Extended Precision Quality Measure For Recommender Systems
CAEPIA'11: Proceedings of the 14th international conference on Advances in artificial intelligence: spanish association for artificial intelligence(2011)
摘要
Recommender systems are highly sensitive to cases of false-positives, that is, recommendations made which have proved not to be relevant. These situations often lead to a loss of trust in the system by the users; therefore, every improvement in the recommendation quality measures is important. Recommender systems which admit an extensive set of values in the votes (usually those which admit more than 5 stars to rate an item) cannot be assessed adequately using precision as a recommendation quality measure; this is due to the fact that the division of the possible values of the votes into just two sets, relevant (true-positive) and not-relevant (false-positive), proves to be too poor and involves the accumulation of values in the not-relevant set. In order to establish a balanced quality measure it is necessary to have access to detailed information on how the cases of false-positives are distributed. This paper provides the mathematical formalism which defines the precision quality measure in recommender systems and its generalization to extended-precision.
更多查看译文
关键词
Precision,quality measure,recommender systems,collaborative filtering
AI 理解论文
溯源树
样例
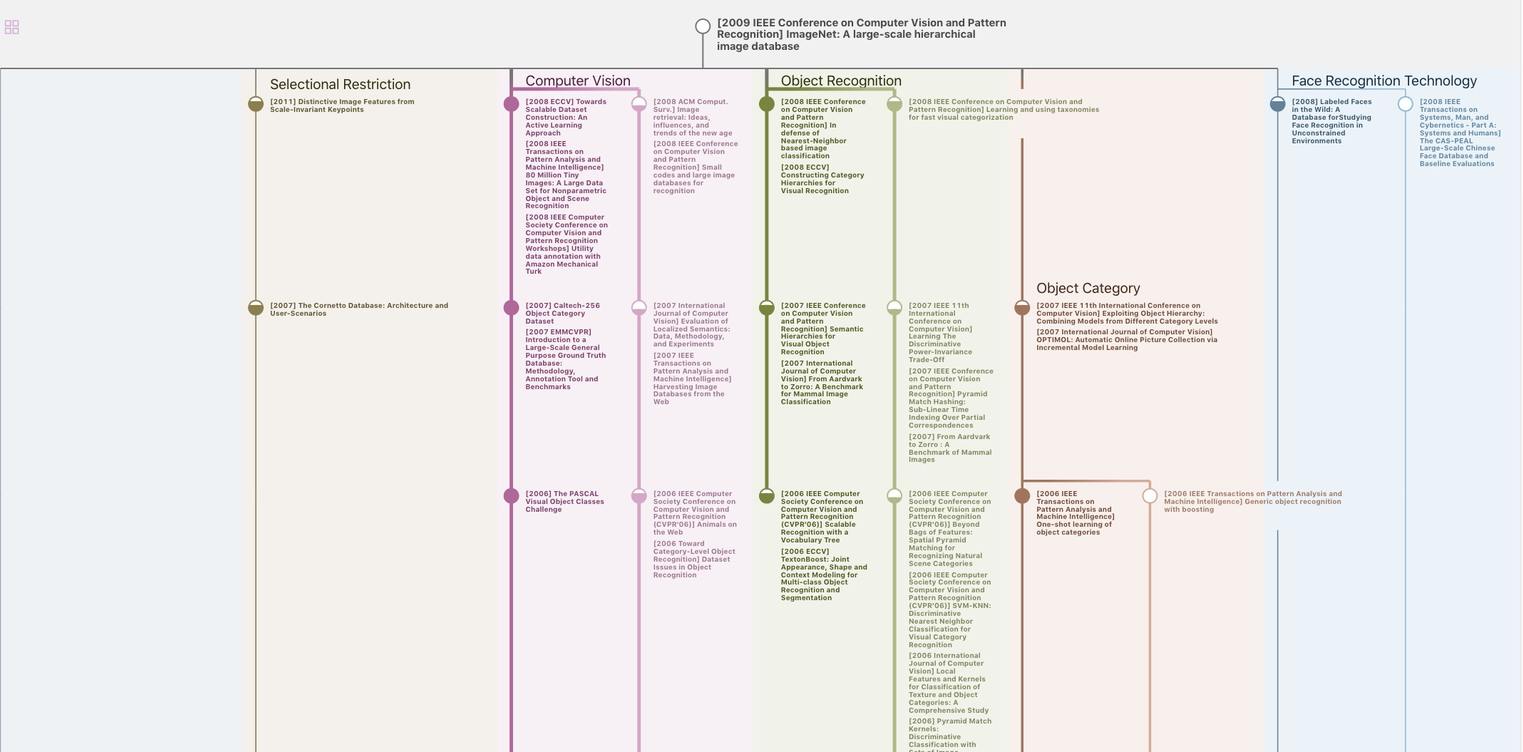
生成溯源树,研究论文发展脉络
Chat Paper
正在生成论文摘要