Design of Bismuth-Doped Fiber Amplifier Based on Neural Network and Multi-Objective Optimization Algorithm
ACTA OPTICA SINICA(2024)
摘要
Objective Multi-band transmission is considered an effective solution to address the increasing capacity constraints in fiber optic communication systems. However, due to the lack of mature optical amplifiers, the large-scale deployment of dense wavelength division multiplexing (DWDM) technology for long-distance transmission in bands such as O, E, and S has not yet been achieved. In recent years, researchers have discovered that different dopants in bismuth-doped silica fibers exhibit broad fluorescence characteristics in the near-infrared region. This finding brings hope for addressing the aforementioned challenges. In traditional approaches, the performance analysis of amplifiers often requires solving a set of coupled differential equations using methods such as the Runge-Kutta algorithm combined with the Shooting method or Relaxation method. When incorporating global optimization algorithms, it becomes necessary to solve thousands of related equations, resulting in a complex and time-consuming process. Previous research methods have mainly focused on the optimization design of Raman fiber amplifiers or hybrid optical amplifiers, with fewer studies specifically targeting the structural optimization design of doped fiber amplifiers, particularly bismuth-doped fiber amplifier (BDFA). Moreover, most of these studies have employed single-objective optimization algorithms, resulting in obtaining only one optimal solution at a time. In general, there is a trade-off relationship between the gain and noise performance of amplifiers. Increasing the gain often leads to the deterioration of the noise performance, and vice versa. As a result, there is no unique optimal solution. Therefore, it is necessary to design a method that can accurately model the amplifier and efficiently optimize multiple performance metrics simultaneously. Methods The backpropagation neural network (BPNN) is a type of multilayer feedforward neural network consisting of input layer, hidden layers, and output layer. The input layer contains six neurons corresponding to the input signal wavelength and five structural parameters of the amplifier. The output layer contains two neurons corresponding to the Gain and noise figure (NF) of the respective wavelength signals. The main characteristic of BPNN is the forward propagation of signals and the backward propagation of errors. It belongs to the supervised learning methods. For multi-objective problems, the objective values are typically mutually constrained, and there is no unique optimal solution. Using multi-objective optimization algorithms can provide a set of independent optimal solutions, allowing engineering designers to choose based on their actual needs. NSGA-II is a multi-objective optimization algorithm that improves upon the non-dominated sorting genetic algorithm (NSGA). By introducing fast non-dominated sorting, elite preservation strategy, and crowding distance operator, NSGA-II reduces computational complexity, improves optimization efficiency, and ensures the diversity of individuals in the population. Results and Discussions Simulation experiments were conducted using a theoretical model of a two-stage BDFA to obtain a sample set. The BPNN model was trained and tested with different sample sizes, with a training-to-testing set ratio of 9:1. It was observed that as the sample set size increased, the overall trend of RMSE decreased while the R-2 value increased (Fig. 4). When the sample size reached 3000, the BPNN model achieved an RMSE of 0.191 for Gain and 0.084 for NF in the testing phase, with R-2 values of 0. 999 and 0. 998, respectively. The established BPNN model exhibits high prediction accuracy and can effectively capture the nonlinear relationship between the structural parameters and performance of the two-stage BDFA. Based on the established BPNN model, the objective function is evaluated, and after 100 iterations, a Pareto optimal solution set containing 500 solutions is obtained (Fig. 6). Furthermore, a comparison is made between the performance of using SVM and BPNN for predicting Gain and NF. The results show that the BPNN model has smaller prediction errors and higher accuracy in predicting Gain and NF. Additionally, the time required for optimization design using BPNN-NSGA-II is five orders of magnitude lower than using Relaxation method combined with NSGA-II, taking less than 80 seconds to complete the design. Compared to SVM-NSGA-II, the time is reduced by one order of magnitude (Fig. 9). Conclusions This paper proposes a multi-objective optimization method that combines BPNN and NSGA-II algorithms for accurate modeling and efficient design optimization of two-stage BDFA. By establishing a BPNN model to map the nonlinear relationship between structural parameters and performance, it avoids the need for repetitive solving of coupled differential equations. After training and testing, the BPNN model exhibits low RMSE and high R-2 values. Using this BPNN model in conjunction with the NSGA-II algorithm, a Pareto optimal solution set containing 500 solutions is obtained. The paper also provides the Gain and NF spectra for five different amplifier configurations. Compared to other methods, the proposed approach significantly reduces the optimization design time, improves optimization efficiency, and enables the simultaneous attainment of multiple optimal solutions, providing decision-makers with more choices.
更多查看译文
关键词
fiber optic communication,bismuth-doped fiber amplifier,backpropagation neural network,multi-objective optimization,fast and elitist nondominated sorting genetic algorithm
AI 理解论文
溯源树
样例
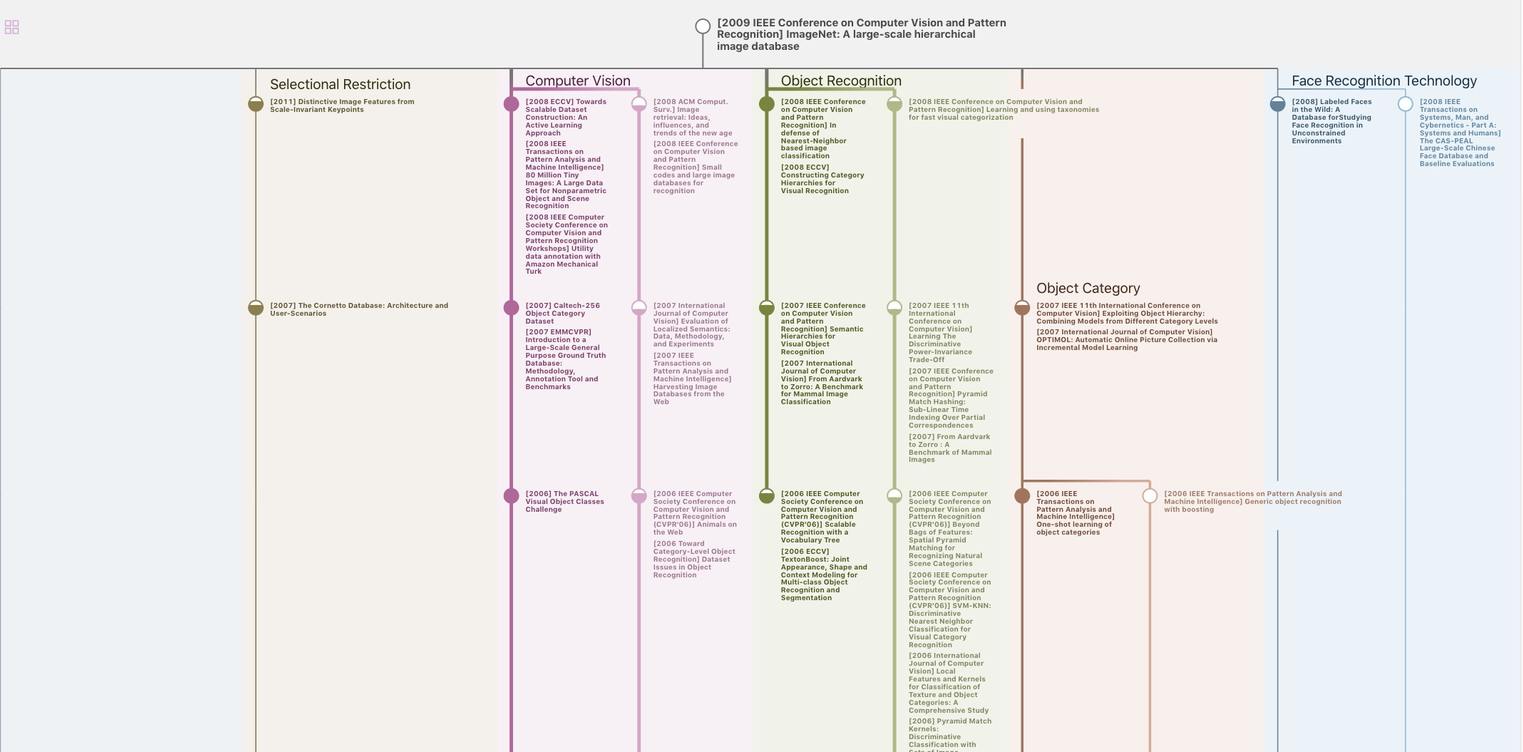
生成溯源树,研究论文发展脉络
Chat Paper
正在生成论文摘要