Generalizing to a zero-data task: a computational chemistry case study
msra(2006)
摘要
We investigate the problem of learning several tasks simultaneously in order to transfer the acquired knowledge to a completely new task for which no training data are available. Assuming that the tasks share some representation that we can discover eciently, such a scenario should lead to a better model of the new task, as compared to the model that is learned by only using the knowledge of the new task. We have evaluated several supervised learning algorithms in order to discover shared representations among the tasks defined in a computational chemistry/drug discovery problem. We have cast the problem from a statistical learning point of view and set up the general hypotheses that have to be tested in order to validate the multi-task learning approach. We have then evaluated the performance of the learning algorithms and showed that it is indeed possible to learn a shared representation of the tasks that allows to generalize to a new task for which no training data are available. From a theoretical point of view, our contribution also comprises a modification to the Support Vector Machine algorithm, which can produce state-of-the-art results using multi-task learning concepts at its core. From a practical point of view, our contribution is that this algorithm can be readily used by pharmaceutical companies for virtual screening campaigns.
更多查看译文
AI 理解论文
溯源树
样例
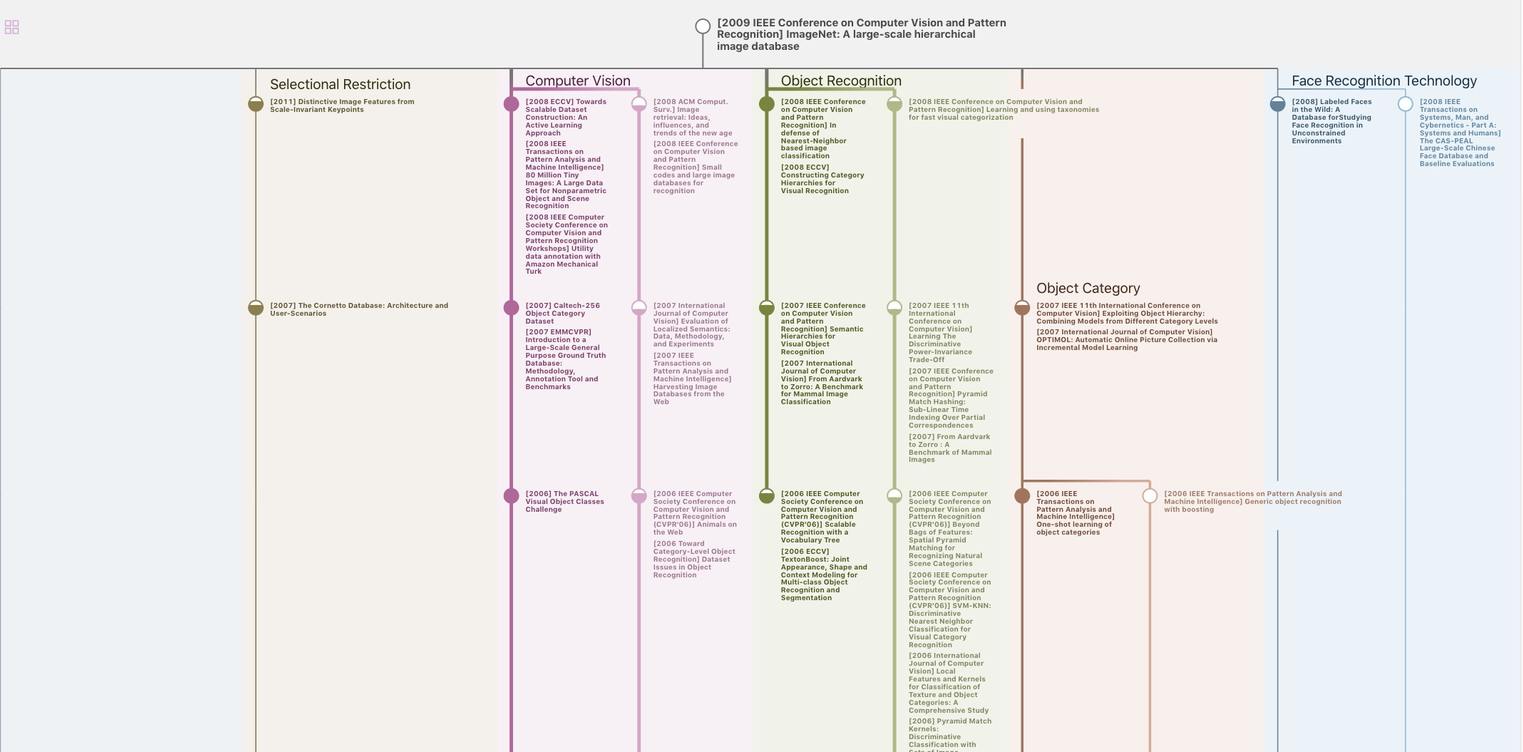
生成溯源树,研究论文发展脉络
Chat Paper
正在生成论文摘要