UnPART: PART without the 'partial' condition of it.
Information Sciences(2018)
摘要
The PART rule-induction algorithm creates rulesets by iteratively creating partial decision trees and extracting a rule from each tree. A recent study showed that growing trees further and combining it with pruning created classifiers with better discriminating capacity and less structural complexity. In this work we propose an algorithm that works in a similar way to PART, but building decision trees to their full extent, dropping the ‘partial’ condition of PART. We call this algorithm UnPART. We also propose using a different decision tree as base for PART-like algorithms. We choose CHAID* as replacement for C4.5, and propose CHAID*-based UnPART, PART and BFPART algorithms. We compare the six PART-like algorithms and their base decision trees from different points of view: discriminating capacity, structural complexity and computational cost. Results show that C4.5-based UnPART creates the best classifying models whereas CHAID*-based UnPART creates the simplest classifiers. We then compare these eight algorithms to a wider set of 21 comprehensible decision tree and rule-induction algorithms over 96 datasets from the perspective of discriminating capacity. Results show that C4.5-based UnPART has the best discriminating capacity among the compared algorithms.
更多查看译文
关键词
Comprehensible classifiers,Interpretable models,Rule sets,Full decision trees,Partial decision trees,Machine learning
AI 理解论文
溯源树
样例
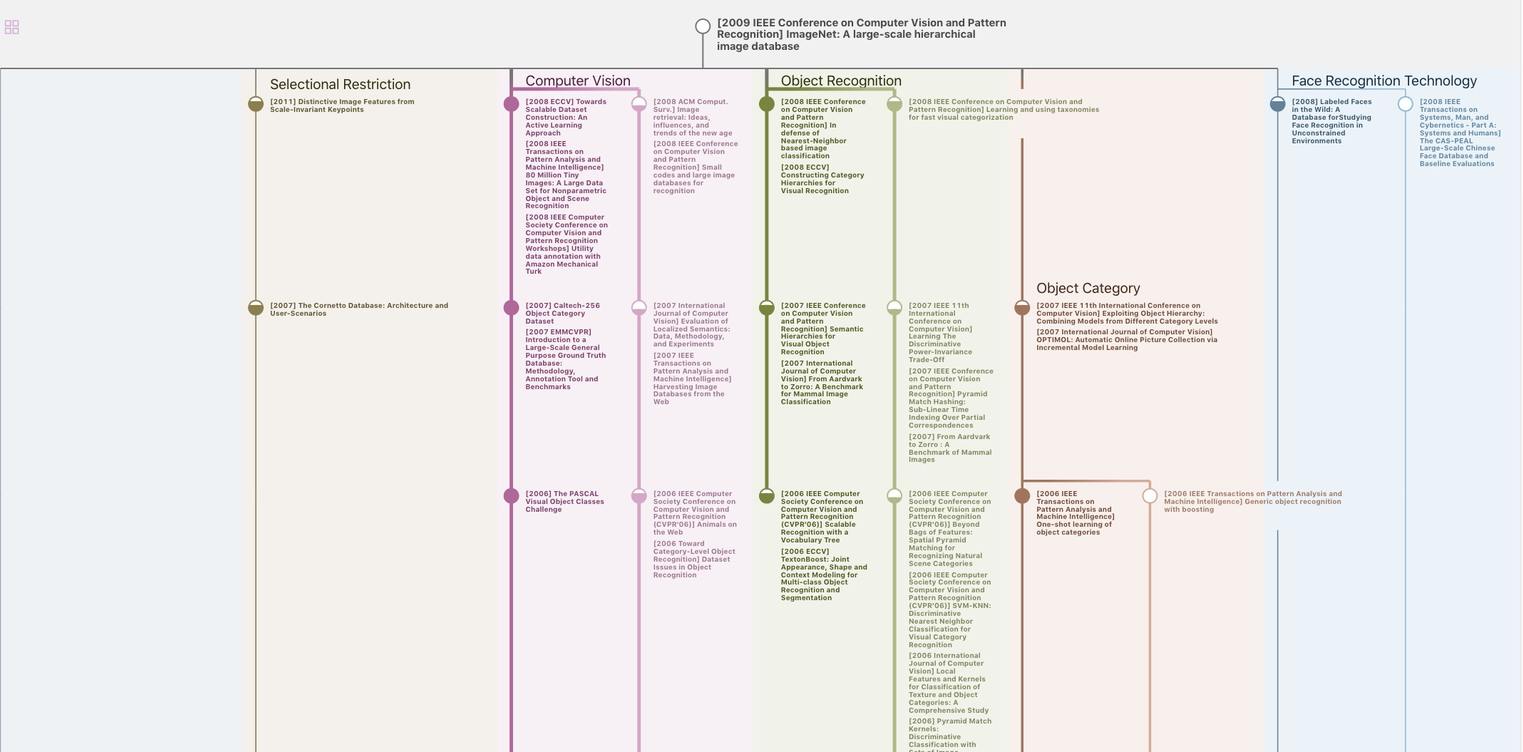
生成溯源树,研究论文发展脉络
Chat Paper
正在生成论文摘要