Comparisons Of Standard Curve-Fitting Methods To Quantitate Neisseria-Meningitidis Group-A Polysaccharide Antibody-Levels By Enzyme-Linked-Immunosorbent-Assay
msra(1991)
摘要
We examined several of the more commonly used models (log-log, two forms of the logit-log, and the four-parameter logistic-log transformations) for forming standard or calibration curves by using a standardized enzyme-linked immunosorbent assay (ELISA). Assay range, accuracy, and error for each function were measured and compared. Antibody levels to Neisseria meningitidis group A polysaccharide were estimated by calculating antibody concentrations of a serially diluted standard reference serum of known concentration. Each function achieved a high squared correlation coefficient (r2 > 0.97), indicating a high degree of accuracy in forming the standard curves. However, when predicted antibody concentrations were compared with the known values, the log-log function exhibited the least precision, with extreme precentages of error occurring at several dilutions. A partially specified logit-log transformation performed better than the log-log model over a reduced range of standard dilutions. This indicated that a high r2 alone was not a reliable measure of the accuracy of the standard curve. Of the methods surveyed, the logistic-log and fully specified logit-log functions were the most accurate models for forming standard curves and for interpolating antibody concentrations from the standard curve. The accuracy of the fully specified logit-log function is highly dependent on the precise specification of two unknown quantities, the optical densities at zero and infinite concentrations, prior to fitting the model to a typical set of calibration data. The four-parameter logistic-log function was the preferred choice for quantitating N. meningitidis group A total polysaccharide antibody by using a standardized ELISA. The function does not require prespecification of any parameters before estimating the standard curve, and the four parameters are readily interpretable in terms of identifiable physical quantities. This model also has the advantage that it is easiest to visualize since it does not incorporate complex transformations of the optical density scale.
更多查看译文
AI 理解论文
溯源树
样例
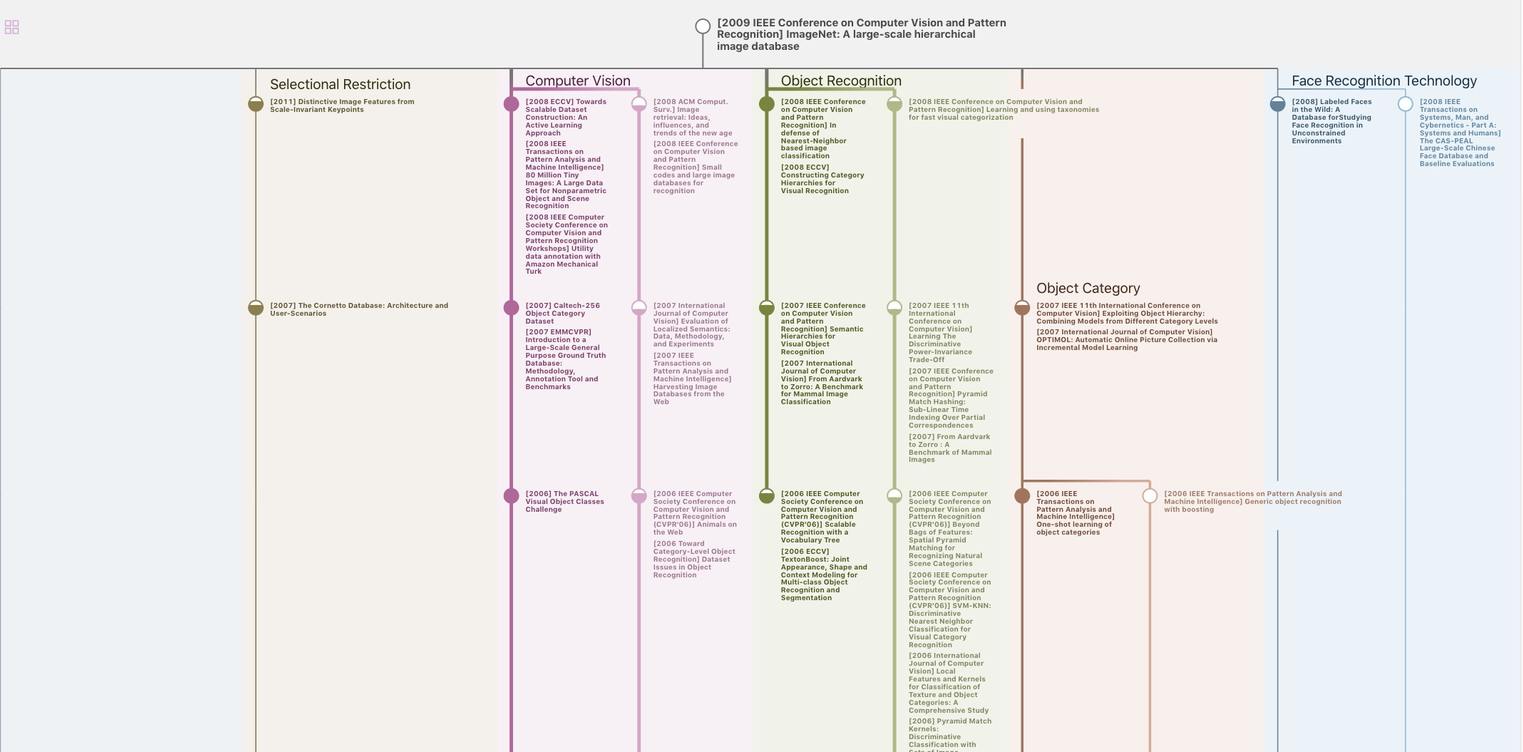
生成溯源树,研究论文发展脉络
Chat Paper
正在生成论文摘要