Recursive $\ell_{1,\infty}$ Group Lasso
IEEE Transactions on Signal Processing(2012)
摘要
We introduce a recursive adaptive group lasso algorithm for real-time penalized least squares prediction that produces a time sequence of optimal sparse predictor coefficient vectors. At each time index the proposed algorithm computes an exact update of the optimal $\ell_{1,\infty}$-penalized recursive least squares (RLS) predictor. Each update minimizes a convex but nondifferentiable function optimization problem. We develop an on-line homotopy method to reduce the computational complexity. Numerical simulations demonstrate that the proposed algorithm outperforms the $\ell_{1}$ regularized RLS algorithm for a group sparse system identification problem and has lower implementation complexity than direct group lasso solvers.
更多查看译文
关键词
least squares approximation,system identification,prediction algorithms,real time,indexes,numerical simulation,signal processing,indexation,vectors,adaptive filters,homotopy,computational complexity
AI 理解论文
溯源树
样例
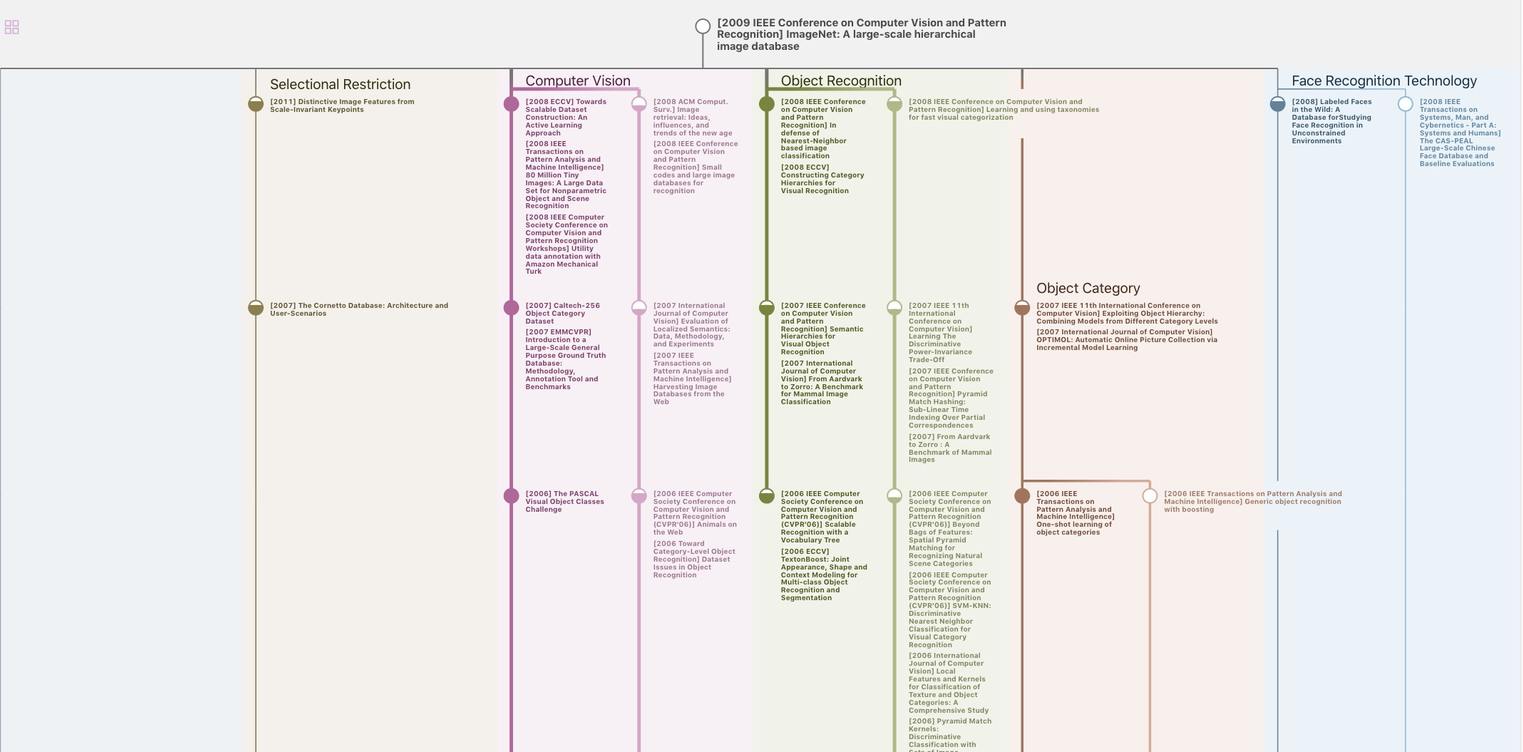
生成溯源树,研究论文发展脉络
Chat Paper
正在生成论文摘要