Multi-scale, multi-level, heterogeneous features extraction and classification of volumetric medical images
ICIP(2013)
摘要
This paper articulates a novel method for the heterogeneous feature extraction and classification directly on volumetric images, which covers multi-scale point feature, multi-scale surface feature, multi-level curve feature, and blob feature. To tackle the challenge of complex volumetric inner structure and diverse feature forms, our technical solution hinges upon the integrated approach of locally-defined diffusion tensor (DT), DT-based anisotropic convolution kernel (DACK), DACK-based multi-scale analysis, and DT-governed curve feature growing. The extracted structural features can be further semantically classified. At the computational fronts, we design CUDA-based algorithm to conduct parallel computation for time consuming tasks. Various experiments and timing tests demonstrate the effectiveness, robustness, and high performance of our method.
更多查看译文
关键词
multiscale multilevel heterogeneous feature extraction,multilevel curve feature extraction,multiscale surface feature extraction,multiscale point feature extraction,multi-scale heterogeneous features,parallel architectures,convolution,dt-based anisotropic convolution kernel,cuda,cuda-based algorithm,diverse feature form,feature extraction,image classification,locally-defined diffusion tensor,volumetric image,volumetric medical images classification,dt-governed curve feature extraction,dack-based multiscale analysis,curve propagation,complex volumetric inner structure,diffusion tensor,blob feature extraction,tensors,medical image processing
AI 理解论文
溯源树
样例
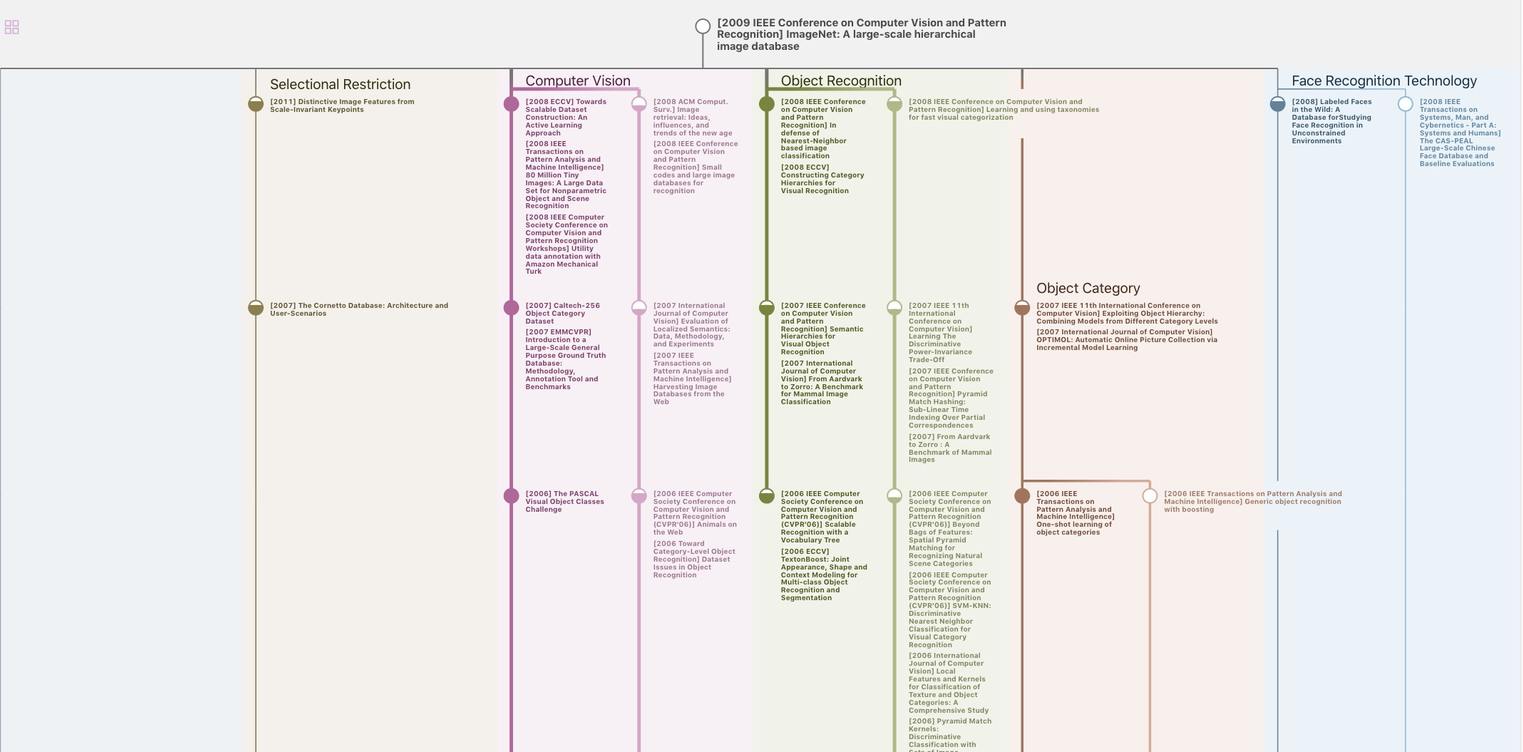
生成溯源树,研究论文发展脉络
Chat Paper
正在生成论文摘要