Information Theoretic Feature Selection And Projection
SPEECH, AUDIO, IMAGE AND BIOMEDICAL SIGNAL PROCESSING USING NEURAL NETWORKS(2008)
摘要
Pattern recognition systems are trained using a finite number of samples. Feature dimensionality reduction is essential to improve generalization and optimal exploitation of the information content in the feature vector. Dimensionality reduction eliminates redundant dimensions that do not convey reliable statistical information for classification, determines a manifold on which projections of the original high-dimensional feature vector exhibit maximal information about the class label, and reduces the complexity of the classifier to help avoid over-fitting. In this chapter we demonstrate computationally efficient algorithms for estimating and optimizing mutual information, specifically for the purpose of learning optimal feature dimensionality reduction solutions in the context of pattern recognition. These techniques and algorithms will be applied to the classification of multichannel EEG signals for brain computer interface design, as well as sonar imagery for target detection. Results will be compared with widely used benchmark alternatives such as LDA and kernel LDA.
更多查看译文
关键词
information content,mutual information,brain computer interface,feature vector,feature selection,pattern recognition
AI 理解论文
溯源树
样例
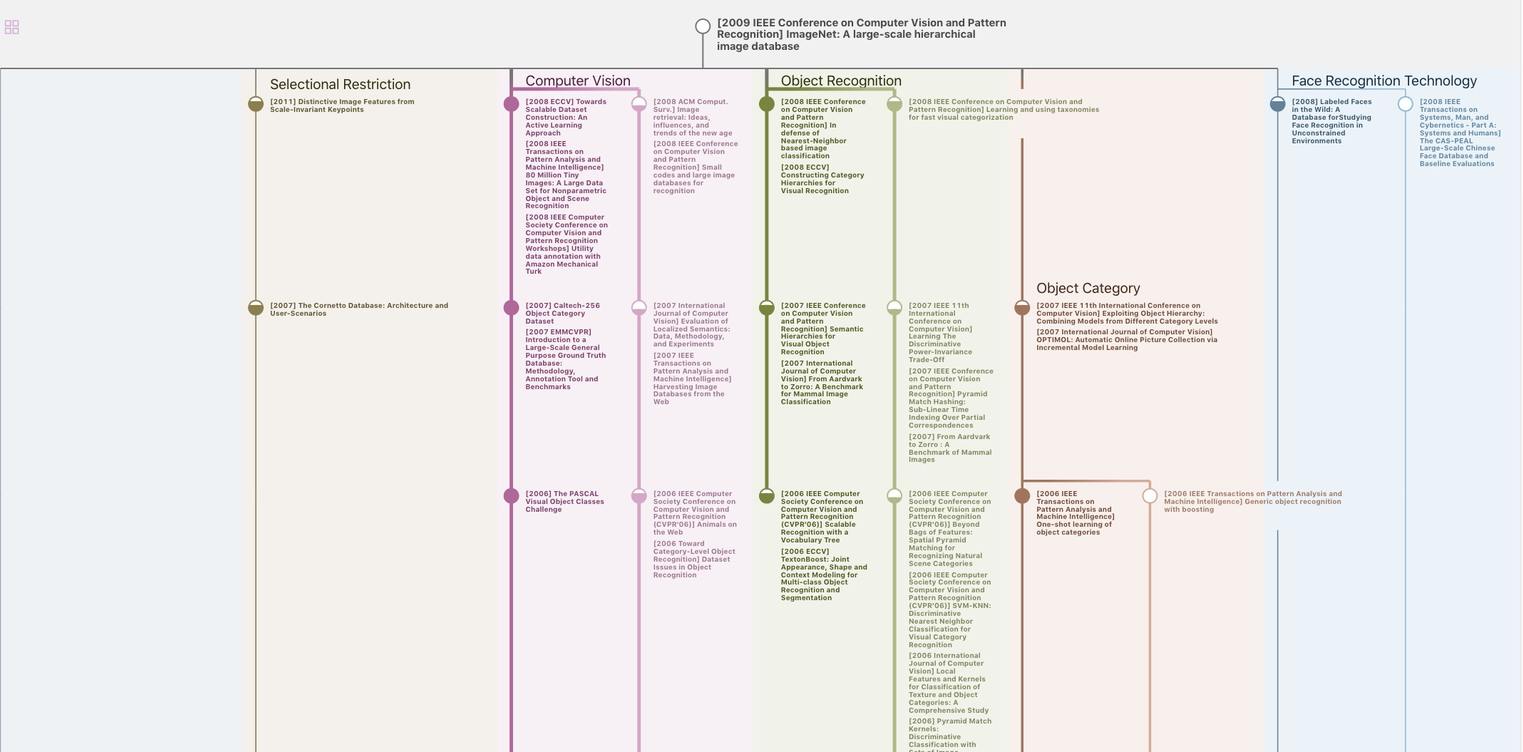
生成溯源树,研究论文发展脉络
Chat Paper
正在生成论文摘要