Learning $\ell_1$-based analysis and synthesis sparsity priors using bi-level optimization.
neural information processing systems(2014)
摘要
We consider the analysis operator and synthesis dictionary learning problems based on the the $\ell_1$ regularized sparse representation model. We reveal the internal relations between the $\ell_1$-based analysis model and synthesis model. We then introduce an approach to learn both analysis operator and synthesis dictionary simultaneously by using a unified framework of bi-level optimization. Our aim is to learn a meaningful operator (dictionary) such that the minimum energy solution of the analysis (synthesis)-prior based model is as close as possible to the ground-truth. We solve the bi-level optimization problem using the implicit differentiation technique. Moreover, we demonstrate the effectiveness of our leaning approach by applying the learned analysis operator (dictionary) to the image denoising task and comparing its performance with state-of-the-art methods. Under this unified framework, we can compare the performance of the two types of priors.
更多查看译文
AI 理解论文
溯源树
样例
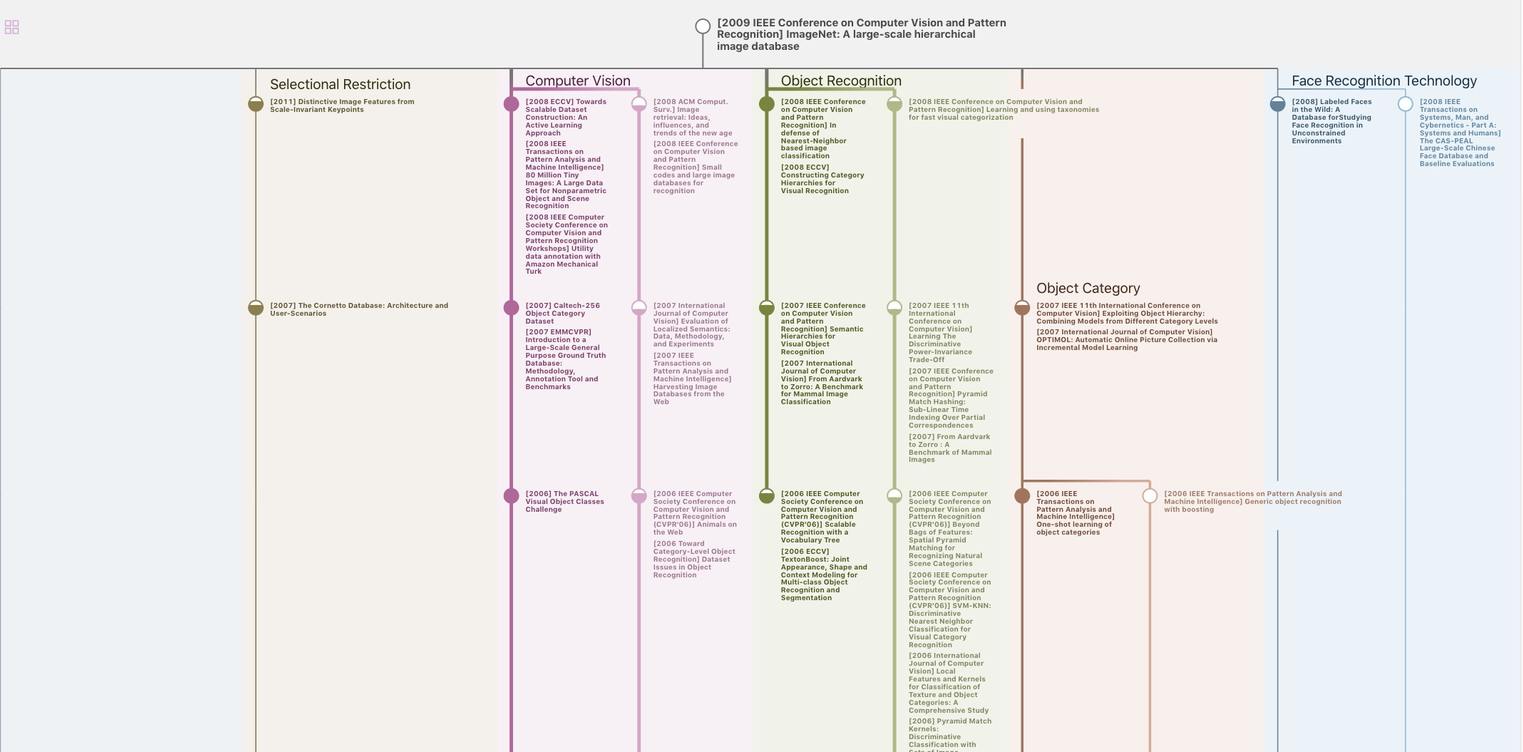
生成溯源树,研究论文发展脉络
Chat Paper
正在生成论文摘要