Collecting Image Annotations Using Amazon's Mechanical Turk.
CSLDAMT '10: Proceedings of the NAACL HLT 2010 Workshop on Creating Speech and Language Data with Amazon's Mechanical Turk(2010)
摘要
Crowd-sourcing approaches such as Amazon's Mechanical Turk (MTurk) make it possible to annotate or collect large amounts of linguistic data at a relatively low cost and high speed. However, MTurk offers only limited control over who is allowed to particpate in a particular task. This is particularly problematic for tasks requiring free-form text entry. Unlike multiple-choice tasks there is no correct answer, and therefore control items for which the correct answer is known cannot be used. Furthermore, MTurk has no effective built-in mechanism to guarantee workers are proficient English writers. We describe our experience in creating corpora of images annotated with multiple one-sentence descriptions on MTurk and explore the effectiveness of different quality control strategies for collecting linguistic data using Mechanical MTurk. We find that the use of a qualification test provides the highest improvement of quality, whereas refining the annotations through follow-up tasks works rather poorly. Using our best setup, we construct two image corpora, totaling more than 40,000 descriptive captions for 9000 images.
更多查看译文
关键词
correct answer,linguistic data,Mechanical MTurk,different quality control strategy,limited control,Mechanical Turk,best setup,descriptive caption,effective built-in mechanism,follow-up task,Collecting image
AI 理解论文
溯源树
样例
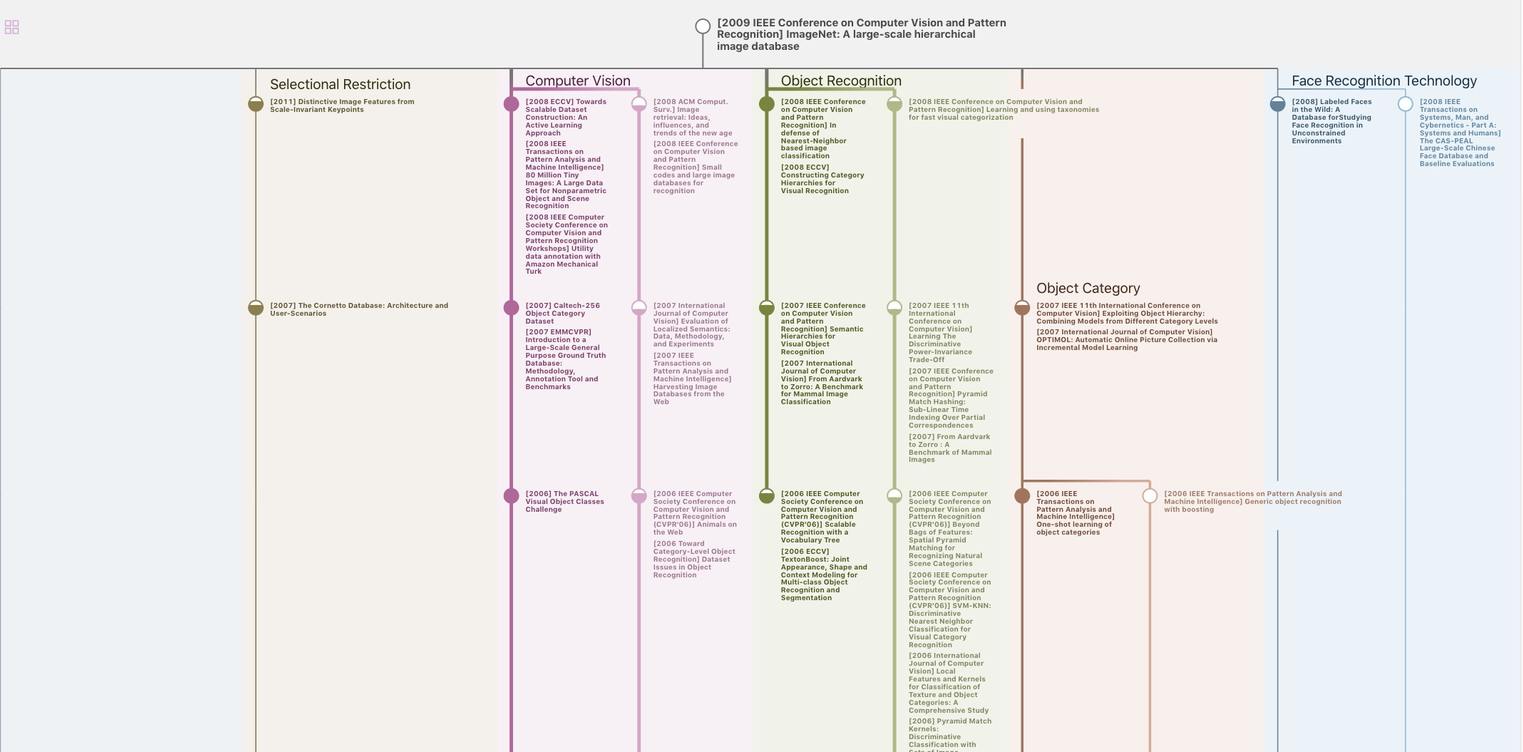
生成溯源树,研究论文发展脉络
Chat Paper
正在生成论文摘要