Voice conversion based on Gaussian mixture modules with Minimum Distance Spectral Mapping
2015 5th International Conference on Information Science and Technology (ICIST)(2015)
摘要
Voice conversion (VC) is the task of modifying a source speaker's voice to match that of a specific target speaker. Traditional methods use Gaussian mixture models (GMM), but the converted speech quality is often badly degraded due to over-smoothing. More recent approaches such as Dynamic Frequency Warping (DFW) maintain more spectrum details during transformation, but require specific formant frequency estimates, with estimation errors resulting in poor similarity between source and target speakers. This paper proposes a new method for voice conversion called Minimum Distance Spectral Mapping (MDSM), based on a frequency-warped point-to-point mapping that robustly and accurately transforms formant frequencies while also maintaining spectral details. The proposed MDSM method uses a minimum distance alignment between source and target speakers, rather than direct formant estimates, which increases robustness and also preserves other spectral details such as formant bandwidth. Results show that the proposed method offers a good trade-off between voice quality and identity similarity, outperforming traditional GMM and DFW in both subjective and objective evaluations.
更多查看译文
关键词
Voice Conversion,Gaussian mixture models,frequency warping,point-to-point mapping
AI 理解论文
溯源树
样例
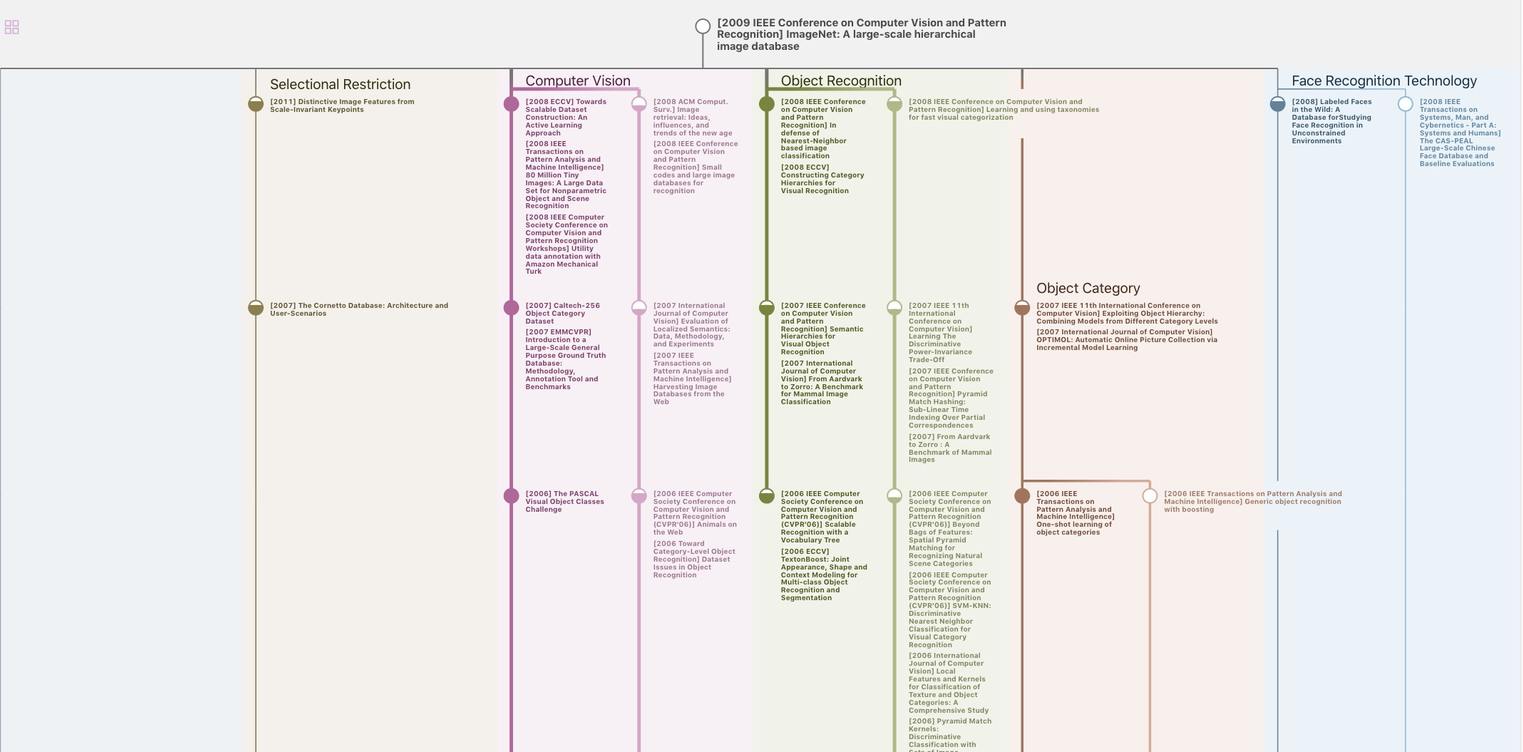
生成溯源树,研究论文发展脉络
Chat Paper
正在生成论文摘要