Convex Quadratic Programming for Slimming Convolutional Networks.
ICIP(2022)
摘要
To reduce the computational and memory cost of ConvNets, structured pruning consists in removing features in convolutional and/or fully-connected layers of a pretrained network. As a novel contribution, we study the selection of features as a convex quadratic program with linear constraints. In addition, the updating of weights is treated as the resolution of a linear least-squares system. The quadratic objective function arises from the least-squares reconstruction error on the outputs of pruned layers. Experiments show that our method gives accuracies on par with other reconstruction error-based methods, while achieving a clear gain on computation time.
更多查看译文
关键词
convex quadratic programming,quadratic programming,networks
AI 理解论文
溯源树
样例
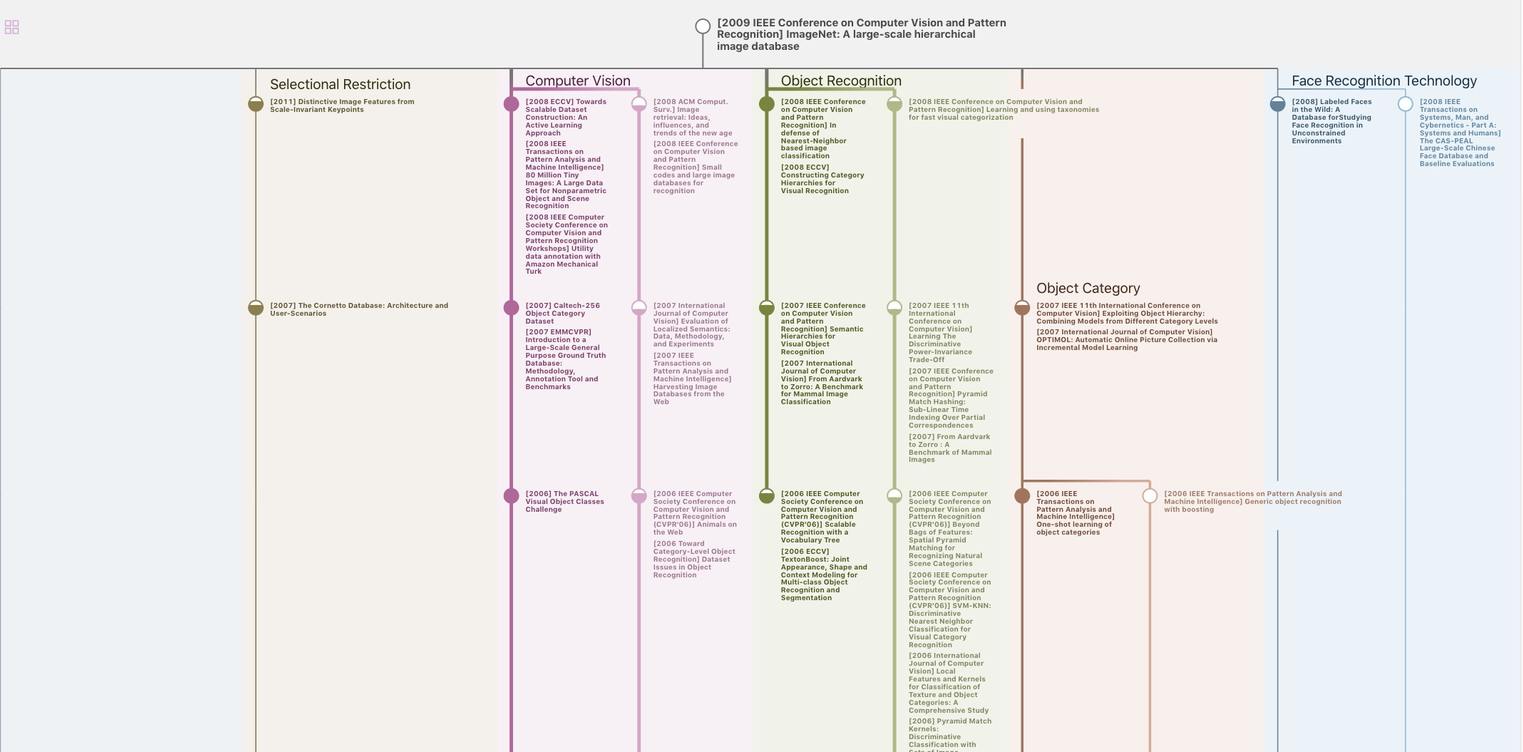
生成溯源树,研究论文发展脉络
Chat Paper
正在生成论文摘要