004 ), evoked potentials ( Farina et al., 2004a; Keenan et al., 2006; van Dijk et al., 1999 ), and spectral and amplitude estimates ( Basmajian and De Luca, 1985; Farina et al., 2004b; Keenan et al., 2005 ). As the measure of EMG–EMG coherence relies on the ability of the EMG to accurately reflect motor unit activity, it is critical to consider all the potential factors that may influence the EMG when interpreting the results of EMG–EMG coherence. In the current study, the location of the EMG electrodes relative to the innervation zone is one such important condition that influences EMG–EMG coherence. References Amjad et al., 1997 A.M. Amjad D.M. Halliday J.R. Rosenberg B.A. Conway An extended difference of coherence test for comparing and combining several independent coherence estimates: theory and application to the study of motor units and physiological tremor J Neurosci Methods 73 1997 69 79 Baker et al., 1999 S.N. Baker J.M. Kilner E.M. Pinches R.N. Lemon The role of synchrony and oscillations in the motor output Exp Brain Res 128 1999 109 117 Basmajian and De Luca, 1985 J.V. Basmajian C.J. De Luca Muscles alive: their functions revealed by electromyography 5th ed. 1985 Williams & Wilkins Baltimore Beck et al., 2008 T.W. Beck T.J. Housh J.T. Cramer M. Mielke R. Hendrix The influence of electrode shift over the innervation zone and normalization on the electromyographic amplitude and mean power frequency versus isometric torque relationships for the vastus medialis muscle J Neurosci Methods 169 2008 100 108 Christakos, 1997 C.N. Christakos On the detection and measurement of synchrony in neural populations by coherence analysis J Neurophysiol 78 1997 3453 3459 Farina et al., 2004a D. Farina A. Blanchietti M. Pozzo R. Merletti M-wave properties during progressive motor unit activation by transcutaneous stimulation J Appl Physiol 97 2004 545 555 Farina et al., 2004b D. Farina R. Merletti R.M. Enoka The extraction of neural strategies from the surface EMG J Appl Physiol 96 2004 1486 1495 Farmer et al., 1993 S.F. Farmer F.D. Bremner D.M. Halliday J.R. Rosenberg J.A. Stephens The frequency content of common synaptic inputs to motoneurones studied during voluntary isometric contraction in man J Physiol 470 1993 127 155 Farmer et al., 2007 S.F. Farmer J. Gibbs D.M. Halliday L.M. Harrison L.M. James M.J. Mayston Changes in EMG coherence between long and short thumb abductor muscles during human development J Physiol 579 2007 389 402 Halliday et al., 1998 D.M. Halliday B.A. Conway S.F. Farmer J.R. Rosenberg Using electroencephalography to study functional coupling between cortical activity and electromyograms during voluntary contractions in humans Neurosci Lett 241 1998 5 8 Halliday et al., 1995 D.M. Halliday J.R. Rosenberg A.M. Amjad P. Breeze B.A. Conway S.F. Farmer A framework for the analysis of mixed time series/point process data—theory and application to the study of physiological tremor, single motor unit discharges and electromyograms Prog Biophys Mol Biol 64 1995 237 278 Hansen et al., 2002 S. Hansen N.L. Hansen L.O. Christensen N.T. Petersen J.B. Nielsen Coupling of antagonistic ankle muscles during co-contraction in humans Exp Brain Res 146 2002 282 292 Kakuda et al., 1999 N. Kakuda M. Nagaoka J. Wessberg Common modulation of motor unit pairs during slow wrist movement in man J Physiol 520 Pt. 3 1999 929 940 Keenan et al., 2005 K.G. Keenan D. Farina K.S. Maluf R. Merletti R.M. Enoka Influence of amplitude cancellation on the simulated electromyogram J Appl Physiol 98 2005 120 131 Keenan et al., 2006 K.G. Keenan D. Farina R. Merletti R.M. Enoka Influence of motor unit properties on the size of the simulated evoked surface EMG potential Exp Brain Res 169 2006 37 49 Keenan et al., 2007 K.G. Keenan D. Farina F.G. Meyer R. Merletti R.M. Enoka Sensitivity of the cross-correlation between simulated surface EMGs for two muscles to detect motor unit synchronization J Appl Physiol 102 2007 1193 1201 Keenan et al., 2009 K.G. Keenan V.J. Santos M. Venkadesan F.J. Valero-Cuevas Maximal voluntary fingertip force production is not limited by movement speed in combined motion and force tasks J Neurosci 29 2009 8784 8789 Kilner et al., 1999 J.M. Kilner S.N. Baker S. Salenius V. Jousmaki R. Hari R.N. Lemon Task-dependent modulation of 15–30 Hz coherence between rectified EMGs from human hand and forearm muscles J Physiol 516 1999 559 570 Kilner et al., 2003 J.M. Kilner S. Salenius S.N. Baker A. Jackson R. Hari R.N. Lemon Task-dependent modulations of cortical oscillatory activity in human subjects during a bimanual precision grip task Neuroimage 18 2003 67 73 Kleine et al., 2001 B.U. Kleine D.F. Stegeman D. Mund C. Anders Influence of motoneuron firing synchronization on SEMG characteristics in dependence of electrode position J Appl Physiol 91 2001 1588 1599 Lapatki et al., 2006 B.G. Lapatki R. Oostenveld J.P. Van Dijk I.E. Jonas M.J. Zwarts D.F. Stegeman Topographical characteristics of motor units of the lower facial musculature revealed by means of high-density surface EMG J Neurophysiol 95 2006 342 354 Merletti et al., 2003 R. Merletti D. Farina M. Gazzoni The linear electrode array: a useful tool with many applications J Electromyogr Kinesiol 13 2003 37 47 Mesin et al., 2009 L. Mesin R. Merletti A. Rainoldi Surface EMG: the issue of electrode location J Electromyogr Kinesiol 19 2009 719 726 Myers et al., 2003 L.J. Myers M. Lowery M. O’Malley C.L. Vaughan C. Heneghan A. St Clair Gibson Rectification and non-linear pre-processing of EMG signals for cortico-muscular analysis J Neurosci Methods 124 2003 157 165 Neto and Christou, 2010 O.P. Neto E.A. Christou Rectification of the EMG signal impairs the identification of oscillatory input to the muscle J Neurophysiol 103 2010 1093 1103 Oldfield, 1971 R.C. Oldfield The assessment and analysis of handedness: the Edinburgh inventory Neuropsychologia 9 1971 97 113 Piitulainen et al., 2009 H. Piitulainen T. Rantalainen V. Linnamo P. Komi J. Avela Innervation zone shift at different levels of isometric contraction in the biceps brachii muscle J Electromyogr Kinesiol 19 2009 667 675 Rainoldi et al., 2004 A. Rainoldi G. Melchiorri I. Caruso A method for positioning electrodes during surface EMG recordings in lower limb muscles J Neurosci Methods 134 2004 37 43 Roy et al., 1986 S.H. Roy C.J. De Luca J. Schneider Effects of electrode location on myoelectric conduction velocity and median frequency estimates J Appl Physiol 61 1986 1510 1517 Saitou et al., 2000 K. Saitou T. Masuda D. Michikami R. Kojima M. Okada Innervation zones of the upper and lower limb muscles estimated by using multichannel surface EMG J Hum Ergol (Tokyo) 29 2000 35 52 Schneidman et al., 2006 E. Schneidman M.J. Berry 2nd R. Segev W. Bialek Weak pairwise correlations imply strongly correlated network states in a neural population Nature 440 2006 1007 1012 Semmler, 2002 J.G. Semmler Motor unit synchronization and neuromuscular performance Exerc Sport Sci Rev 30 2002 8 14 Semmler et al., 2003 J.G. Semmler K.W. Kornatz R.M. Enoka Motor-unit coherence during isometric contractions is greater in a hand muscle of older adults J Neurophysiol 90 2003 1346 1349 Stegeman et al., 2010 D.F. Stegeman W.J. van de Ven G.A. van Elswijk R. Oostenveld B.U. Kleine The alpha-motoneuron pool as transmitter of rhythmicities in cortical motor drive Clin Neurophysiol 2010 doi:10.1016/j.clinph.2010.03.052 van Dijk et al., 1999 J.G. van Dijk I. van Benten C.G. Kramer D.F. Stegeman CMAP amplitude cartography of muscles innervated by the median, ulnar, peroneal, and tibial nerves Muscle Nerve 22 1999 378 389 Williams and Baker, 2009 E.R. Williams S.N. Baker Circuits generating corticomuscular coherence investigated using a biophysically based computational model. I. Descending systems J Neurophysiol 101 2009 31 41 Yao et al., 2007 B. Yao S. Salenius G.H. Yue R.W. Brown J.Z. Liu Effects of surface EMG rectification on power and coherence analyses: an EEG and MEG study J Neurosci Methods 159 2007 215 223 Zhou and Rymer, 2004 P. Zhou W.Z. Rymer Factors governing the form of the relation between muscle force and the electromyogram (EMG): a simulation study J Neurophysiol 2004 2878 2886
更多查看译文
关键词
EMG–EMG coherence,Motor unit,Common modulation,Cross-correlation,Hand,Pinch grip,Synchronization
AI 理解论文
溯源树
样例
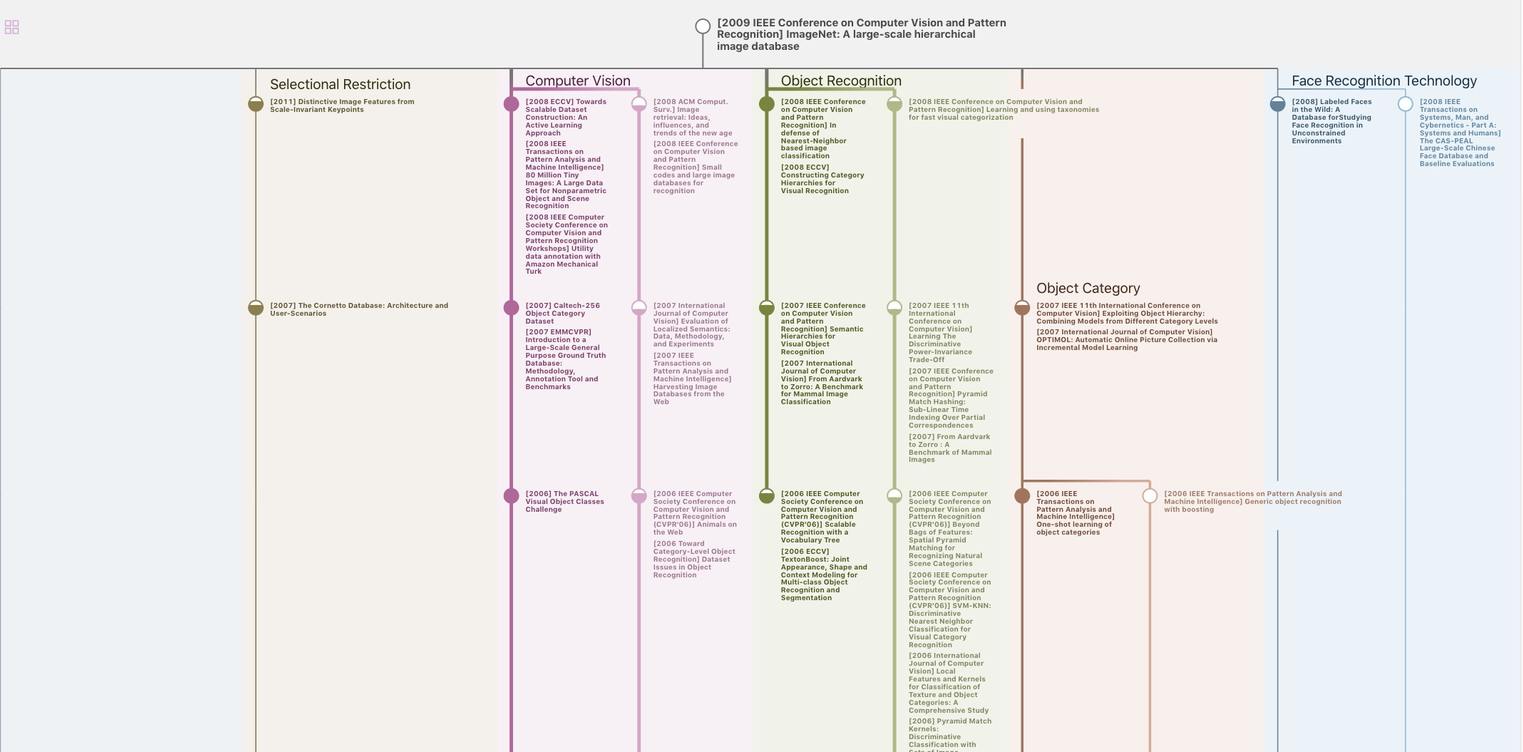
生成溯源树,研究论文发展脉络
Chat Paper
正在生成论文摘要