Motion fields to predict play evolution in dynamic sport scenes
CVPR(2010)
摘要
Videos of multi-player team sports provide a challenging domain for dynamic scene analysis. Player actions and interactions are complex as they are driven by many factors, such as the short-term goals of the individual player, the overall team strategy, the rules of the sport, and the current context of the game. We show that constrained multi-agent events can be analyzed and even predicted from video. Such analysis requires estimating the global movements of all players in the scene at any time, and is needed for modeling and predicting how the multi-agent play evolves over time on the field. To this end, we propose a novel approach to detect the locations of where the play evolution will proceed, e.g. where interesting events will occur, by tracking player positions and movements over time. We start by extracting the ground level sparse movement of players in each time-step, and then generate a dense motion field. Using this field we detect locations where the motion converges, implying positions towards which the play is evolving. We evaluate our approach by analyzing videos of a variety of complex soccer plays.
更多查看译文
关键词
player movement,video signal processing,dense motion field,play evolution,player position,constrained multiagent events,dynamic scene analysis,multiplayer team sports,multiagent play,multi-agent systems,motion estimation,global movement estimation,player action,sport,video,ground level sparse movement,location detection,player interaction,soccer,dynamic sport scene,motion fields,information analysis,convergence,games,layout,color,tracking,predictive models,multi agent systems
AI 理解论文
溯源树
样例
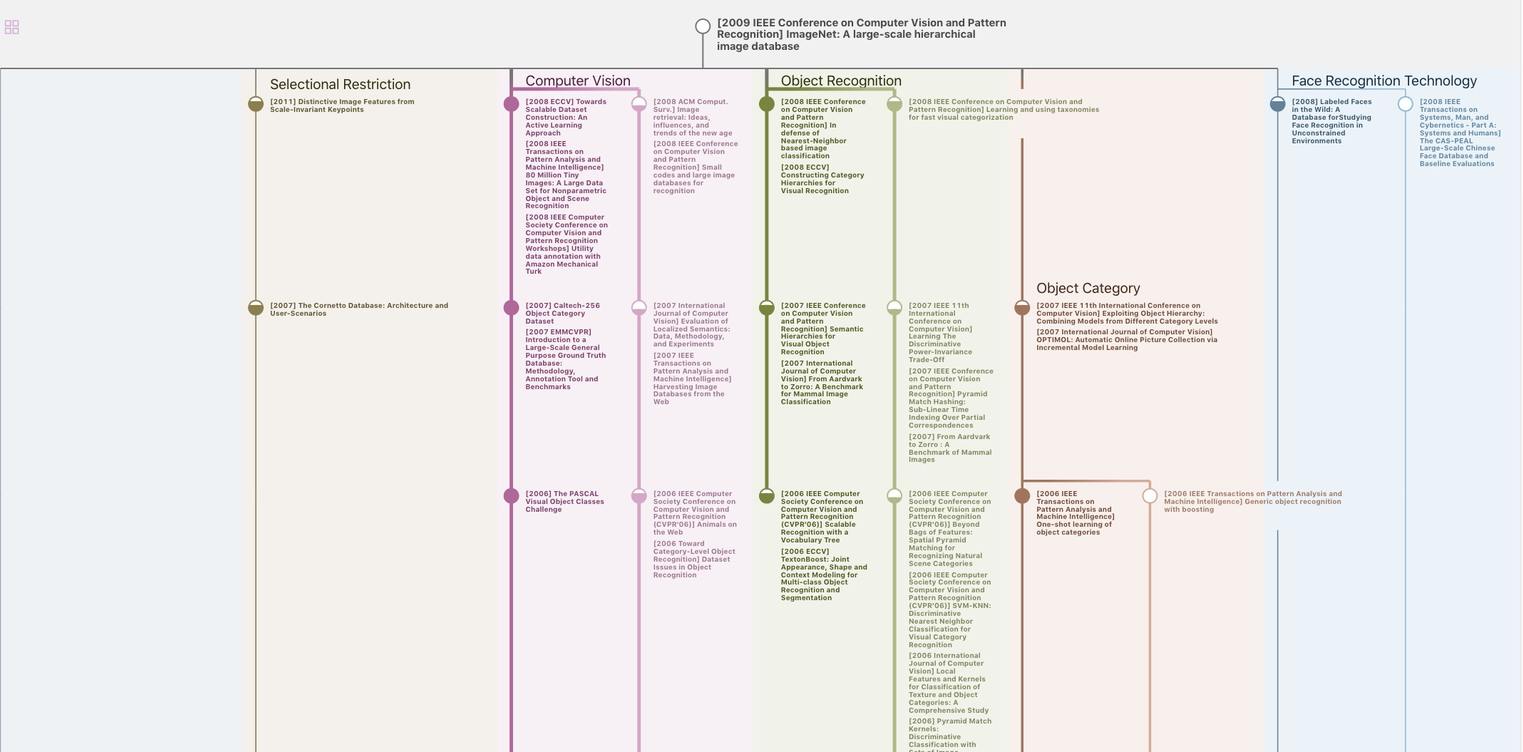
生成溯源树,研究论文发展脉络
Chat Paper
正在生成论文摘要