A comparison of supervised classification methods for auditory brainstem response determination.
Studies in Health Technology and Informatics(2007)
摘要
The ABR is commonly used in the Audiology clinic to determine and quanti, hearing loss. Its interpretation is subjective, dependent upon the expertise and experience of the clinical scientist. It? this study we investigated the role of machine learning for pattern classification in this domain. We extracted features from the ABRs of 85 test subjects (550 waveforms) and compared four complimentary supervised classification methods: Naive Bayes, Support Vector Machine, Multi-Layer Perceptron and KStar The ABR dataset comprised both high level and near threshold recordings, labeled as 'response' or 'no response' by the human expert. Features were extracted from single averaged recordings to make the classification process straightforward. A best classification accuracy of 83.4% was obtained using Naive Bayes and five relevant features extracted from time and wavelet domains. Naive Bayes also achieved the highest specificity (863%). The highest sensitivity (93.1%) was obtained with Support Vector Machine-based classification models. In terms of the overall classification accuracy, four classifiers have shown the consistent, relatively high performance, indicating the relevance of selected features and the feasibility of using machine learning and statistical classification models in the analysis of ABR.
更多查看译文
关键词
Auditory Brainstem Response,wavelet decomposition,feature extraction,classification,decision support
AI 理解论文
溯源树
样例
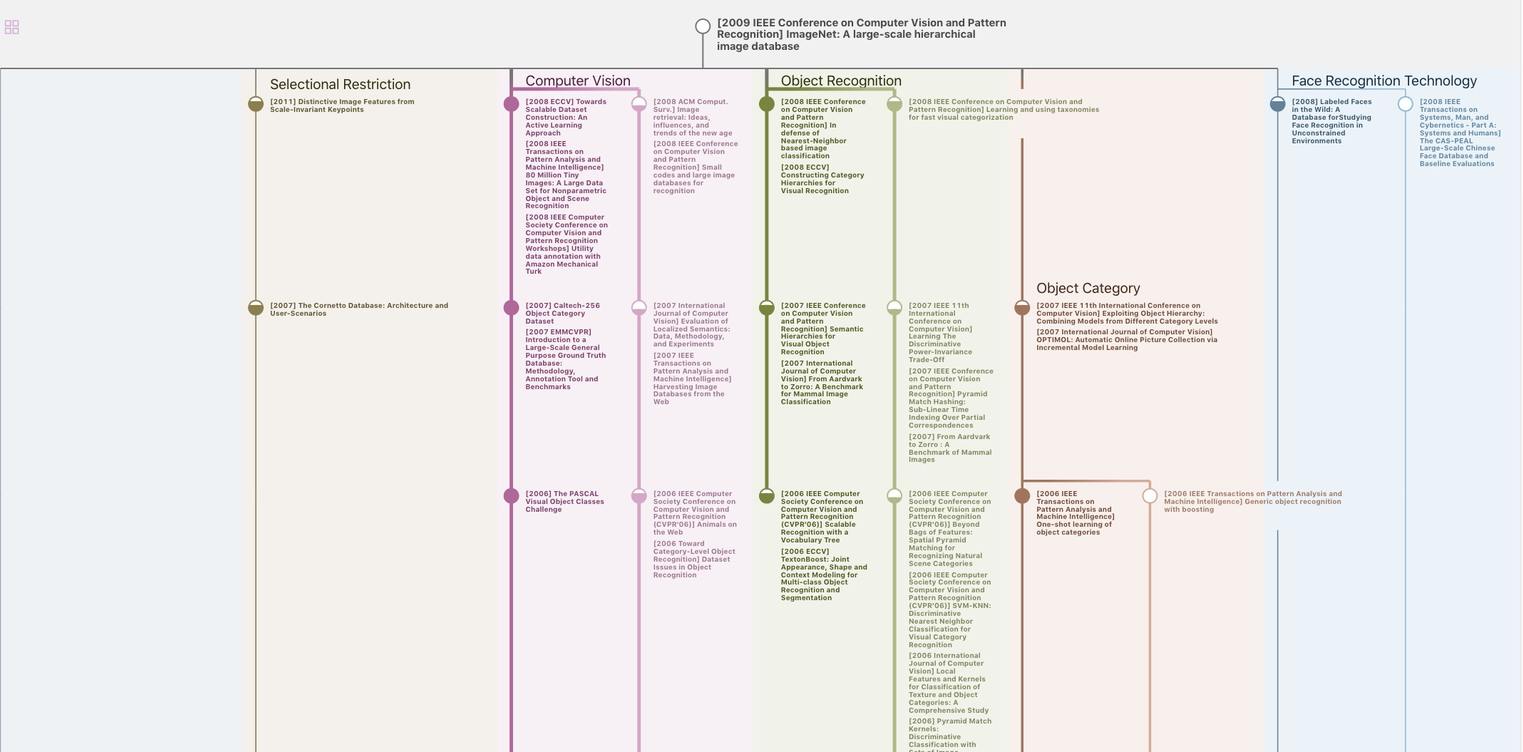
生成溯源树,研究论文发展脉络
Chat Paper
正在生成论文摘要