Tensor Decompositions For Learning Latent Variable Models
Journal of Machine Learning Research(2014)
摘要
This work considers a computationally and statistically efficient parameter estimation method for a wide class of latent variable models-including Gaussian mixture models, hidden Markov models, and latent Dirichlet allocation-which exploits a certain tensor structure in their low-order observable moments (typically, of second- and third-order). Specifically, parameter estimation is reduced to the problem of extracting a certain (orthogonal) decomposition of a symmetric tensor derived from the moments; this decomposition can be viewed as a natural generalization of the singular value decomposition for matrices. Although tensor decompositions are generally intractable to compute, the decomposition of these specially structured tensors can be efficiently obtained by a variety of approaches, including power iterations and maximization approaches (similar to the case of matrices). A detailed analysis of a robust tensor power method is provided, establishing an analogue of Wedin's perturbation theorem for the singular vectors of matrices. This implies a robust and computationally tractable estimation approach for several popular latent variable models.
更多查看译文
关键词
latent variable models,tensor decompositions,mixture models,topic models,method of moments,power method
AI 理解论文
溯源树
样例
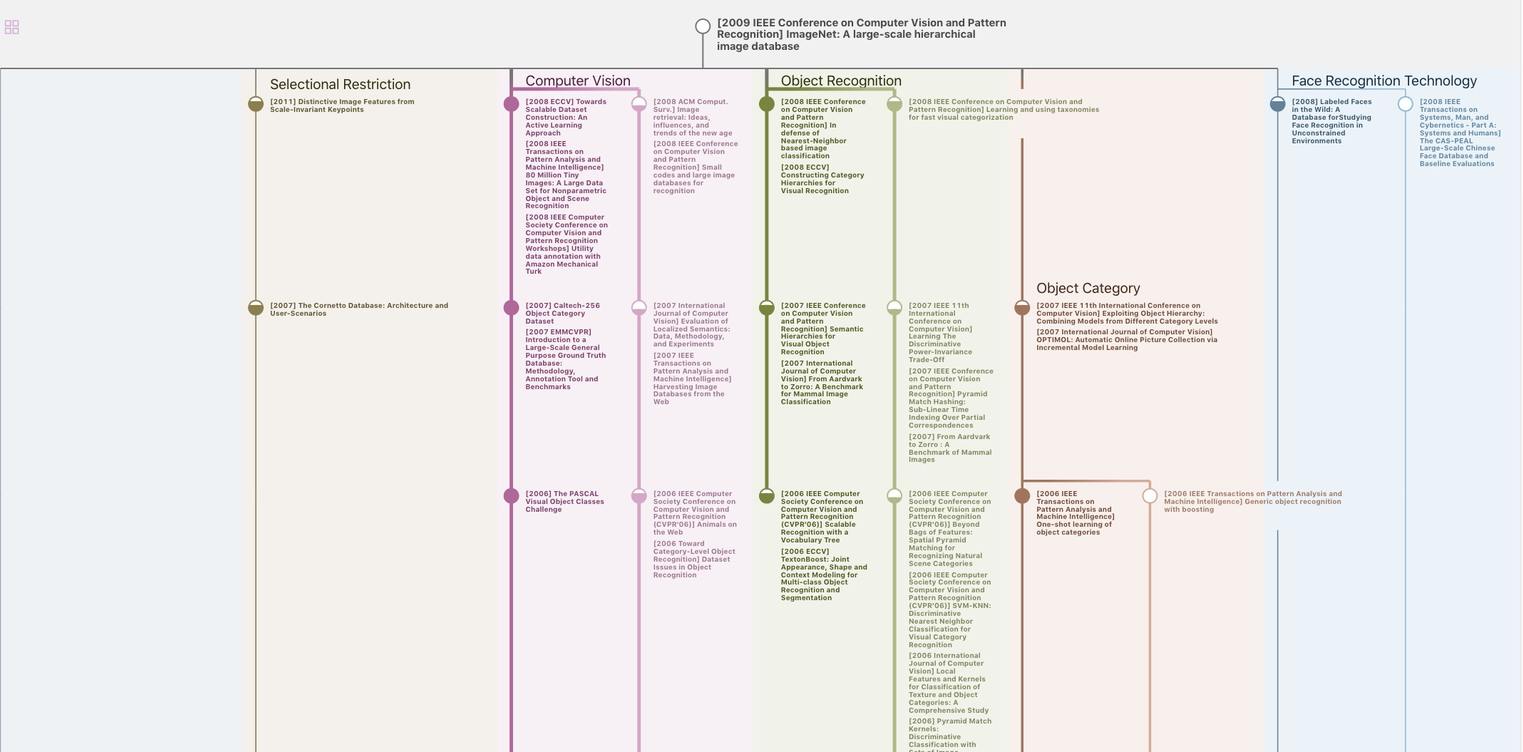
生成溯源树,研究论文发展脉络
Chat Paper
正在生成论文摘要