Efficient Mining Of Top Correlated Patterns Based On Null-Invariant Measures
ECML PKDD'11: Proceedings of the 2011 European conference on Machine learning and knowledge discovery in databases - Volume Part II(2011)
摘要
Mining strong correlations from transactional databases often leads to more meaningful results than mining association rules. In such mining, null (transaction)-invariance is an important property of the correlation measures. Unfortunately, some useful null-invariant measures such as Kulczynski and Cosine, which can discover correlations even for the very unbalanced cases, lack the (anti)-monotonicity property. Thus, they could only be applied to frequent itemsets as the post-evaluation step. For large datasets and for low supports, this approach is computationally prohibitive. This paper presents new properties for all known null-invariant measures. Based on these properties, we develop efficient pruning techniques and design the Apriori-like algorithm NICOMINER for mining strongly correlated patterns directly. We develop both the threshold-bounded and the top-k variations of the algorithm, where top-k is used when the optimal correlation threshold is not known in advance and to give user control over the output size. We test NICOMINER on real-life datasets from different application domains, using Cosine as an example of the null-invariant correlation measure. We show that NICOMINER outperforms support-based approach more than an order of magnitude, and that it is very useful for discovering top correlations in itemsets with low support.
更多查看译文
关键词
low support,mining association rule,Apriori-like algorithm NICOMINER,correlation measure,known null-invariant measure,null-invariant correlation measure,optimal correlation threshold,strong correlation,top correlation,useful null-invariant,efficient mining,top correlated pattern
AI 理解论文
溯源树
样例
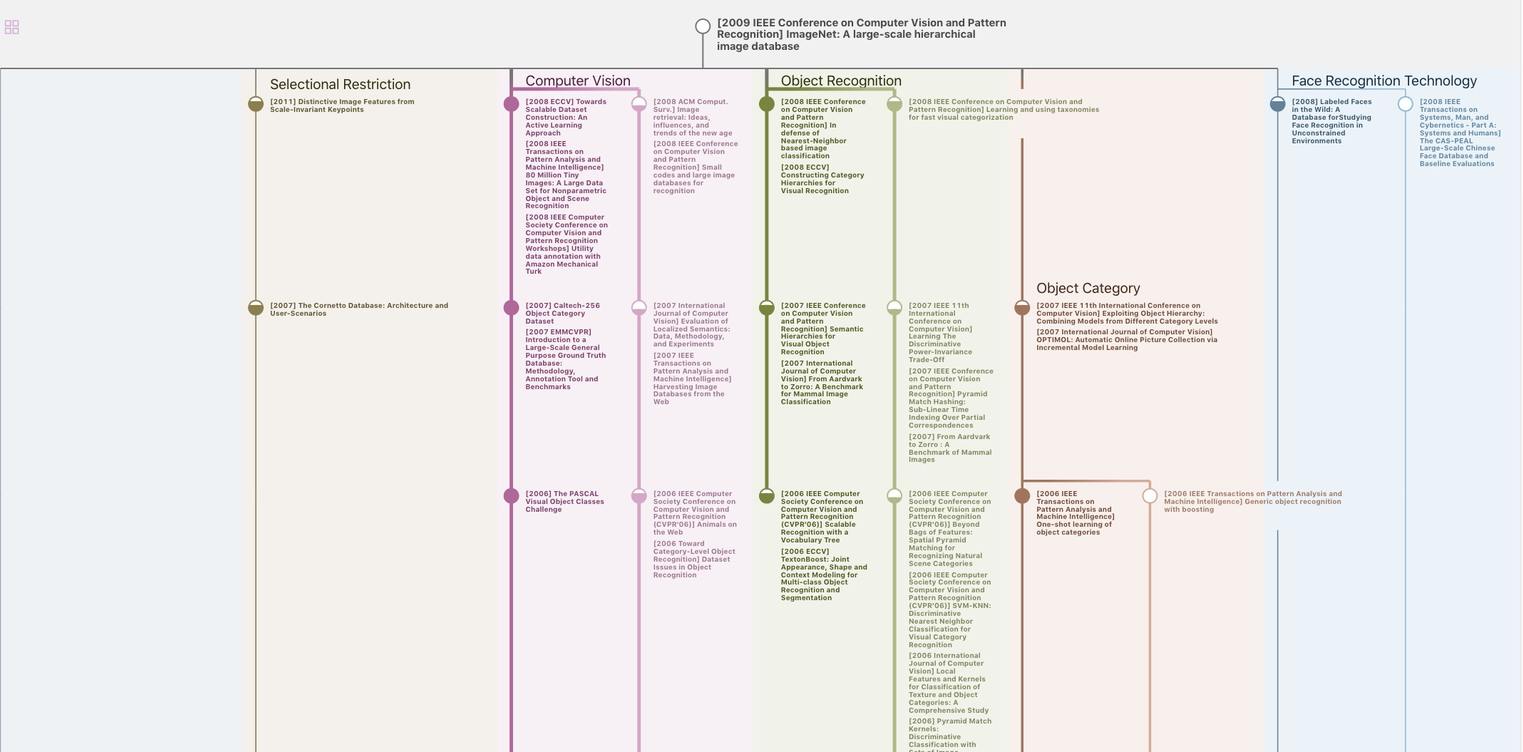
生成溯源树,研究论文发展脉络
Chat Paper
正在生成论文摘要