Unsupervised Discovery of Abnormal Activity Occurrences in Multi-dimensional Time Series, with Applications in Wearable Systems
SDM(2010)
摘要
We present a method for unsupervised discovery of abnormal occurrences of activities in multi-dimensional time series data. Unsupervised activity discovery approaches dier from traditional supervised methods in that there is no requirement for manually labeled training datasets. In addition, they minimize the need for eld experts' knowledge during the setup phases, which makes the deployment phase faster and simpler. We focus our attention on wearable computing systems and their applications in human activity monitoring for health care and medicine. The developed method constructs activity models in multi-dimensional time series based on the frequency and coincidence of activity perceptual primitives in single-dimensional time series data. We study the frequent variations exposed in human activity time series data and leverage physical attributes of the data to classify the activity primitives. A graph clustering approach is used to construct the frequent activity structures. Such structures are used to locate normal and abnormal occurrences of activities in time series. A method is presented to distinguish the abnormal activity occurrences from the normal occurrences. Two state-of-the- art wearable embedded systems (Smartcane and Smartshoe) are used to perform empirical evaluation of the developed methods.
更多查看译文
关键词
time series data,wearable computer,graph clustering,time series,health care,embedded system
AI 理解论文
溯源树
样例
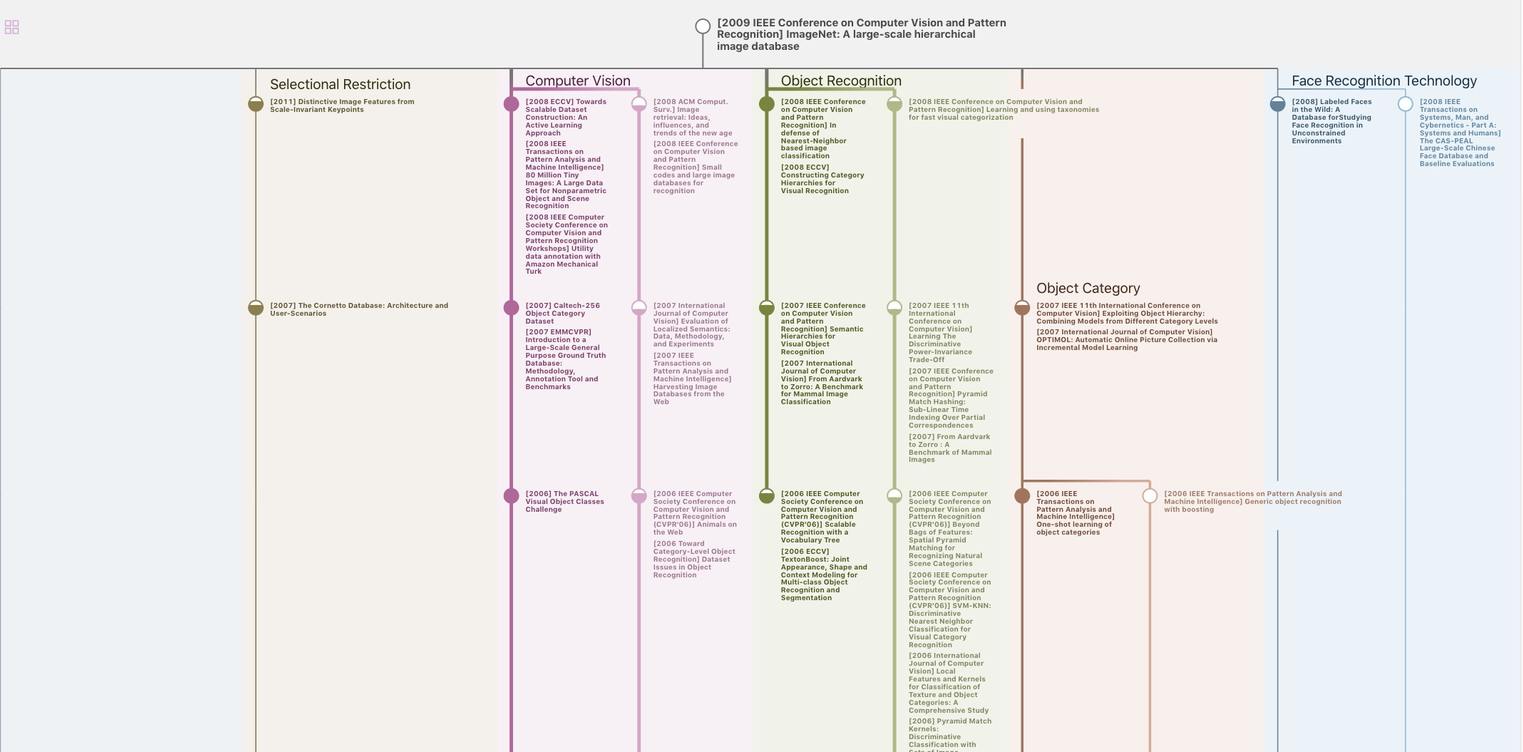
生成溯源树,研究论文发展脉络
Chat Paper
正在生成论文摘要