Difference-Based Estimates for Generalization-Aware Subgroup Discovery.
ECMLPKDD'13: Proceedings of the 2013th European Conference on Machine Learning and Knowledge Discovery in Databases - Volume Part III(2013)
摘要
For the task of subgroup discovery, generalization-aware interesting measures that are based not only on the statistics of the patterns itself, but also on the statistics of their generalizations have recently been shown to be essential. A key technique to increase runtime performance of subgroup discovery algorithms is the application of optimistic estimates to limit the search space size. These are upper bounds for the interestingness that any specialization of the currently evaluated pattern may have. Until now these estimates are based on the anti-monotonicity of instances, which are covered by the current pattern. This neglects important properties of generalizations. Therefore, we present in this paper a new scheme of deriving optimistic estimates for generalization aware subgroup discovery, which is based on the instances by which patterns differ in comparison to their generalizations. We show, how this technique can be applied for the most popular interestingness measures for binary as well as for numeric target concepts. The novel bounds are incorporated in an efficient algorithm, which outperforms previous methods by up to an order of magnitude.
更多查看译文
AI 理解论文
溯源树
样例
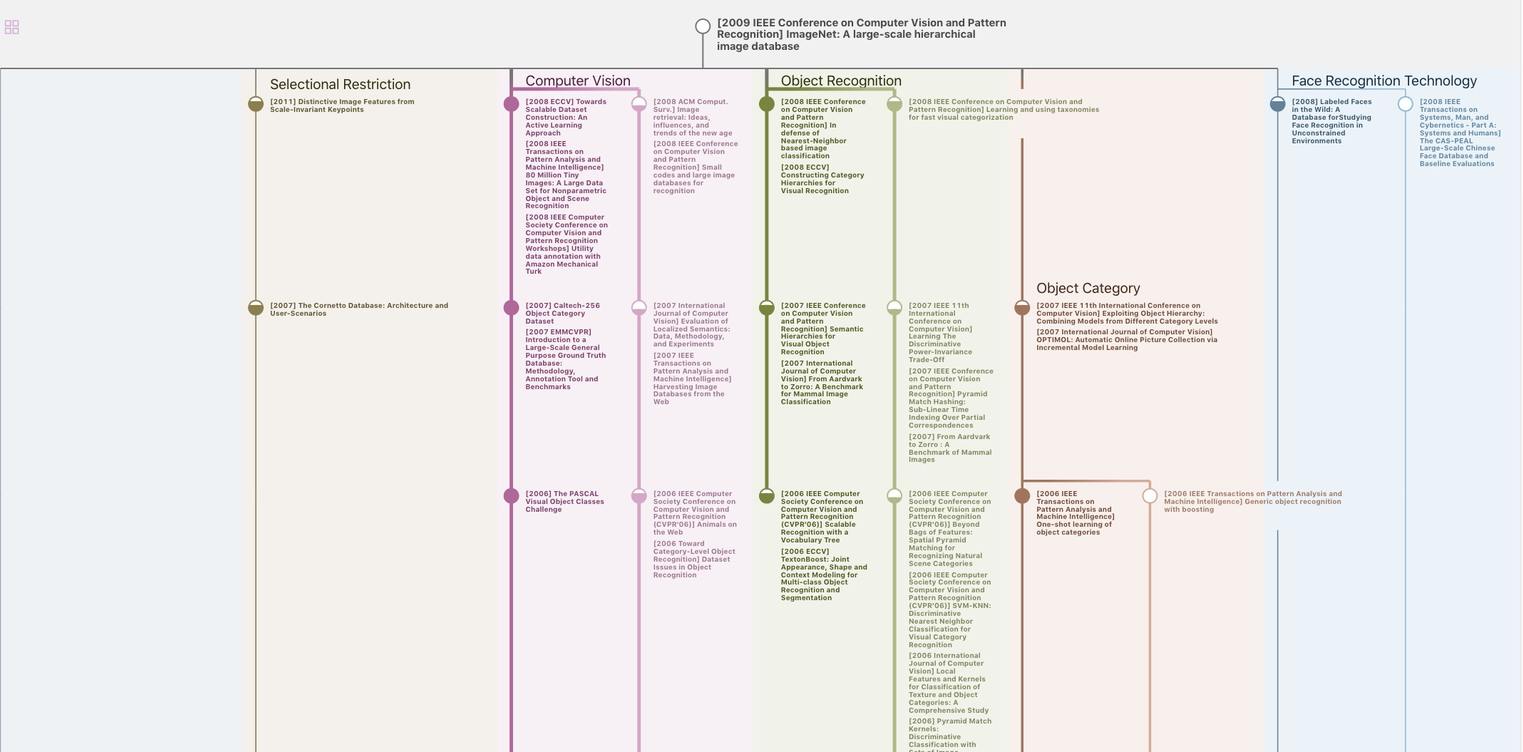
生成溯源树,研究论文发展脉络
Chat Paper
正在生成论文摘要