GP-Pi: Using Genetic Programming with Penalization and Initialization on Genome-Wide Association Study
ARTIFICIAL INTELLIGENCE AND SOFT COMPUTING, PT II(2013)
摘要
The advancement of chip-based technology has enabled the measurement of millions of DNA sequence variations across the human genome. Experiments revealed that high-order, but not individual, interactions of single nucleotide polymorphisms (SNPs) are responsible for complex diseases such as cancer. The challenge of genome-wide association studies (GWASs) is to sift through high-dimensional datasets to find out particular combinations of SNPs that are predictive of these diseases. Genetic Programming (GP) has been widely applied in GWASs. It serves two purposes: attribute selection and/or discriminative modeling. One advantage of discriminative modeling over attribute selection lies in interpretability. However, existing discriminative modeling algorithms do not scale up well with the increase in the SNP dimension. Here, we have developed GP-Pi. We have introduced a penalizing term in the fitness function to penalize trees with common SNPs and an initializer which utilizes expert knowledge to seed the population with good attributes. Experimental results on simulated data suggested that GP-Pi outperforms GPAS with statistically significance. GP-Pi was further evaluated on a real GWAS dataset of Rheumatoid Arthritis, obtained from the North American Rheumatoid Arthritis Consortium. Our results, with potential new discoveries, are found to be consistent with literature.
更多查看译文
关键词
Genome-Wide Association Study,Genetic Programming,Penalization,Initialization,Rheumatoid Arthritis
AI 理解论文
溯源树
样例
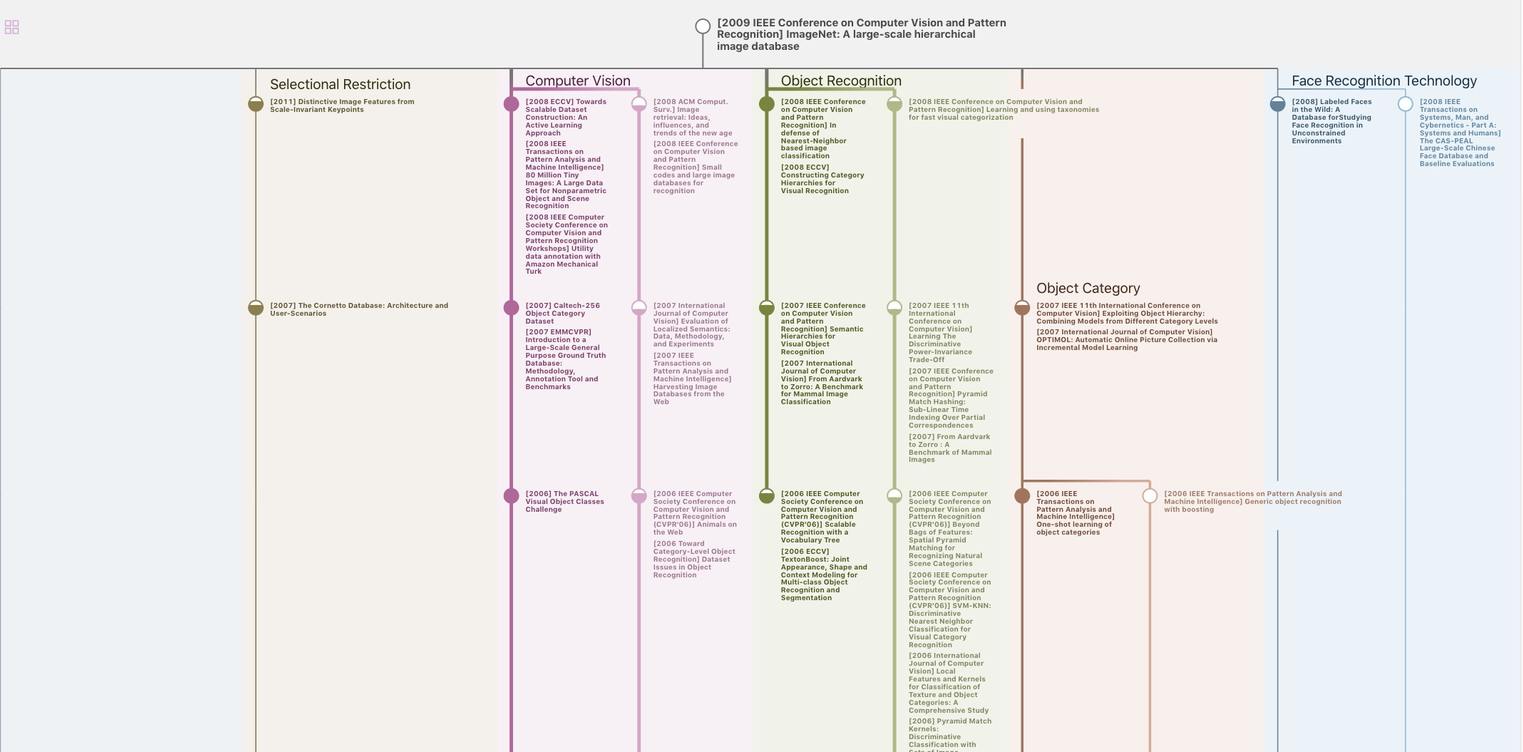
生成溯源树,研究论文发展脉络
Chat Paper
正在生成论文摘要