Semiparametric Latent Variable Models for Guided Representation
msra(2011)
摘要
Unsupervised discovery of latent representations, in addition to being useful
for density modeling, visualisation and exploratory data analysis, is also
increasingly important for learning features relevant to discriminative tasks.
Autoencoders, in particular, have proven to be an effective way to learn latent
codes that reflect meaningful variations in data. A continuing challenge,
however, is guiding an autoencoder toward representations that are useful for
particular discriminative tasks. A complementary challenge is to find codes
that are explicitly invariant to irrelevant transformations of the data. To
address these difficulties, we introduce the semiparametric latent variable
model (SPLVM), which combines an autoencoder with a Gaussian process latent
variable model. The SPLVM enables an autoencoder's unsupervised representation
to both incorporate relevant label information and ignore irrelevant
variations. We apply this model to several different datasets, examining how
both labels and nuisance variables can provide cues for useful latent
representations.
更多查看译文
关键词
gaussian process,latent variable model,exploratory data analysis
AI 理解论文
溯源树
样例
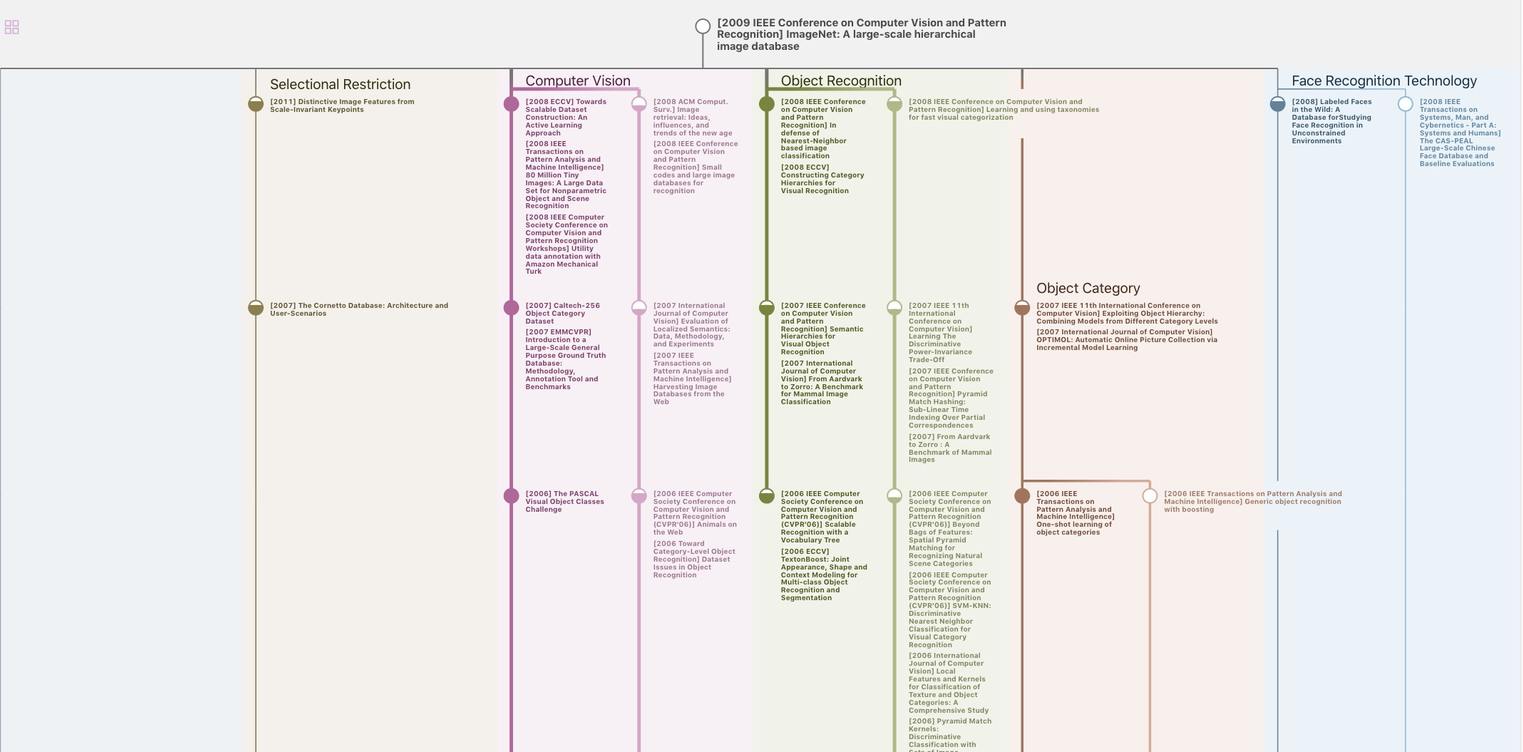
生成溯源树,研究论文发展脉络
Chat Paper
正在生成论文摘要