Visual words assignment on a graph via minimal mutual information loss.
BMVC(2012)
摘要
Visual codewords assignment plays an important role in many Bag of Features (BoF) models for image understanding and visual recognition. It allocates image descriptors to the most similar codewords in the pre-configured visual dictionary to generate descriptive histogram for the consequent categorization. Nevertheless, existing assignment approaches, e.g. nearest neighbors strategy and Gaussian similarity, suffer from two problems: 1) too strong Euclidean assumption and 2) neglecting the label information of the local features. Accordingly, in this paper, we propose an assignment method to simultaneously consider the above two issues in a unified model via graph learning and information theoretic criterions. For learning, the proposed model can be efficiently solved in a closed-form with the reasonable graph topology invariant approximation. Moreover, the learned projections enable us to extend the assignment ability to the out-of-sample visual features beyond the initial training graph. Experiments on our own manifold dataset and two benchmarks verify the effectiveness of the proposed graph assignment method.
更多查看译文
AI 理解论文
溯源树
样例
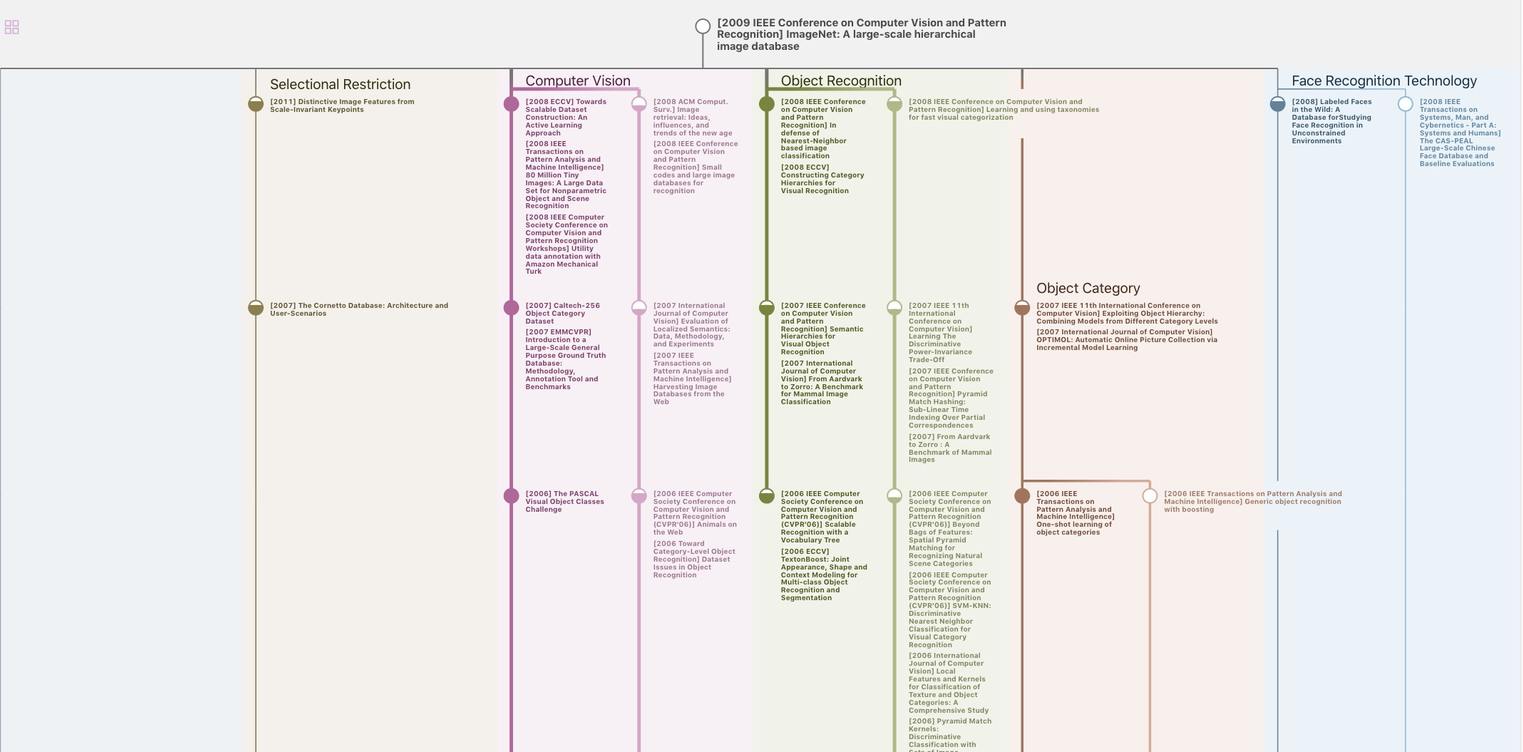
生成溯源树,研究论文发展脉络
Chat Paper
正在生成论文摘要