Detection of Deviations in Mobile Applications Network Behavior
CoRR(2012)
摘要
In this paper a novel system for detecting meaningful deviations in a mobile application's network behavior is proposed. The main goal of the proposed system is to protect mobile device users and cellular infrastructure companies from malicious applications. The new system is capable of: (1) identifying malicious attacks or masquerading applications installed on a mobile device, and (2) identifying republishing of popular applications injected with a malicious code. The detection is performed based on the application's network traffic patterns only. For each application two types of models are learned. The first model, local, represents the personal traffic pattern for each user using an application and is learned on the device. The second model, collaborative, represents traffic patterns of numerous users using an application and is learned on the system server. Machine-learning methods are used for learning and detection purposes. This paper focuses on methods utilized for local (i.e., on mobile device) learning and detection of deviations from the normal application's behavior. These methods were implemented and evaluated on Android devices. The evaluation experiments demonstrate that: (1) various applications have specific network traffic patterns and certain application categories can be distinguishable by their network patterns, (2) different levels of deviations from normal behavior can be detected accurately, and (3) local learning is feasible and has a low performance overhead on mobile devices.
更多查看译文
AI 理解论文
溯源树
样例
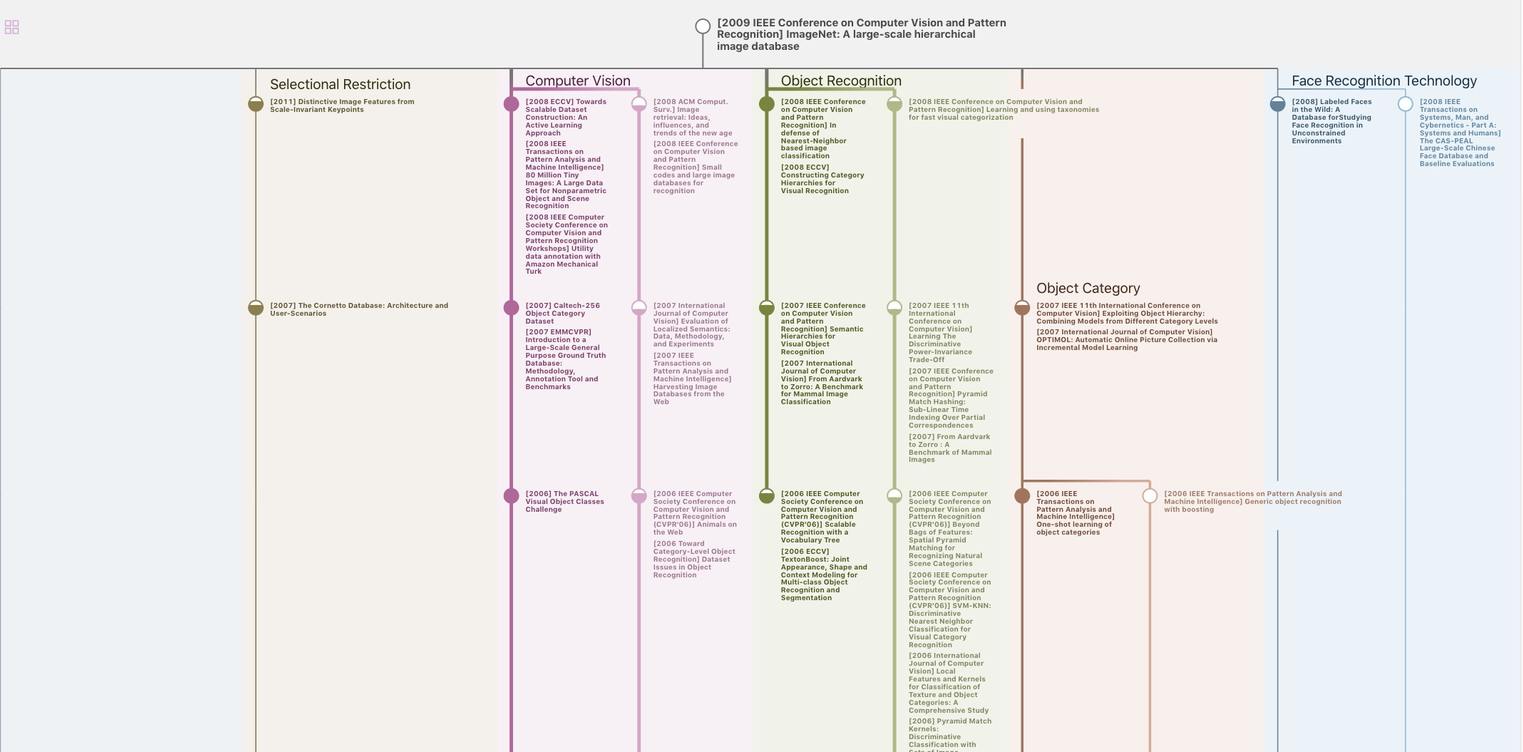
生成溯源树,研究论文发展脉络
Chat Paper
正在生成论文摘要